Get to know some of the Helmholtz scientists who would like to welcome a visiting researcher to their group for a short-term research stay. Find out what projects they are currently working on, what your contribution can look like and what you can learn from their specific research approaches.
Helmholtz-wide cooperations
If you are looking for a suitable host supervisor, please note: You can also contact other scientists (usually PIs or Head of Divisions) at the 18 Helmholtz centers for this purpose and arrange with them to participate in the Helmholtz Visiting Researcher Grant or the HIDA Trainee Network. The list below is not exhaustive.
For an overview of the Helmholtz centers and specific research areas, please click here:
Helmholtz Hosts
Get to know your potential hosts at the Helmholtz Centers and learn more about their respective data science research by clicking on the cards.
Please note: You have to contact your potential host supervisor by email in order to propose and discuss a possible research project before submitting your application.
If you do have questions, please email: hida@helmholtz.de
Would you like to become a Helmholtz host yourself and are looking for support for your research project? Then please contact us at the above e-mail address as well.
Helmholtz Center for Environmental Research - UFZ
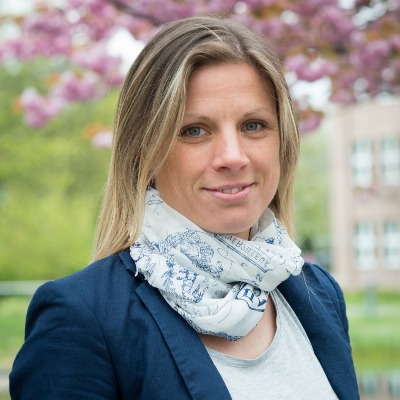
Jana Schor
Jana Schor - UFZ
Helmholtz Centre for Environmental Research (UFZ) - Data Science group at the department of bioinformatics
Short summary of your group's research: The new research group Data Science under the supervision of Dr. Jana Schor focuses on Data Science methods, like Machine Learning and Data Integration, to boost the extraction of knowledge from human and environmental health Big Data sets. We apply and develop respective computational methods on public and in-house data sets, mainly in the field of environmental and health research, to generate novel hypotheses, and make predictions on a large scale. To increase the credibility of our AI approaches we introduce explainability and methods for the quantification of uncertainty into our applications. We further attach great importance on the principles of reproducible research.
What infrastructure, programs and tools are used in your group? High performance computing infrastructure at the UFZ and storage as well as data integration capabilities of the European Open Science Cloud; Software is developed in R, and Python, and is provided in Singularity containers and/or in public gitlab repositories at the HZDR; for training our deep learning models or other computationally expensive calculations we use the GPUs that are integrated into our servers and the HPC system.
What could a participant of the HIDA Trainee Network learn in your group? How could he or she support you in your group? A participant of the HIDA Trainee Network will learn to organize his/her data analysis or software development projects according to clear data management plans and with SOPs that allow shared and joint code development, respective documentation, and the reproducibility of research results. We offer to share our expertise in the application of machine learning approaches and respective data pre-processing strategies. Further, we are experienced in story telling with data and offer to teach respective principles to the participants. We welcome participants with a background in machine learning who are interested in the applications of their methods to our research questions, e.g. in the field of predictive toxicology. We also invite researchers with a chemistry, (eco-)toxicological or biological background who might inspire us with interesting research questions and who are willing to learn helpful data science strategies.
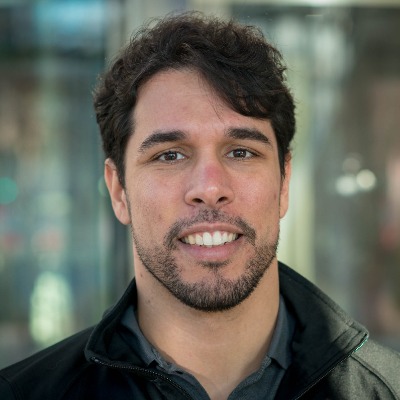
Ulisses Nunes da Rocha
Ulisses Nunes da Rocha - UFZ
Helmholtz Centre for Environmental Research (UFZ) - Microbial Data Science at the department of Environmental Microbiology
Short summary of your group's research: Our group strives to assess environmental health of terrestrials and man made environments by predicting how resilient/stable microbial communities are to disturbances. A special emphasis is put on the development of concepts and theories to scale microbial interactions to the real diversity found in nature. The key research topics of the Microbial Data Science group are based on genetic potential of microbial communities, multi-omics integration and predictive biology. Currently these topics cover:
- Use of (in silico) mock microbial communities to test microbial ecology theories;
- From microbial 'Big Data' to novel ecological concepts and theories;
- Predictive analytics in microbiology, microbial ecology and environmental microbiology.
What infrastructure, programs and tools are used in your group? We use the High-Performance Computing (HPC) Cluster EVE as our main computational resource. We develop and use tools to analyze, resolve and interpret multi-omics data, specially metagenomes. Eg.: tools for the recovery of multi-domain genomes (prokaryotes, viruses and eukaryotes) from metagenomic data. As programming languages, we mostly use Python, R, Bash and Perl to handle and process microbial big data.
What could a participant of the HIDA Trainee Network learn in your group? How could he or she support you in your group? A participant from the HIDA Trainee Network will learn how to download, process and interpret metagenomic data using both publicly available datasets as well as in-house generated data. Further, the participant will work with hands on experimental design and predictive analytics using omics data. We welcome participants from a broad range of fields (e.g. microbiology, ecology, computer/data science) that are eager to learn and/or expand their knowledge in computational biology/data science.
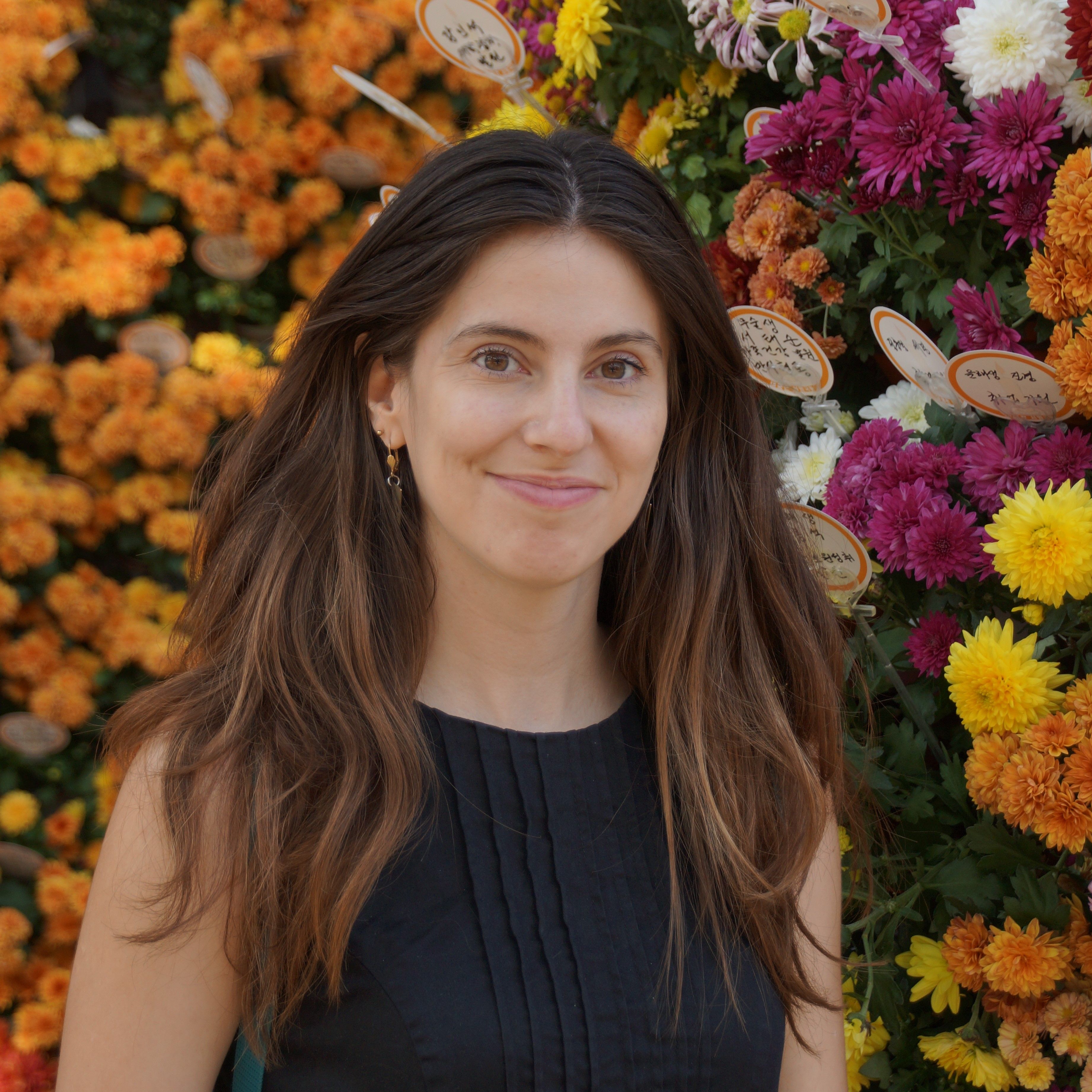
Mariana Madruga de Brito
Mariana Madruga de Brito - UFZ
Helmholtz Centre for Environmental Research (UFZ) - Environmental Risks and Extreme events group
Short summary of your group's research: The research stay will be conducted at the working group Environmental Risks and Extreme events led by Prof. Christian Kuhlicke (https://www.ufz.de/index.php?en = 40374). Dr. Mariana Madruga de Brito will supervise the research. The group has expertise in computational social sciences and digital humanities. We use text as data for natural hazards adaptation, resilience and vulnerability research. We are particularly interested in understanding how these processes affect society and who are prominent stakeholders. Topics of interest include natural language processing (NLP), topic modelling, and unsupervised and supervised learning algorithms. Our text corpora include newspaper articles, tweets, and large sets of peer-reviewed articles.
What infrastructure, programs and tools are used in your group? The participant will have access to high-performance computing infrastructure at the UFZ. As programming languages, we mainly use R and Python.
What could a participant of the HIDA Trainee Network learn in your group? How could he or she support you in your group? As an interdisciplinary research group, we offer many possibilities for a participant of the HIDA Trainee Network and many different applied projects to support. Weekly meetings will be conducted to help monitor progress. The participant can either apply their NLP knowledge to our existing data or shape a new project together with a focus on natural hazards and climate change adaptation research. The final goal is to have a prominent joint publication.
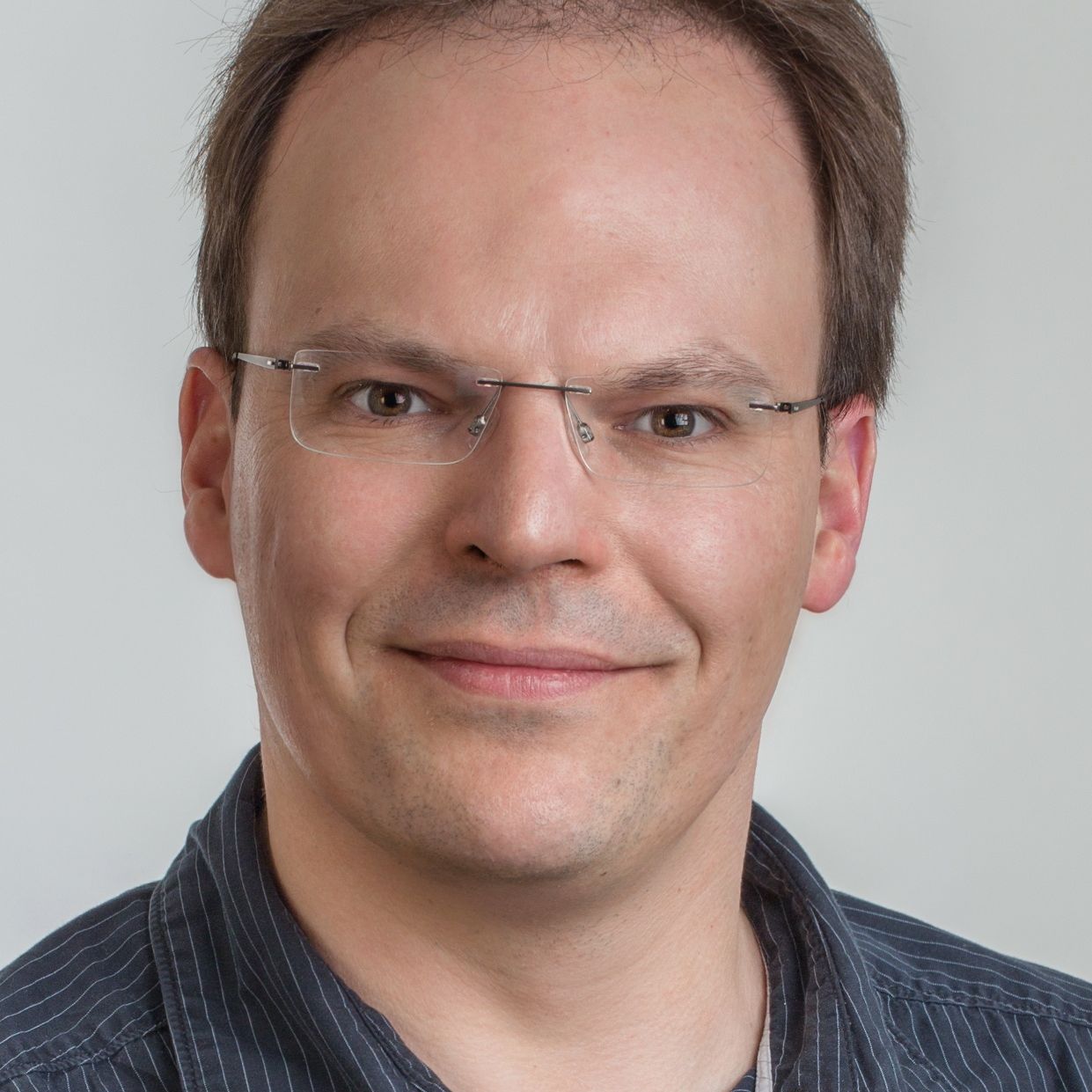
Patrick Fink
Patrick Fink - UFZ
Helmholtz Centre for Environmental Research (UFZ) - Workgroup Experimental Aquatic Ecology: Department River Ecology, and Department Aquatic Ecosystem Analysis and Management
Short summary of your group's research: We investigate the ecology of aquatic organisms in response to environmental change and heterogeneity. In particular, we are interested in adaptations of these organisms on the levels of behaviour, physiology, phenotypic plasticity and evolution. We use a broad spectrum of methods ranging from molecular biology, analytical chemistry, stable isotope analysis, physiology and behaviour. In particular, we use the UFZ’s MOBile aquatic mesoCOSm facility (MOBICOS) to perform controlled, multifactorial experiments on the effects of natural and anthropogenic stressors on stream ecosystems. Thereby, we aim to relate the organisms and their adaptive response to properties of the whole ecosystem such as biodiversity, food web structure, trophic transfer or matter and energy to gain a better understanding of ecosystems' structure and functions.
What infrastructure, programs and tools are used in your group? Mesocosm infrastructure MOBICOS for the study of biodiversity, ecosystem functioning and multiple stressors on streams, rivers and lakes; water quality monitoring stations along land use gradients from pristine to heavily impacted sites; combined with laboratory studies on eco-physiology of aquatic organisms to study their adaptations to environmental change; and analytical chemistry to quantify compounds critical for the regulation of ecosystem functioning.
What could a participant of the HIDA Trainee Network learn in your group? How could he or she support you in your group? We work across multiple scales from applied field ecology, mesocosm experiments in semi-natural settings to highly controlled assays of physiological functions and analytical chemistry in the laboratory. Hence, researchers from different backgrounds can find both familiar settings to which they contribute previous experience, but also challenging new opportunities to broaden their approaches. By combining large scale field experiments with small scale ecophysiological assays and studies of molecular regulatory mechanisms, we aim for a holistic approach to study the mechanisms regulating the diversity of organisms, their physiological functions and how these contribute to the functioning of entire ecosystems. Researchers from all fields of biological, chemical and environmental sciences are welcome to contribute to these goals by contributing their diverse methods, approaches and creativity.
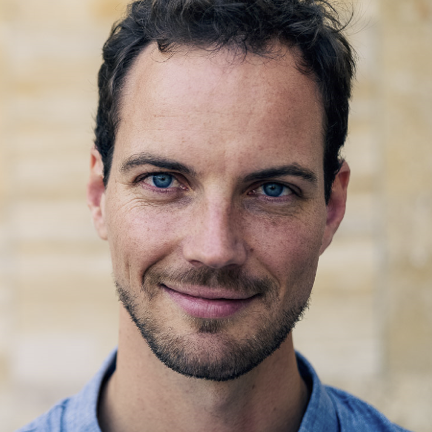
Jakob Zscheischler
Jakob Zscheischler - UFZ
Helmholtz Centre for Environmental Research (UFZ) - Computational Hydrosystems
Three-sentence summary of your group's research: We work on better understanding and modelling compound weather and climate events and their impacts. We use statistical and machine learning approaches to analysis climate and impact data to better understand compounding climate drivers of impacts, We further analysie climate model projections to estimate future climate risk.
What infrastructure, programs and tools are used in your group? We analyse large amounts of data, learn new statistical and machine learning approaches to estimate climate risks.
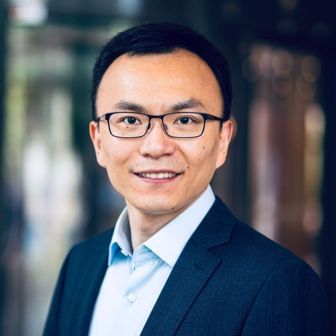
Jian Peng
Jian Peng - UFZ
Helmholtz Centre for Environmental Research (UFZ) - Department of Remote Sensing
Short summary of your group's research: The Department of Remote Sensing conducts innovative research to advance the understanding of the Earth system via various remote-sensing techniques. It has extensive research experience in quantifying land surface dynamics from multi-source Earth observations across scales. Another focus lies on the investigation of land-atmosphere interaction and climate extremes using novel remote sensing products.
What infrastructure, programs and tools are used in your group? Our team has established data lake to facilitate access to and analyse of datasets from satellite, airborne remote sensing and earth system model outputs. Modelling frameworks including radiative transfer model and land surface model have also been developed in last years. Computational infrastructures including in-house HPC and external cloud computing system can also be used by guest researchers.
What could a participant of the HIDA Trainee Network learn in your group? How could he or she support you in your group? The guest researcher can benefit from our expertise on:
1.Quantification of land surface parameters using multi-source remote sensing observations.
2. Integrate remote sensing data and land surface modelling framework to better quantify water cycle and vegetation dynamics
3. Explore land-atmosphere feedbacks and hydro-climatic extremes through novel use of remote sensing datasets (e.g., detection of extreme events, impacts of extremes on water cycle and ecosystems).
The guest researcher are encouraged to contribute to the above mentioned research topics using machine learning approaches.
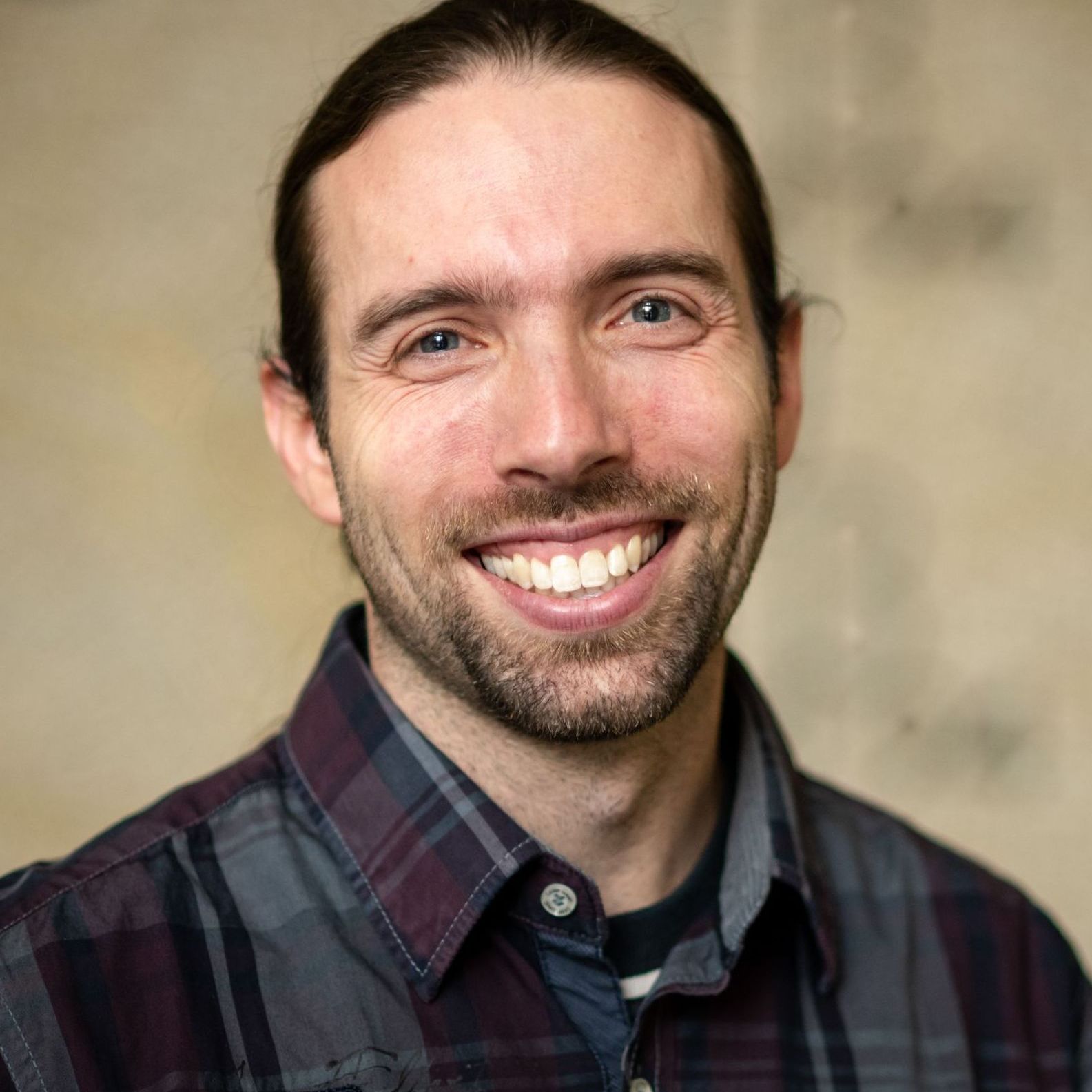
Oliver Lechtenfeld
Oliver Lechtenfeld - UFZ
Helmholtz Centre for Environmental Research (UFZ) - Department of Analytical Chemistry - Research Group BioGeoOmics
Short Summary of your Groups research: The research group BioGeoOmics integrates state-of-the art instrumental and methodological approaches to study dynamic interactions of molecules in the environment. These molecular interactions include natural processes (e.g. metabolism, organic matter diagenesis) as well as anthropogenic interferences (interaction of biological systems with chemicals). In addition to analytical method development we also develop chemoinformatics and machine learning aided data evaluation techniques.
What infrastructure, programs and tools are used in your group?At the core of the the analytical toolkit of the BioGeoOmics-group, we use ultra-high resolution mass spectrometry (FT-ICR MS) and mass spectrometric imaging (MSI). We devolp methods for non-target metabolomics and biogeochemistry using stable isotopes to study chemical and biological transformations of organic molecules, their spatial localization in tissues, and to identify metabolites and unknown chemicals and transformation products in complex mixtures.
Our group develops (automated and interactive) data processing pipelines using R, the ETL platform KNIME, and PostgreSQL DBMS. We apply multivariate statistical tools and machine learning models to extract knowledge from the zentillion data points generated from ultra-high resolution mass spectrometry and large evvironmental data sets.
What could a participant of the HIDA Trainee Network learn in your group? How could he or she support you in your group? Since our group has a focus on non-targted mass spectrometry, our guest researchers will be able to learn how to utilize and interpret non-targeted data, how to use statistical tools to evaluate the data quality, and how to derive confidence limits inorder to make sound statements about the data. Besides instrumental training on state-of-the art FT-ICR MS systems, we can provide helpful insights about data management and quality control.
Future guest researchers are welcome to provide input on fast and efficient visualization strategies for large amount of data points, on (technical legal) aspects) of software publication, and on continuous integration/deployment.
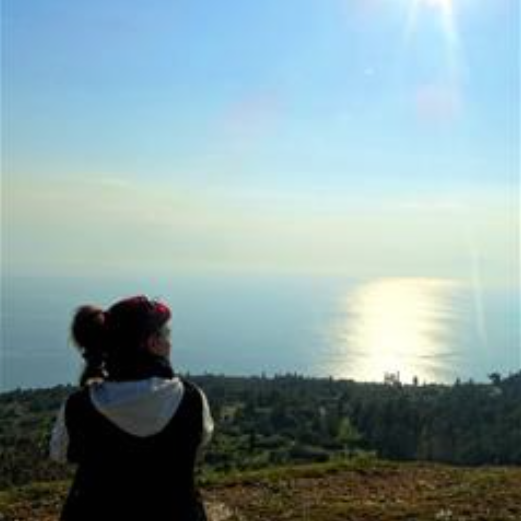
Elina Takola
Elina Takola - UFZ
Helmholtz Centre for Environmental Research (UFZ) - Computational Landscape Ecology
Three-sentence summary of your group's research: My research interests are in the field of landscape ecology, with a focus on animal ecology, biodiversity monitoring, data syntheses and meta-analyses.
I am a supporter of open science and FAIR principles. I mostly R and sometimes Python to analyze published studies and big datasets.
What could a guest researcher learn in your group? How could he or she support you in your group? Guests can participate in data synthesis projects and meta-analyses. We summarize large amounts of literature in a comprehensive way, in order to provide a complete picture of evidence to scientists, stakeholders and decision-makers.
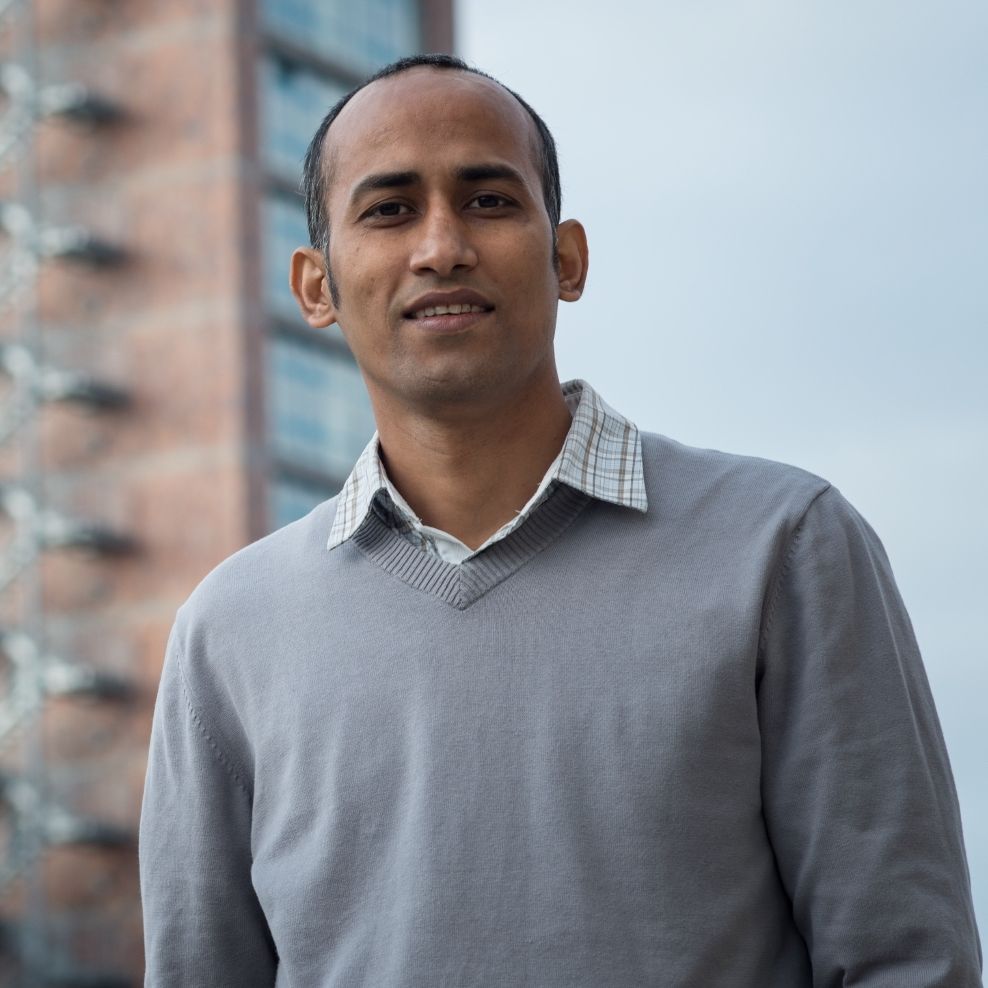
Rohini Kumar
Rohini Kumar - UFZ
Helmholtz Centre for Environmental Research (UFZ) - Department of Computational Hydrosystems
Three-sentence summary of your group's research: Our group works on improving our understanding of hydroclimatic drivers, anthropogenic pressures, and responses to the fate of water and nutrient cycles in the terrestrial system. We use data analytics and mechanistic modeling approaches to analyze and disentangle the varying role of climate, landscapes, and socioeconomic drivers shaping the hydrological response of the system. Our analysis encompasses a range of temporal scales and spatial domains varying from hourly to annual timescale in small headwater catchments to big continental-scale river basins, and our investigations cover challenging freshwater resource problems with an emphasis on unraveling hydroclimatic extremes (e.g., floods, droughts, heatwaves) under historical, contemporary and future climate conditions.
What infrastructure, programs and tools are used in your group? We use both the local PC and High-Performance Computing (HPC) systems tailored to specific needs. We use our in-house developed multi-scale hydrologic modeling system (www.ufz.de/mhm) and water quality models for large-scale simulations.
What could a guest researcher learn in your group? How could he or she support you in your group? Given the wide diversity of our work, we offer learning processes on the building blocks of mechanistic models, working with large-sample and large-scale databases. We welcome and invite researchers from different disciplines (e.g. computational hydrology, water quality, agronomy, and soil sciences) to work with us on synthesizing and documenting processes, patterns, and trends of water and nutrient flow under historical and future climate and socioeconomic conditions.
Helmholtz Centre for Ocean Research GEOMAR
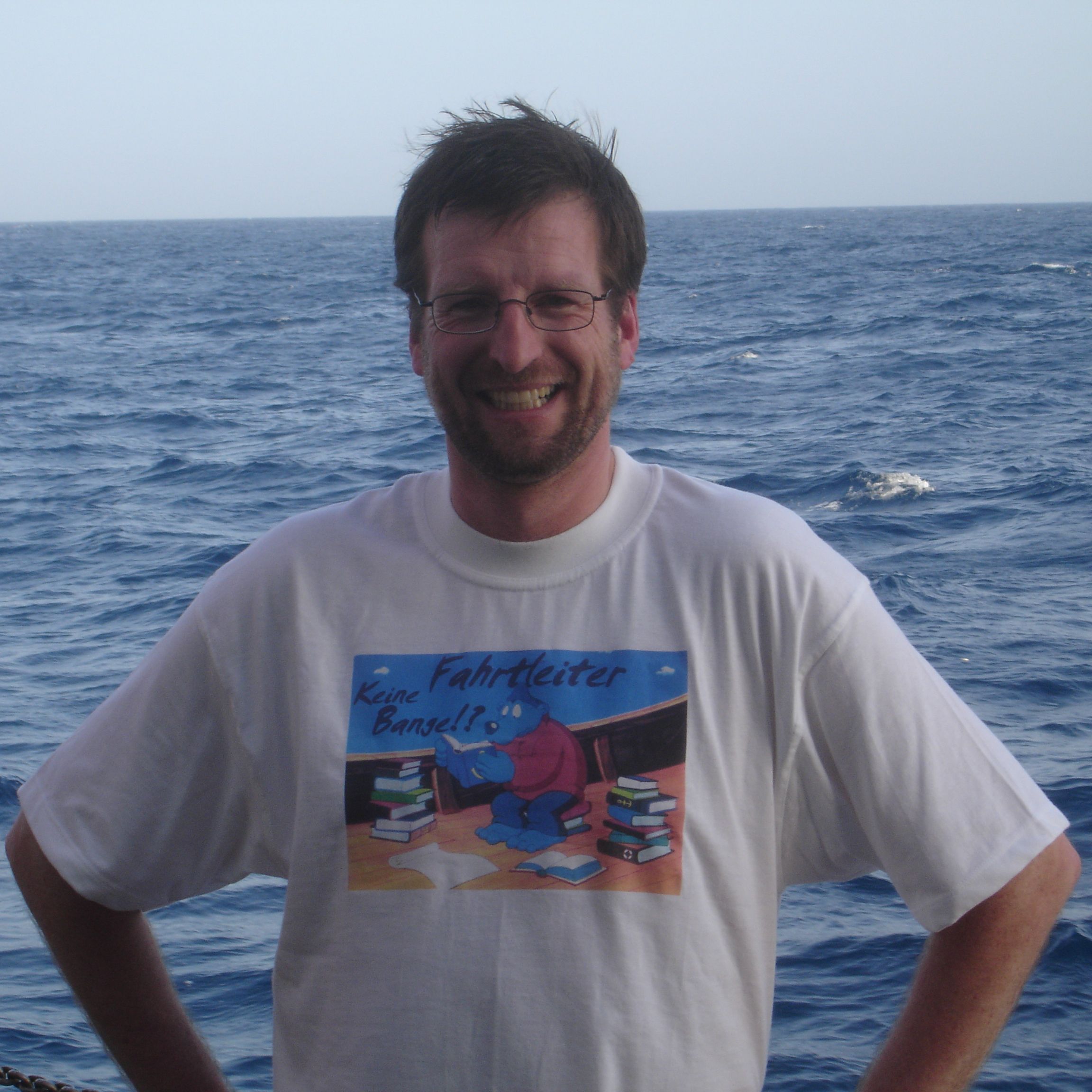
Hermann Bange
Hermann Bange - GEOMAR
GEOMAR Helmholtz Centre for Ocean Research Kiel - Marine Biogeochemistry (Res Div) / Chemical Oceanography (Res Unit) / Trace Gas Biogeochemisty (Working Group)
Short summary of your group's research: My working group is working on the biogeochemical pathways and emissions of climate -relevant trace gases such as N2O, CH4, CO, DMS and NO. Moreover, we run the Boknis Eck Time-Series Station (Eckernförde Bay, SW Baltic Sea) and the MEMENTO (the MarinE MethanE and Nitrous Oxide) database. We are operating worldwide.
What infrastructure, programs and tools are used in your group? - Boknis Eck Time-Series Station (www.bokniseck.de); Boknis Eck underwater observatory
- MEMENTO database (ttps://memento.geomar.de)
- Various research vessels
- Lab based measuerments
What could a participant of the HIDA Trainee Network learn in your group? How could he or she support you in your group? We offer:
- Work with time series data and databases (Boknis Eck, MEMENTO)
To want:
- Someone who to develop and realise new concepts for visualisation/integration/analysis of data sets.
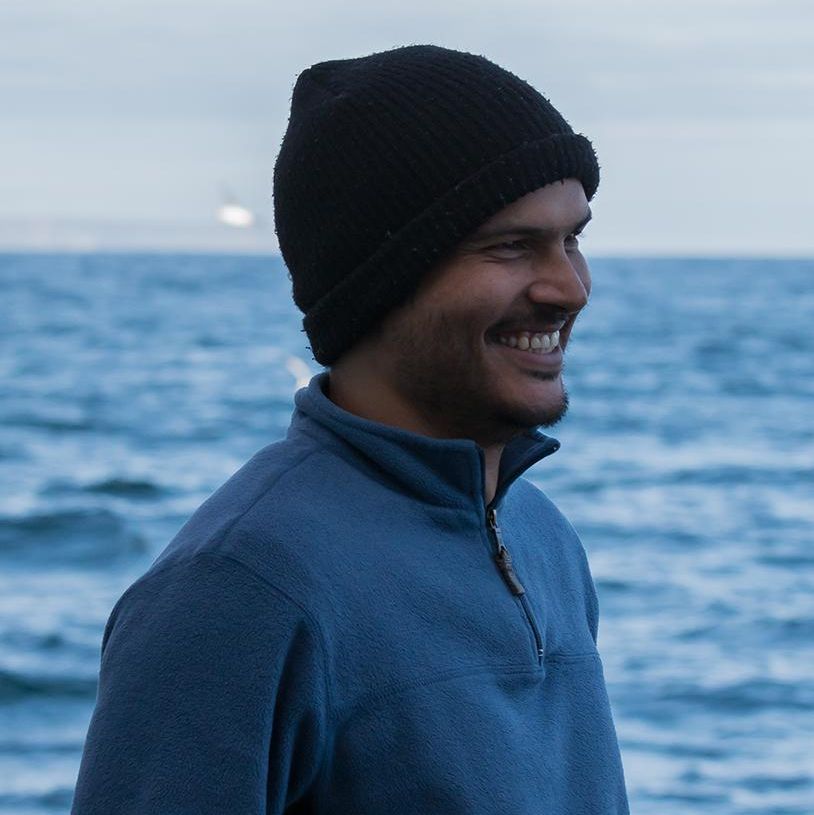
Amir Haroon
Amir Haroon - GEOMAR
GEOMAR Helmholtz Centre for Ocean Research Kiel - Dynamics of the Ocean Floor / Geodynamics
Short summary of your group's research: We apply numerous geophysical methods to understand sub-seafloor processes for tectonic, environmental and resource questions. Our expertise involves not only the evaluation of the individual methods / datasets, but has recently evolved into the application of state-of-the-art machine learning workflows to integrate disparate geophysical and geological data on various spatial scales.
What infrastructure, programs and tools are used in your group?
HPC Computing Centres at CAU Kiel
Open-source programms for interpreting Electromagnetic data
Python tools for ML
What could a participant of the HIDA Trainee Network learn in your group? How could he or she support you in your group? We are seeking for guest researchers with various geophysical data (e.g. CSEM, Seismics and Borehole Data) seeking to develop new concepts on integrating these into a consistent subsurface model.
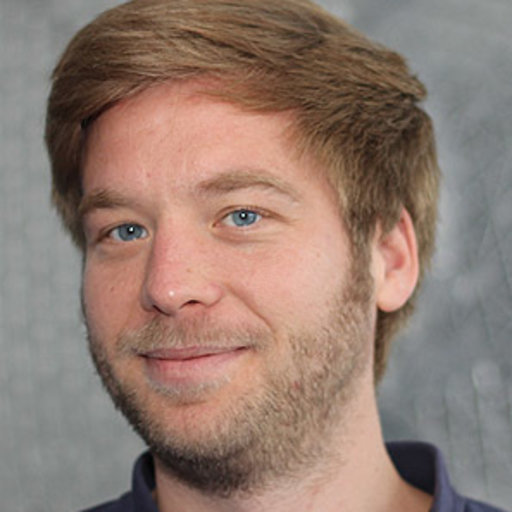
David Nakath
David Nakath - GEOMAR
GEOMAR Helmholtz Centre for Ocean Research Kiel - Marine Geosystems
Short summary of your group's research: The Oceanic Machine Vision Group at the GEOMAR works on the topic of optical underwater surveys employing artificial intelligence (AI) and classical computer vision approaches. To this end, we seek to enable cameras to serve as faithful measurement instruments and navigation sensors in the deep sea. The latter, visually challenging environment, presents us with a lot of geometric (refraction) and radiometric problems (attenuation, scattering) which we seek to solve.
What infrastructure, programs and tools are used in your group? We normally devise novel approaches writing Python / C++ Code on Linux machines storing it on GIT, potentially in some remote (over ssh) work. If desired, access to GPUs can be provided in addition.
The subsequent evaluation is often conducted on synthetic as well as real data. To this end, we maintain multiple sets of underwater imagery, specifically tailored to the problems we work on. We also have camera-light systems, which can be operated manually, inside a test tank or directly in the Baltic Sea. In addition, they can be attached to AUVs and the like, to capture actual deep sea datasets. Hence, new data can be taken, if necessary.
What could a participant of the HIDA Trainee Network learn in your group? How could he or she support you in your group? The guest can learn about synthesizing visual data or taking real data in controlled conditions in a test tank, or at the actual sea. Furthermore, we can provide deep insights about geometric and radiometric problems typically encountered in underwater scenarios. We have a wide range of topics inter alia covering refraction, color correction, medium estimation, light pose optimization, structure from motion, state estimation and image segmentation/classification. We will approach those problems with the means of classical computer vision but also with neural networks in conjunction with differentiable physical models. Finally, we would be happy if the guest researcher would co-author a research paper and/or contribute to our software.
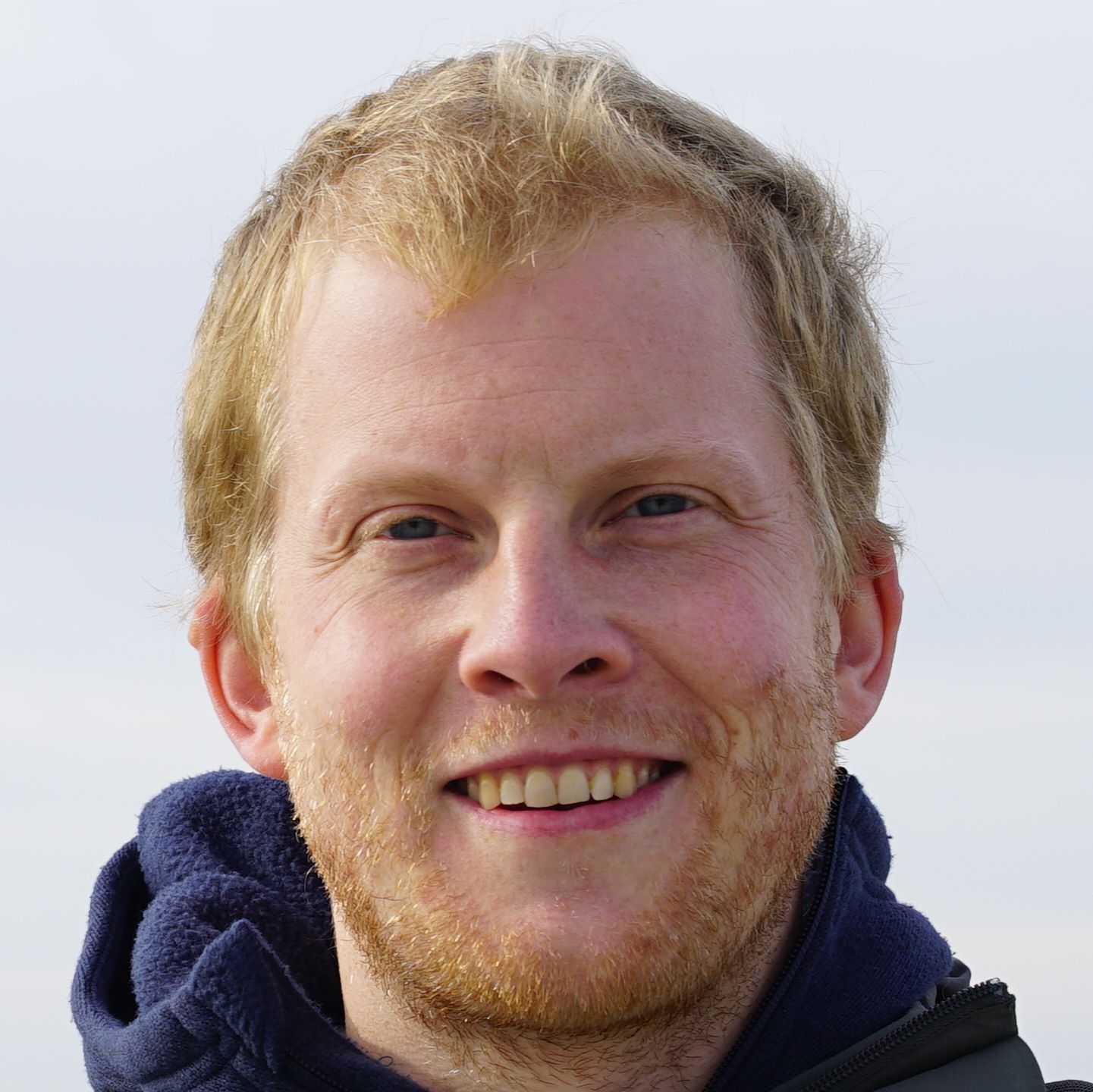
Timm Schoening
Timm Schoening - GEOMAR
GEOMAR Helmholtz Centre for Ocean Research Kiel - Data Science Unit
Three-sentence summary of your group's research: The Data Science Unit (DSU) of GEOMAR develops Data Science methods for marine research and applies them together with the researchers to the heterogeneous data of GEOMAR and its partner institutes. The DSU offers support and training on Data Science methods to researchers at GEOMAR. We aim to adress the grand challenges of marine science with data science methods.
What infrastructure, programs and tools are used in your group? We work with all data types relevant to the marine sciences: time series (e.g. in climate research, ecology), grids (e.g. in seafloor mapping, microscopy imagery), cubes (e.g. in ocean current simulation, 4D visualization). Our data products are developed and operated interactively (e.g. Jupyter notebooks) and most of the magic happens in Python. High-performance computing can be conducted on HGF computing ressources, at our compute cluster at Kiel University or on mobile GPU clusters for HPC computing at sea.
What could a guest researcher learn in your group? How could he or she support you in your group? Working with marine science data sets. Detecting and classifiying events in heterogeneous data (time series, grids, data cubes, ...). AI and ML methods. Supervised / unsupervised clustering of data. Integration of AI, simulation, and observation methods and data into Digital Twin frameworks. Working towards solutions for pressing ocean and society challenges.
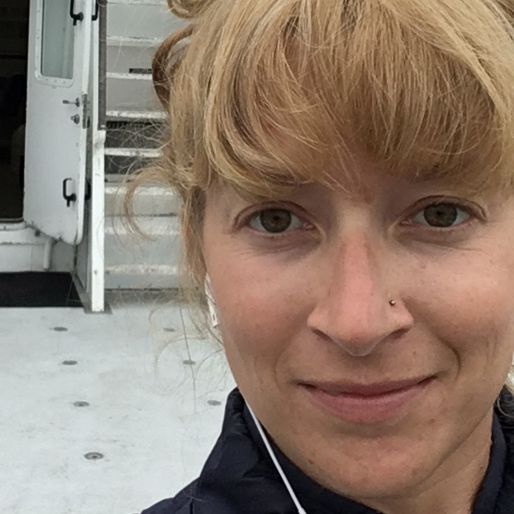
Kristin Bergauer
Kristin Bergauer - GEOMAR
GEOMAR Helmholtz Centre for Ocean Research Kiel - RD3/OEB
Three-sentence summary of your group's research: Our laboratory conducts research on single-celled organisms known as bacteria and archaea that have a crucial role in the cycling of (micro)nutrients in both the photic and aphotic regions of the global oceans. Our research in the field of biological oceanography encompasses the integration of marine microbial ecology and biogeochemistry, operating under the overarching framework of physical oceanography. At GEOMAR, our research focuses on investigating microbial B vitamins, ectoenzymes, and the functional capabilities of microorganisms. We employ integrated multi-omics approaches, together with laboratory studies conducted at the Boknis Eck Time-Series Station in the Southwest Baltic Sea and during oceanographic cruises.
What infrastructure, programs and tools are used in your group? State-of-the-art molecular tools are employed in our lab facilities to conduct experiments involving these organisms. These tools include e-DNA analysis, molecular laboratory techniques, flow cytometry, microscopy, and the use of genetically modified organisms (GMOs). Metagenomic, -transcriptomic, and -proteomic analyses generate a vast amount of data, which can be effectively analyzed by leveraging the high-performance computing cluster available at Kiel University. The analysis of Underwater Vision Profiler (UVP) images is presently conducted using the Quantitative Imagery Platform of Villefranche.
What could a guest researcher learn in your group? How could he or she support you in your group? The visiting scientist will have the opportunity to actively participate in ongoing research and gain knowledge regarding the bioinformatic analysis of multi-omic and e-DNA datasets. In summary, this encompasses the implementation of essential software, the development of procedural frameworks, computational methodologies for data-driven microbiology, and the utilization of visualization techniques.
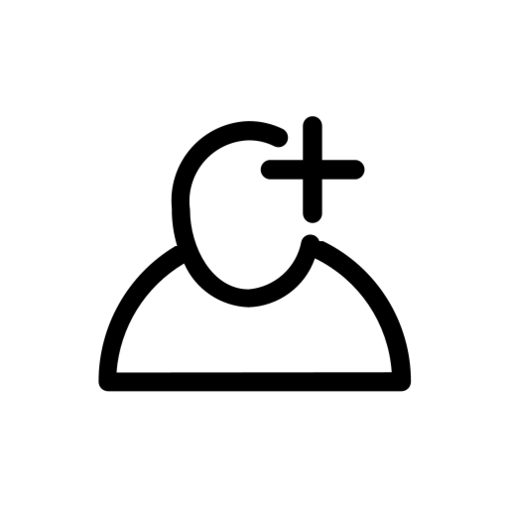
Gastgeber werden
Helmholtz-Gastgeber werden
Wenn Sie daran interessiert sind, eine Nachwuchswissenschaftlerin oder einen Nachwuchswissenschaftler aufzunehmen und Einblick in Ihre Forschung zu gewähren, wenden Sie sich bitte an teresa.weikert@helmholtz.de.
Alfred-Wegener-Institut - AWI
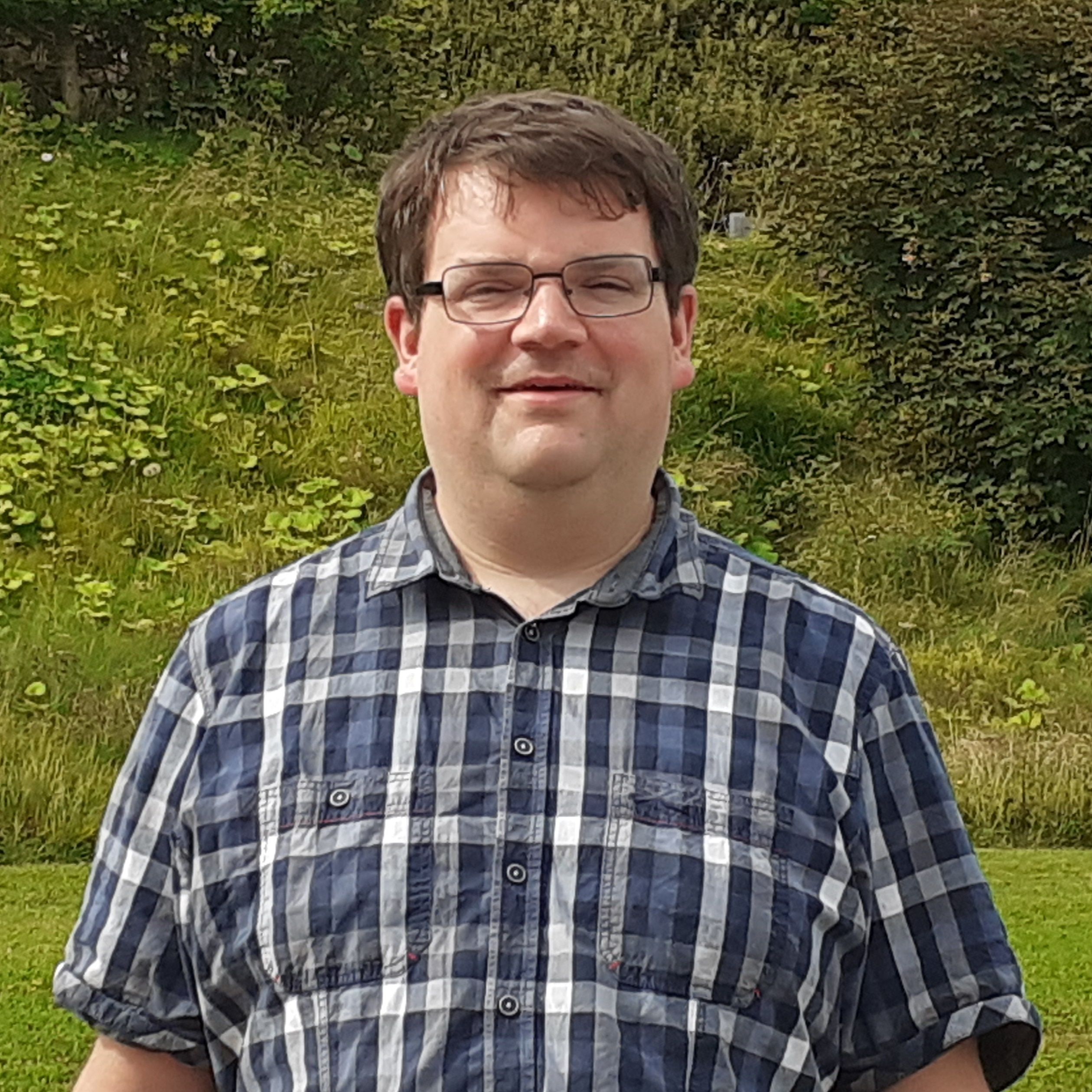
Sebastian Primpke
Sebastian Primpke - AWI
Alfred Wegener Institute, Helmholtz Centre for Polar and Marine Research - Shelf Sea Systems Ecology - Microbial Ecology/Microplastics
Short summary of your group's research: In the working group we work on analytical techniques for the determination of micro- and nanoplastics using chemometric approaches. Data analysis is mainly performed on hyperspectral FTIR and Raman images which are targeted by various chemometric approaches. In general, these approaches are linked to the environment by research cruises, projects and meta analyses.
What infrastructure, programs and tools are used in your group? We have several spectroscopic imaging instruments (FTIR / Raman) available, a selection of dedicated computers designed for data analysis. We are currently using Python and CUDA for data analysis.
What could a participant of the HIDA Trainee Network learn in your group? How could he or she support you in your group? Our group can provide a large material database, instruments and calculation power for the development of chemometric approaches. We can support the guest researcher in the development of their chemometric based analysis ideas and look forward to link these with our existing or planned approaches in form of for example future research proposals with the guest researcher.
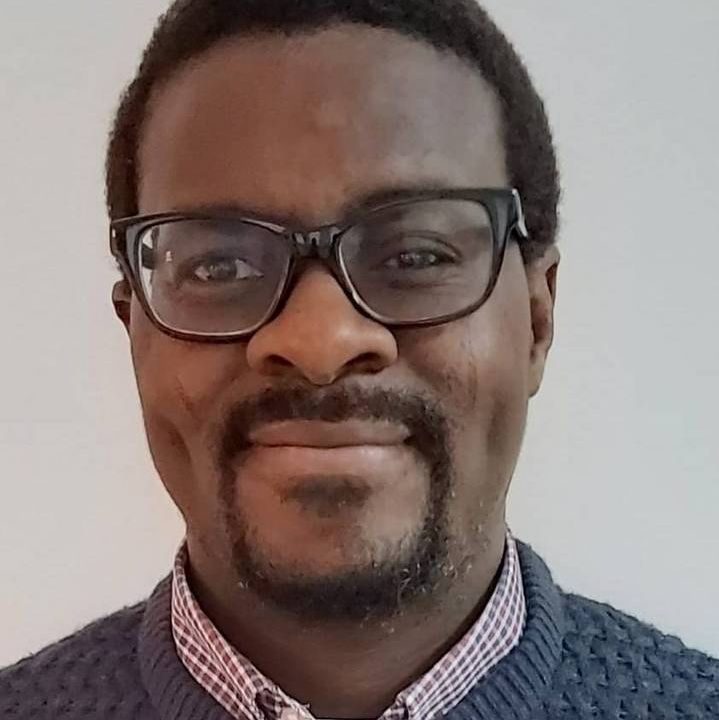
Olanrewaju Sulaiman Oladokun
Olanrewaju Sulaiman Oladokun - AWI
Alfred Wegener Institute, Helmholtz Centre for Polar and Marine Research - Shelf Sea Ecologogy / Marine Aquaculture
Short summary of your group's research: Marine Multiuse system involving mapping of aquaculture site within German Northsea, Development of digital reliability and ecological system from available data for safe deployment and operation of marine system, GUI and Gitlab Modeling of Aquaculture system operation.
What infrastructure, programs and tools are used in your group? Sea site simulation software species
What could a participant of the HIDA Trainee Network learn in your group? How could he or she support you in your group? Contribute GIT; GUI; Matlab Python coding

A. Murat Eren
A. Murat Eren - HIFMB
Helmholtz Institute for Functional Marine Biodiversity - Ecosystem Data Science Group
Three-sentence summary of your group's research: Our research program focuses on understanding the ecology and evolution of naturally occurring microbial life using integrated ‘omics strategies and laboratory experiments. Our group aims to develop new computational approaches and create advanced software platforms that intend to generate hypotheses from complex environmental data to bring us closer to explain mechanisms by which microbes interact with their surroundings, evolve, disperse, and initiate or adapt to environmental change. We are environment agnostic as microbial ecology and evolution happens everywhere, thus, our recent work ranges from the human gastrointestinal tract and oral cavity to sewage systems, and insect ovaries. That said, we find marine systems much more intriguing than most other habitats and desire to channel our future efforts to understanding microbial life in sunlit and dark oceans.
What infrastructure, programs and tools are used in your group? Having to work with extremely large and complex 'omics datasets, the initial stages of our data analyses typically rely on high performance computing infrastructures. Although, as we are often much more interested in targeted analyses of subtle phenomena that reveal specific insights for specific questions rather than overall analyses of our data, later stages of our data analyses often rely on R and/or Python programs and interactive visualization solutions.
Our group includes many of the key developers of anvi'o (https://anvio.org/), a comprehensive open-source software platform that brings together many aspects of today’s cutting-edge computational strategies of data-enabled microbiology, including genomics, metagenomics, metatranscriptomics, pangenomics, metapangenomics, phylogenomics, and microbial population genetics in an integrated and easy-to-use fashion through extensive interactive visualization capabilities.
Anvi'o stands on more than 200,000 lines of Python and JavaScript code that follow modern software development paradigms thanks to the voluntary contributions of more than 30 developers from the academia and industry to empower microbiologists for their complex needs through an architecture that enables reproducible science and simple interfaces for data access.
What could a guest researcher learn in your group? How could he or she support you in your group? Our group can help addressing novel questions through complex 'omics datasets and ad hoc software development, support others with insights from integrated 'omics strategies, and collaborate on integrating software solutions into the anvi'o software ecosystem to make them available to the broader community of life scientists.
Karlsruhe Institute for Technology - KIT
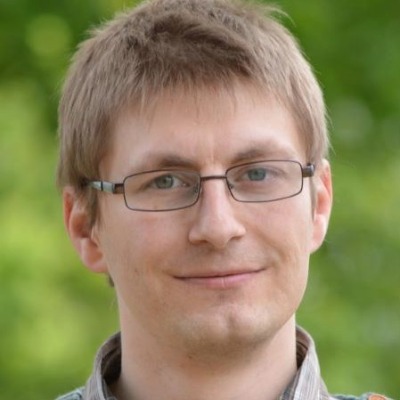
Jörg Meyer
JÖRG MEYER - KIT
Karlsruhe Institute of Technology (KIT) - Data Analytics, Access and Applications
Short summary of your group's research: The department Data Analytics, Access and Applications (D3A) at the Steinbuch Centre for Computing (SCC) performs research in applied Artificial Intelligence engineering, contributes to the European Open Science Cloud, has in-depth expertise in federated authentication and authorization infrastructures, and works on joint research of computer scientists and researchers from the field climate and environment. Recently, we launched a team working on the prospering field of Quantum Computing with a focus on hybrid quantum-classical machine learning algorithms.
What infrastructure, programs and tools are used in your group? Depending on the topic we use various programming languages and computing environments such as high performance computing clusters. For many challenges Python turned out to be the first choice.
What could a participant of the HIDA Trainee Network learn in your group? How could he or she support you in your group? As a candidate you will be part of our lively quantum computing team where you will gain or deepen your understanding on different aspects of quantum machine learning, our current research as well as algorithmic methods. You can further learn state-of-the-art software development methods by supporting us in extending and developing scientific open source software for quantum computing.
You can complement our interdisciplinary team by generalizing and evaluating our methods based on your own background and data and thereby enhance existing activities or even kickstart new activities.
Depending on your interests you can get an overview on other recent research topics in D3A and other research departments at SCC.
At present, Jörg Meyer can only accept applications from people interested in the HIDA Trainee Network, i. e. Helmholtz-internal candidates.
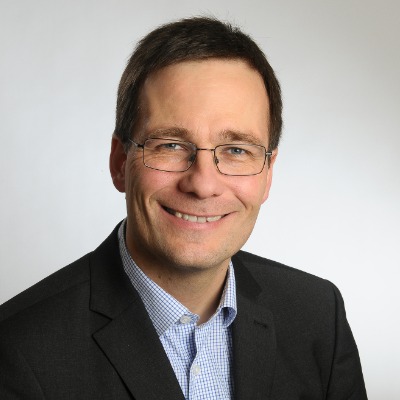
Peter Sanders
Peter Sanders - KIT
Karlsruhe Institute of Technology (KIT) - Algorithm Engineering
Short summary of your group's research: Efficient algorithms and data structures are the basis of all nontrivial computer applications. Algorithmics – the systematic development of efficient algorithms – is therefore crucial for transforming technological potential into applications that are important for technology, business, science, and our daily lives. At the institute of theoretical informatics, our group focuses on the "basic toolbox" of methods that are needed in many applications, e.g., sorting, hash tables, index data structures, route planning in graphs, or partitioning graphs. A particular focus is on parallel algorithms ranging from shared-memory machines to the largest supercomputers. Here, basic tools we develop include load balancing, collective communication primitives, and (hyper)graph partitioning.
Helmholtz researchers outside computer science might profit from a cooperation with our group if improved basic tools can remove performance bottlenecks in their applications.
What infrastructure, programs and tools are used in your group? C++, MPI, Intel TBB, OpenMP. Our own software libraries for sorting, (compressed) hash tables, search trees, (hyper)graph partitioning, route planning,... Various parallel computers ranging from shared-memory machines to large supercomputers (e.g. SuperMUC-NG).
What could a participant of the HIDA Trainee Network learn in your group? How could he or she support you in your group?
Learn: algorithm engineering (i.e., design, analysis, implementation and experimental evaluation as a holistic process), understanding of scalability issues. Use of our techniques and software.
Support: Develop improved basic algorithmic tools with us. Engineer you application with us using our techniques, algorithms, and software libraries. A goal would be to have a prominent joint publication.
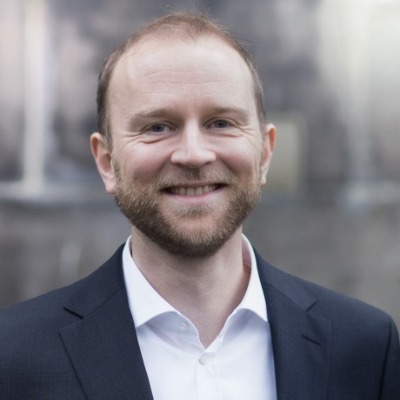
Martin Frank
Martin Frank - KIT
Karlsruhe Institute of Technology (KIT)- Computational Science & Mathematical Methods
Short summary of your group's research: The research group Computational Science and Mathematical Methods (CSMM) under the supervision of Prof. Dr. Martin Frank is an interdisciplinary research group working on different challenges in mathematical modeling. We are unified by our interest in method-oriented mathematics, in mathematical modeling inspired by applications, and in the didactics of mathematical modeling. Our research focuses on bringing modern mathematical techniques such as modeling, simulation, optimization, inverse problems, uncertainty quantification, and machine learning/artificial intelligence into the real-world. Furthermore, we take an interest in kinetic theory, developing models, numerical methods and software implementations and combining them with the aforementioned techniques.
What infrastructure, programs and tools are used in your group? Among other things, we use the modern high performance computing infrastructure of KIT, namely bwUniCluster. We develop software and tools in Julia, Python, C++ and Matlab, depending on the project. We employ several project specific software stacks for uncertainty quantification and medical image processing.
What could a participant of the HIDA Trainee Network learn in your group? How could he or she support you in your group? As an interdisciplinary research group, we offer a lot of possibilities for a participant of the HIDA Trainee Network and many different interesting projects to support. A mathematically oriented participant could learn interesting aspects about machine learning based numerical methods for kinetic equations. Furthermore, the group offers projects in mathematical methods for uncertainty quantification and dynamical low rank.
An application-oriented participant may find interest in the project about neural network based medical image processing or uncertainty quantification in radiation therapy.
A participant, who is interested in teaching, can learn about bringing modern and method oriented mathematics to the classroom and can work on applied mathematical projects with high school students.
We develop an open source simulation toolkit for radiation therapy planning, which also offers the possibility to engage and participate. Detailed descriptions of our projects can be found on our group homepage.
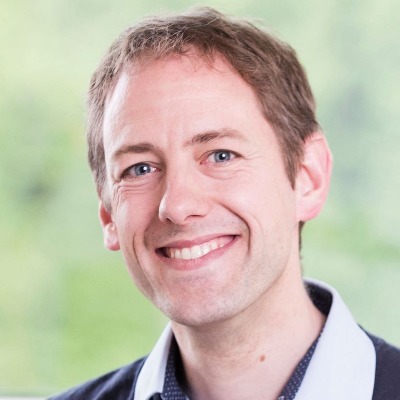
Jan Cermak
Jan Cermak - KIT
Karlsruhe Institute of Technology (KIT) - Satellite Climatology
Short summary of your group's research: We focus on the development, validation and application of geophysical remote sensing techniques, with special attention to the Earth surface - atmosphere interface. Topics of special attention include cloud processes, air pollution, land-surface temperature and interactions between the land surface and lower atmosphere. Our methods are primarily remote sensing and machine learning.
What could a participant of the HIDA Trainee Network learn in your group? How could he or she support you in your group? Collaboration is at the core of our approach to research. We can offer the opportunity to learn in this exchange about our topics and methods. KIT is one of the most exciting locations for atmospheric research, with world-class infrastructures, several seminar series and numerous project links to other institutions around the world. You will become part of this environment.
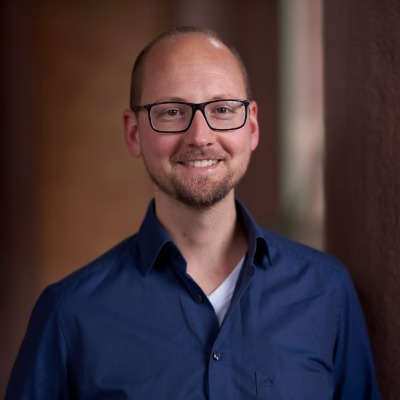
Pascal Friedrich
Pascal Friedrich - KIT
Karlsruhe Institute of Technology (KIT) - Artificial Intelligence for materials science (AiMat), Department of Informatics
Short summary of your group's research: The AiMat group works on the development and application of AI and machine learning (ML) methods for materials science and chemistry. Focus topics are (graph) neural networks for the prediction of molecular and materials properties, the combination of interpretable AI methods, ML-enhanced simulations and automated experiments to autonomous materials acceleration platforms, and generative models for inverse materials design.
What infrastructure, programs and tools are used in your group?
Infrastructure: HPC systems as BWUniCluster and HoreKa
Programs: Python and machine learning libraries, as well as quantum chemistry simulations
What could a participant of the HIDA Trainee Network learn in your group? How could he or she support you in your group? We welcome participants with either a background in machine learning and methods development, who are interested in applying the models to interesting scientific questions, or participants with a background in materials science/chemistry/physics, who want to learn more about machine learning and teach us about their application areas!
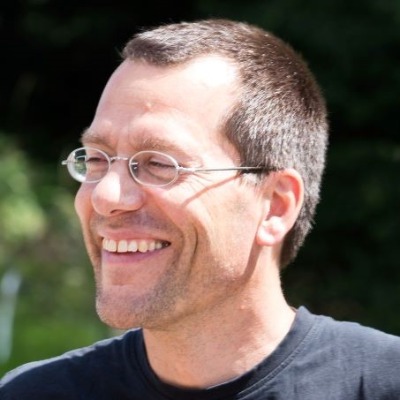
Uwe Ehret
Uwe Ehret - KIT
Karlsruhe Institute of Technology (KIT) - Institute of Water and River Basin Management IWG
Short summary of your group's research: Our group has a wide range of hydrology-related interests comprising conceptual and physically based hydrological modeling, ecohydrology, application of thermodynamic principles to hydrology, data-infrastructures and data-based learning. In the context of HIDA, especially the latter topic is of interest: My colleague Ralf Loritz and I focus on applying machine-learning approaches to hydrological modeling, and applying concepts from information theory for both hydrological system analysis and as a basis for building hybrid hydrological models merging information from data and knowledge.
What infrastructure, programs and tools are used in your group? We have developed and use a range of open-source Matlab- and Python-based tools for analysis and modeling in hydrological and geostatistical contexts. See more on Link.
What could a participant of the HIDA Trainee Network learn in your group? How could he or she support you in your group?
What you can learn from us:
- Applying state-of-the-art tools for supervised and unsupervised learing in hydrological settings
- Applying state-of-the-art machine-learning tools for hydrological forecasting and prediction
- The beauty and generality of concepts from information theory, and how they can be used for hydrological analysis and model building
What we can learn from you:
- A fresh look and feedback on what we do
- New insights into our methods by applying them to your data and problems
- Learn about alternative methods
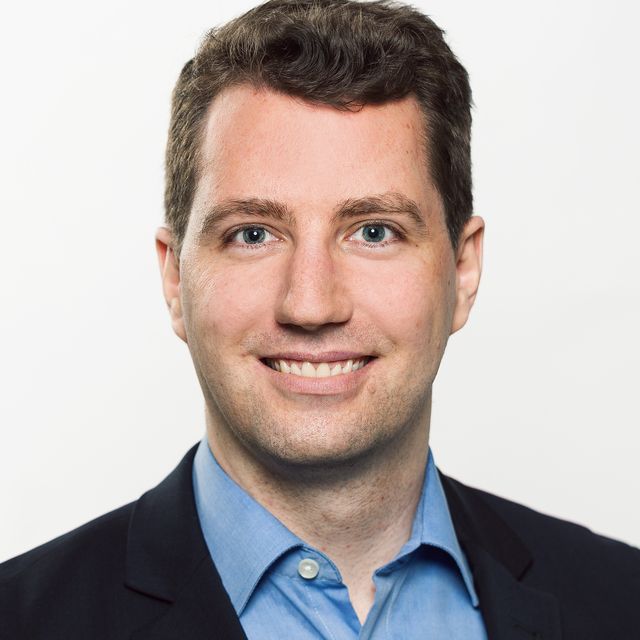
Michael Färber
Michael Färber - KIT
Karlsruhe Institute of Technology (KIT) - Web Science, Institute for Applied Informatics and Formal Description Methods
Short summary of your group's research: The research group "Web Science," headed by Dr. Färber, deals with data science and web science. The focus is on the development and application of artificial intelligence (AI) methods. This includes the semantic representation of knowledge through knowledge graphs, machine learning (e.g. for search and recommendation systems), and natural language processing.
What infrastructure, programs and tools are used in your group?
HPC systems (e.g., www.nhr.kit.edu/userdocs/horeka/, wiki.bwhpc.de/e/Category:BwUniCluster_2.0); own server infrastructure for experiments and demonstrations; programming typically in Python.
What could a participant of the HIDA Trainee Network learn in your group? How could he or she support you in your group? We welcome participants with a background in natural language processing, knowledge graphs, or machine learning (e.g., with a degree in computer science, industrial engineering, computational linguistics, mathematics, or a related subject), who want to perform joint research with us and publish the results at international venues. We offer a modern workplace, a pleasant working atmosphere, and flexible working times. Current research topics include the combination of deep neural networks with knowledge graphs, information extraction for knowledge graph creation, and using knowledge graphs for (explainable) recommender systems. We also welcome new research ideas.
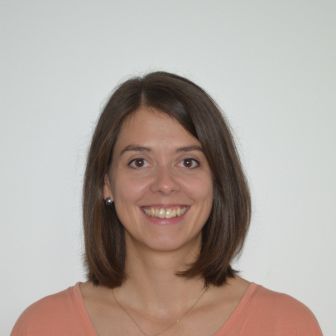
Martina Klose
Martina Klose - KIT
Karlsruhe Institute of Technology (KIT) - Helmholtz Young Investigator Group "Mineral Dust" at the Institute of Meteorology and Climate Research - Department Troposphere Research
Short summary of your group's research: We investigate mineral dust processes with the goal to better quantify the (dust) aerosol cycle and its impacts on climate and environment. Our focus is on dust emission, dust-cloud interactions, interactions of dust with other aerosols, as well as on land-surface properties and their changes. In our research, we apply and develop numerical models, study processes theoretically, and conduct field and laboratory experiments.
What infrastructure, programs and tools are used in your group? High-performance-computing; weather models such as ICON-ART; observational instrumentation
What could a participant of the HIDA Trainee Network learn in your group? How could he or she support you in your group? We can offer expertise with numerical modeling and working with heterogeneous data sets, such as model and observational data. We are interested in expanding our activities toward artificial intelligence and novel statistical methods for data analysis.
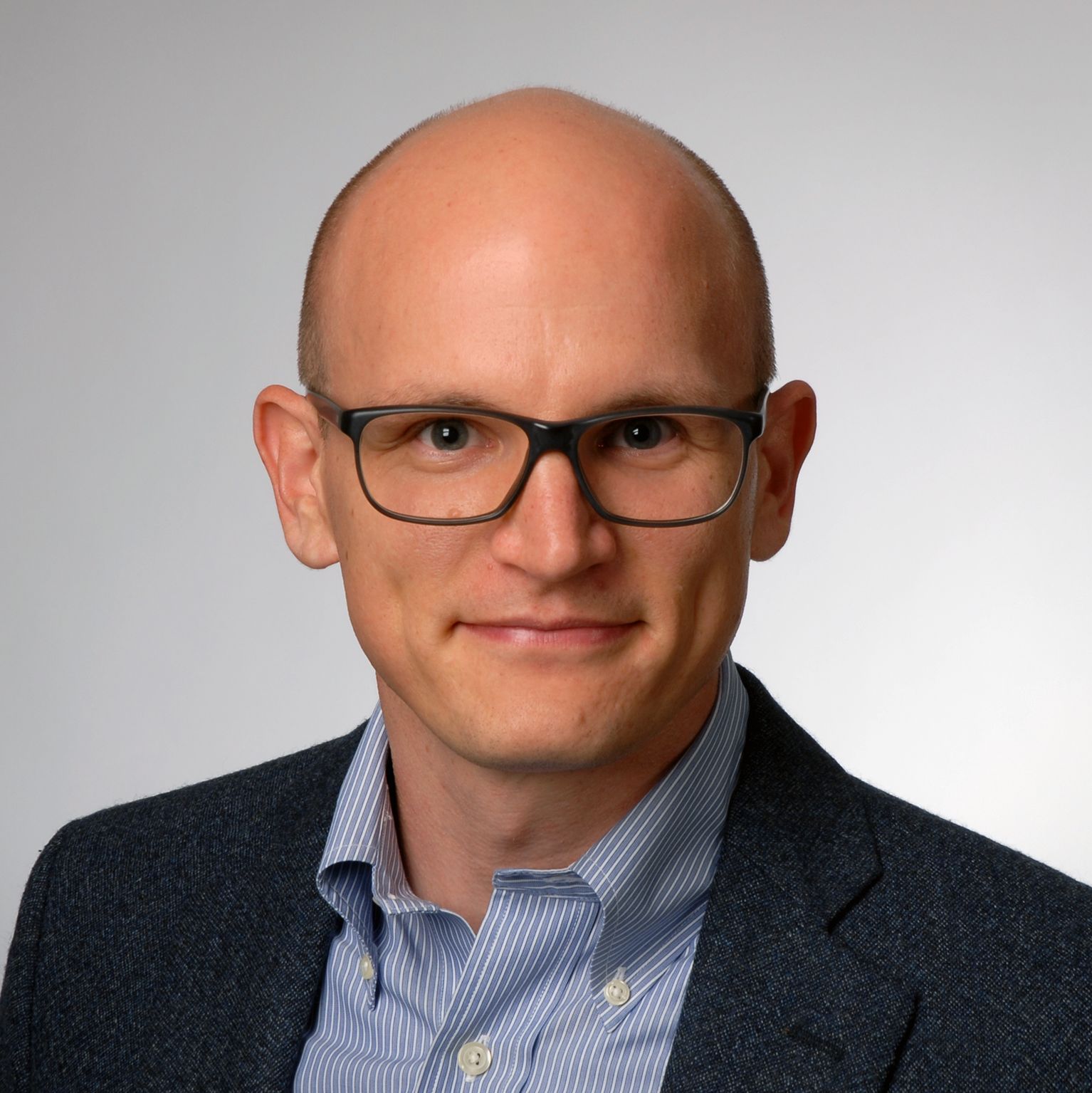
Sebastian Krumscheid
Sebastian Krumscheid - KIT
Karlsruhe Institute of Technology (KIT)- UQ - Junior Research Group Uncertainty Quantification
Short summary of your group's research: We develop advanced mathematical and numerical techniques for the treatment and quantification of uncertainties in complex computational models. Our research focuses on theoretical and methodological aspects, as well as on interdisciplinary projects where theoretical sound methodologies are tailored to applications. For these tasks, we employ a variety of techniques, including tools for high-dimensional approximations and machine learning, Bayesian approaches for inverse problems and data assimilation, as well as efficient and robust sampling methods.
What infrastructure, programs and tools are used in your group? Our interdisciplinary research focus necessitates the use of a wide range of tools. For example, we implement our developed mathematical methodologies in modern programming languages, such as Python, C++, Matlab, and R, depending on the project. Moreover, we take advantage of the modern high-performance computing infrastructure at KIT for computational-intensive tasks.
What could a participant of the HIDA Trainee Network learn in your group? How could he or she support you in your group? As a participant, you will become part of a highly motivated team. You will be able to learn about advanced mathematical and computational methodologies for the treatment and quantification of uncertainties at the interface of computational models and data. With your expertise, we will carry out novel interdisciplinary research by applying our methodologies to your data and problems. Furthermore, your application oriented expertise could inspire new ideas at the intersection of computational models and data that may spark new collaborative research activities.
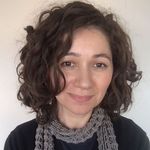
Sanja Lazarova-Molnar
Sanja Lazarova-Molnar - KIT
Karlsruhe Institute of Technology (KIT) - Systems, Data, Simulation & Energy (SYDSEN)
Three-sentence summary of your group's research: The research group Systems, Data, Simulation & Energy (SYDSEN) is concerned with the advancement of the field of Modeling and Simulation by developing new methods to utilize nowadays prevalently available data from easily accessible Internet of Things (IoT) devices. In addition, the research group looks at the synergies between Artificial Intelligence and Simulation to enhance both areas.
The application areas focus on cyber-physical systems, including smart factories and energy systems, and enhancing various performance metrics, such as energy efficiency, production and reliability.
What could a guest researcher learn in your group? How could he or she support you in your group? How to use data-driven methods to enhance modelling and simulation processes.
The guest researcher can participate with collaboration in ongoing or new research, as well as knowledge dissemination and participation in classes.
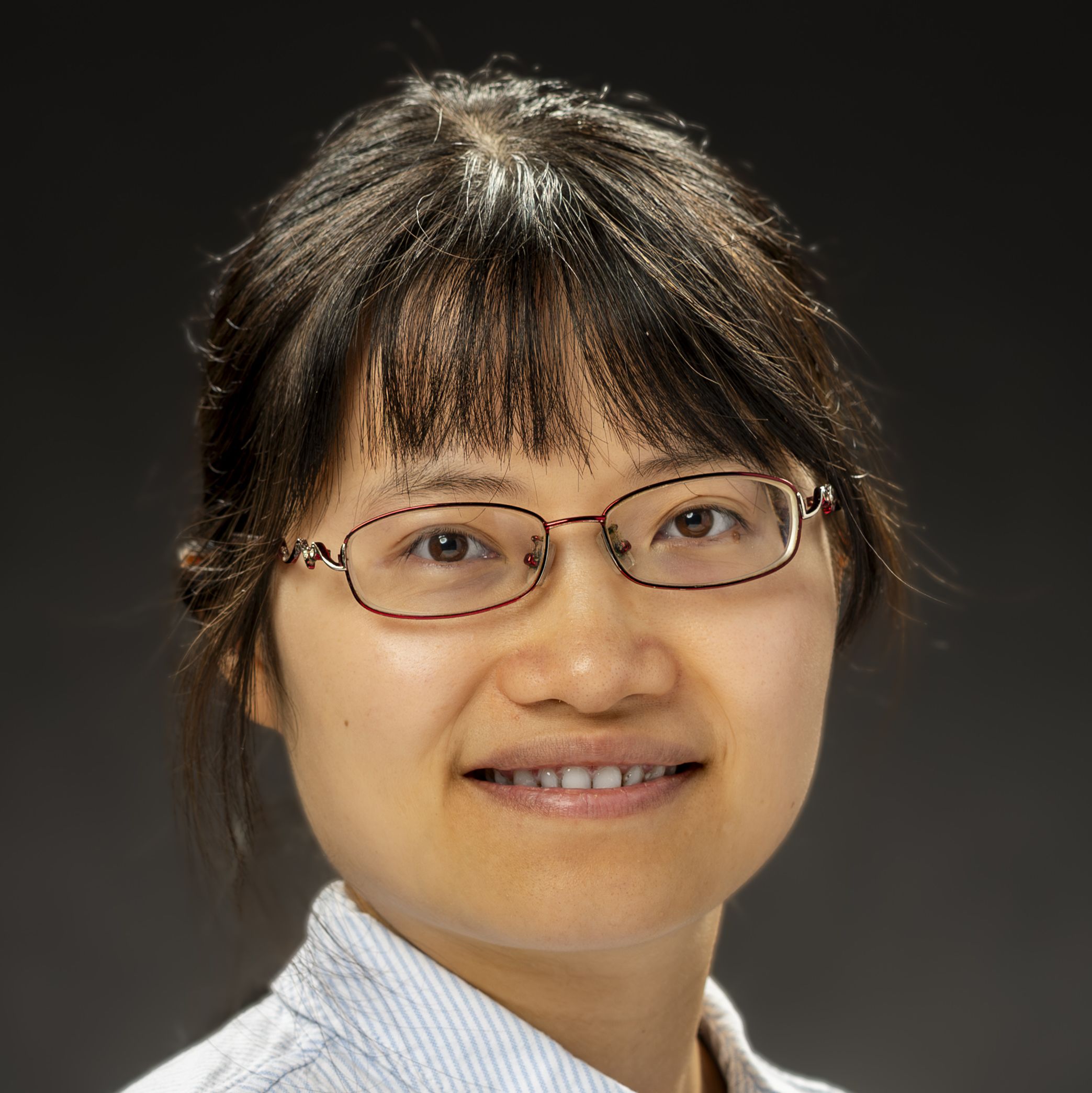
Jingyuan Xu
Jingyuan Xu - KIT
Karlsruhe Institute of Technology (KIT) - Institute of Microstructure Technology
Short summary of your group's research: My research focuses on:
- development of elastoclaoric cooling device
- shape memory alloys and elastomers for elastocaloric cooling
- solar systems (PV, solar thermal, PVT) for cooling, heating and power
- solid-state cooling/heat pump
What could a participant of the HIDA Trainee Network learn in your group? How could he or she support you in your group? Next-generation sustainable zero-carbon energy technologies for cooling, heating and/or power, with emphasis on the renewable energy sources (e.g., solar energy) and/or the waste heat, with the ultimate goal of reducing greenhouse gas emissions and mitigating the impacts of climate change for sustainable future.
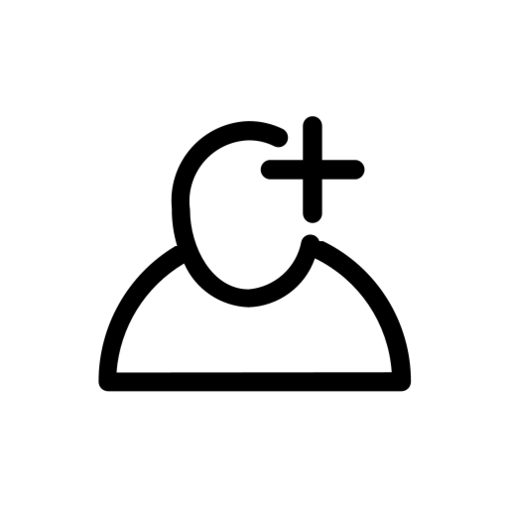
Gastgeber werden
Helmholtz-Gastgeber werden
Wenn Sie daran interessiert sind, eine Nachwuchswissenschaftlerin oder einen Nachwuchswissenschaftler aufzunehmen und Einblick in Ihre Forschung zu gewähren, wenden Sie sich bitte an teresa.weikert@helmholtz.de
Helmholtz-Zentrum Berlin für Materialien und Energie - HZB
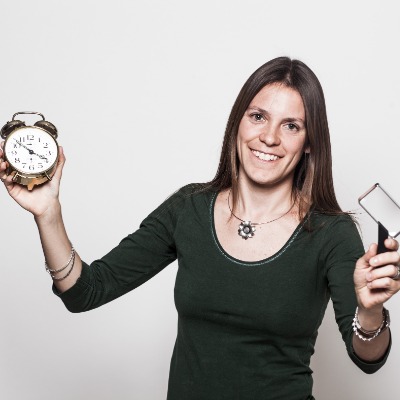
Annika Bande
Annika Bande - HZB
Helmholtz-Zentrum Berlin für Materialien und Energie (HZB)- Young Investigator Group Theory of Electron Dynamics and Spectroscopy
Short summary of your group's research: The electronic Schrödinger equation encodes the excited states of any material and their interaction with light may it be in electron dynamics or spectroscopy. We solve the equations in particular for nanomaterials accounting for their chemical environment. Particular interest lies in employing the recent methods of data science and quantum computation along with the traditional theories.
What infrastructure, programs and tools are used in your group? Different commercial and self-written quantum chemistry codes
What could a participant of the HIDA Trainee Network learn in your group? How could he or she support you in your group? Learn: Questions from the domain science quantum chemistry to data science, in particular handling of scarce data. Cutting-edge method development. Support: Overview knowledge on data science and experience in formulating questions properly for a data-driven solution.
Max Delbrück Center for Moleculare Medicine in the Helmholtz Association (MDC)
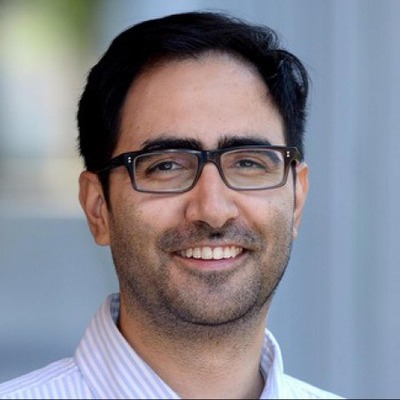
Altuna Akalin
Altuna Akalin - MDC
Max Delbrück Center for Molecular Medicine in the Helmholtz Association (MDC) - Bioinformatics and Omics Data Science Platform
Short summary of your group's research: We have a broad interest in gene regulation, specifically transcriptional regulation and association of transcriptional regulation with epigenomics. We are aiming to use data intensive computational methods to uncover patterns in gene regulation relating to cell differentiation and complex diseases.
What infrastructure, programs and tools are used in your group? HPC, statistical and machine learning tools implemented in Python or R
What could a participant of the HIDA Trainee Network learn in your group? How could he or she support you in your group? Participant can learn domain specific data processing techniques and provide support in machine learning applications.
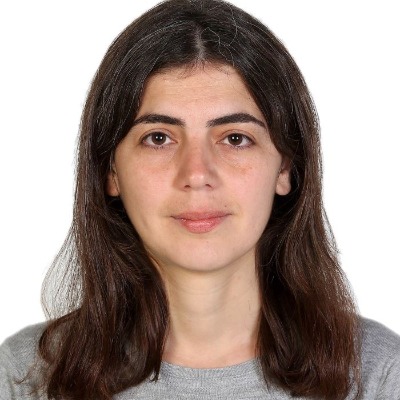
Laleh Haghverdi
Laleh Haghverdi - MDC
Max Delbrück Center for Molecular Medicine in the Helmholtz Association (MDC) - Berlin Institute for Medical Systems Biology (BIMSB), Computational methodologies and omic analytics
Short summary of your group's research: We are a computational biology group working with a range of single-cell omic data modalities including proteomics, transcriptomics, epigenetics and genomics to study biological systems such as development, haematopoiesis or leukaemic stem cells and their niche.Establishment of efficient computational methodologies for analysis of large single-cell omic data sets, resolution of complex lineage trees, data integration and interpretation across multiple modalities and assays as well as mathematical formulation of the applied methods are of central interest in the group.
What infrastructure, programs and tools are used in your group? Python, R, computational tools for single-cell data analysis
What could a participant of the HIDA Trainee Network learn in your group? How could he or she support you in your group? Hight-throughtput measurements of molecular states at the single-cell level are today accessible for biological and clinical studies, thanks to new and still evolving technological developments. Located in the cool central Berlin district, we are an interdisciplinary group trained in physics, mathematics, bioinformatics and molecular biology working together to address several aspects of computational analysis of such newly emerging datasets. We are interested in extending our machine learning and data science collaborations and contacts.
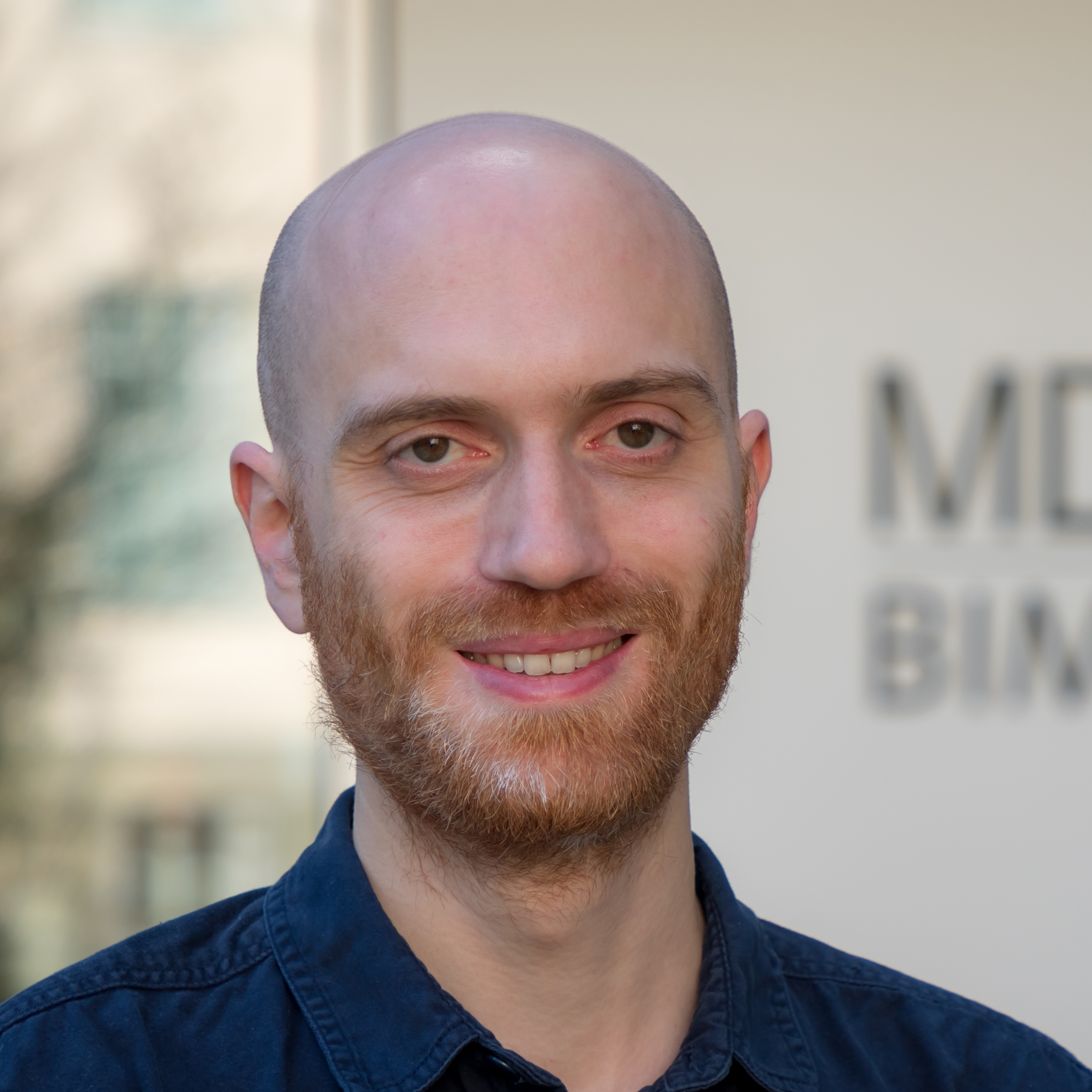
Jakob Metzger
Jakob Metzger
Max Delbrück Center for Molecular Medicine in the Helmholtz Association (MDC) - Metzger Lab
Short summary of your group's research: We are an interdisciplinary group focusing on a quantitative understanding of human neurodevelopment using stem-cell derived organoids. Using machine learning to analyze the difference between normal and pathological processes, we aim to dissect the mechanisms of neurodevelopmental and neurodegenerative diseases.
What infrastructure, programs and tools are used in your group? Python, R, machine learning
What could a participant of the HIDA Trainee Network learn in your group? How could he or she support you in your group? As an interdisciplinary group, we apply a range of computational tools to the analysis of biological data, including imaging and genomics data. Participants can learn data analysis techniques and provide support in developing new data science pipelines, such as machine learning applications.
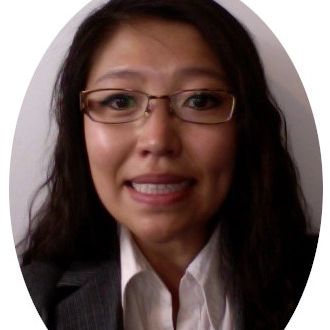
Claudia Chien
Claudia Chien - MDC
Max Delbrück Center for Molecular Medicine in the Helmholtz Association (MDC) - Experimental and Clinical Research Center (ECRC) - Paul Lab
Short summary of your group's research: Our group follows a translational clinical research approach. This means that we try to transfer new developments and findings from basic research directly into clinical work. We mainly focus on research in multiple sclerosis and neuroinflammatory diseases, however the MRI Team within AG Neuroimmunology has several projects that investigate other disorders. These imaging analysis methods and techniques are translatable to many different central nervous system studies.
What infrastructure, programs and tools are used in your group? Berlin Center for Advanced Neuroimaging, NeuroCure Clinical Research Center, Neuroimmunological Colloquium, Berlin Institute of Health, Bernstein Center for Computational Neurosciences
What could a participant of the HIDA Trainee Network learn in your group? How could he or she support you in your group?A guest researcher would learn how to investigate MRI parameters from clinical studies from real patients and volunteers. The researcher would be expected to support our group with set up of analysis pipelines of raw MRIs using high performance computing (https://www.hpc.bihealth.org/) resources and learn how to quality control clinically relevant data.
German Center for Neurodegenerative Diseases - DZNE
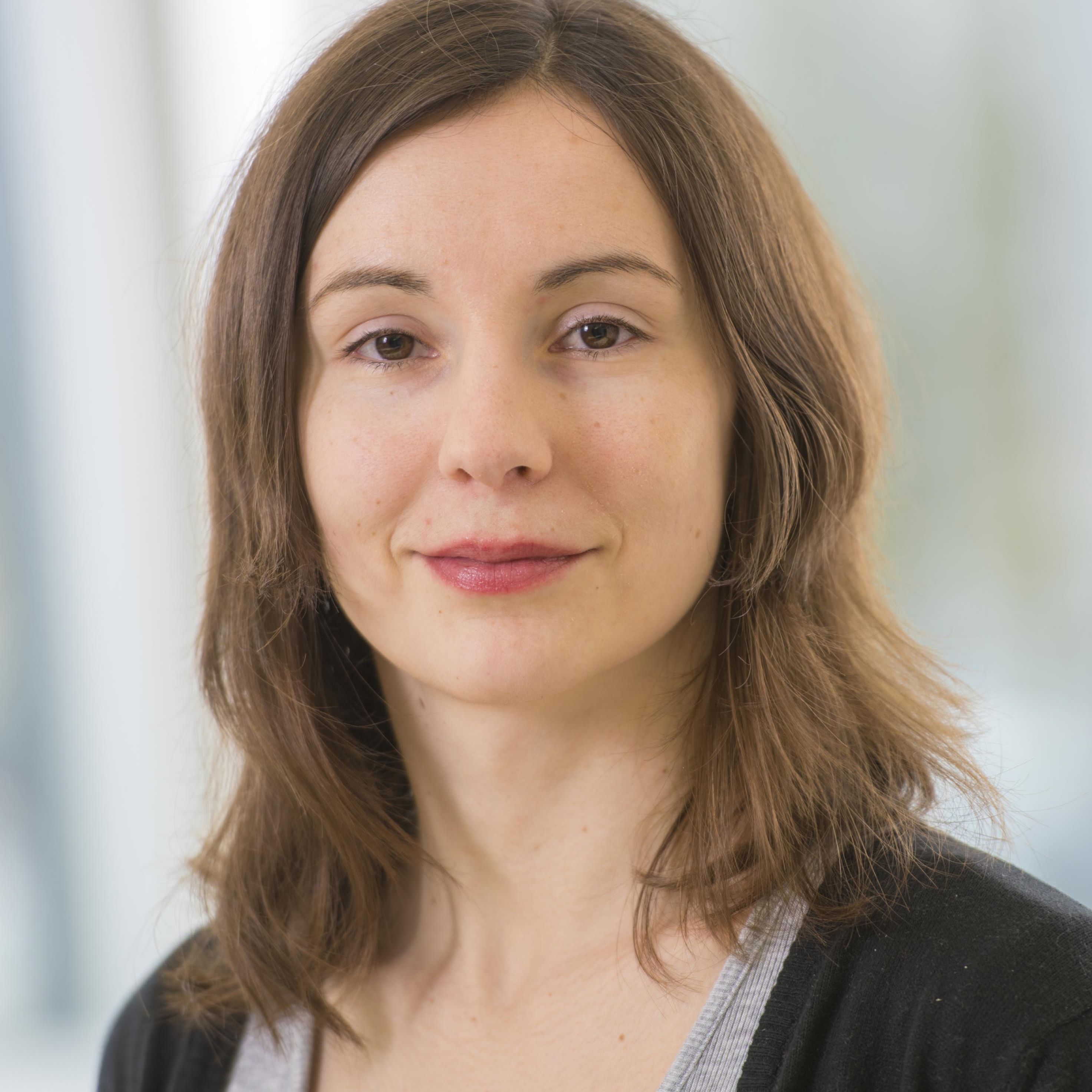
Maren Büttner
Maren Büttner - DZNE
German Center for Neurodegenerative Diseases (DZNE) - Systems Medicine
Short summary of your group's research: Systems medicine at the DZNE aims to develop holistic approaches to tackle diseases such as Alzheimer’s, Parkinson’s, or amyotrophic lateral sclerosis (ALS) and translate new insights into the clinics. We aim to change how flow cytometry data is currently analyzed. Technological advances in flow cytometry increased the possible number of features to be comparable to mass cytometry. However, the most widely used analysis methods for flow data is the targeted gating approach, which relies on manual selection of cell populations. This approach has several shortcomings as results vary across experts and for one person over time, does not scale well for hundreds of patients and samples, and tends to focus on known cell types, such that new discoveries especially in the disease context become unlikely.
What could a participant of the HIDA Trainee Network learn in your group? How could he or she support you in your group? A guest researcher can learn how to leverage deep learning models to jointly integrate flow cytometry data from different sites and enable joint integration without sharing data with our swarm learning approach.
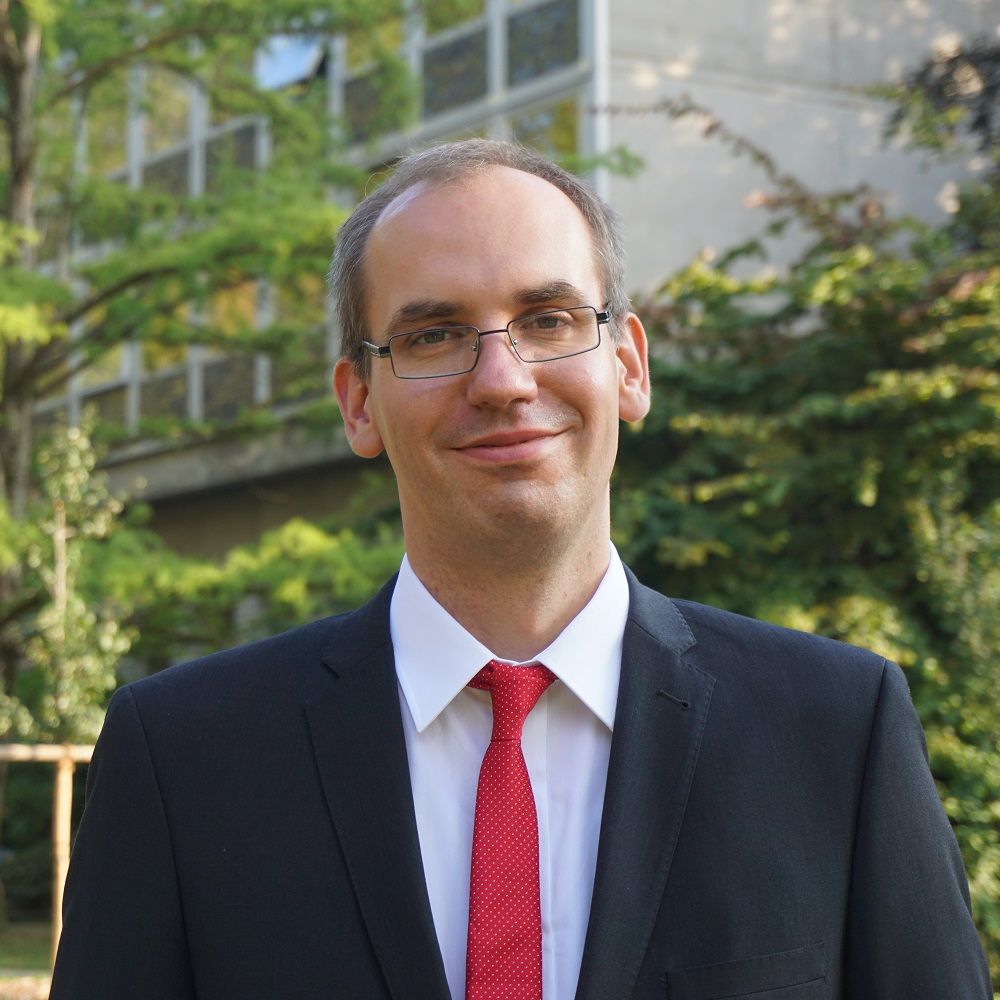
Matthias Becker
Matthias Becker - DZNE
German Center for Neurodegenerative Diseases (DZNE) - Modular High Performance Computing and Artificial Intelligence
Short summary of your group's research: The Modular High Performance Computing and Artificial Intelligence group works on building and improving computational architectures for growing data sets in neurodegeneration with the focus on memory-centric systems. Furthermore, we employ swarm learning for federated machine learning in many different applications. Another application of artificial intelligence is the use of GANs and VAEs to create synthetic cohorts in a Helmholtz AI project.
What infrastructure, programs and tools are used in your group?
- We use high-memory systems (6TB+) for improving the processing of genomics data analysis applications using c/C++ and Rust.
- For ML applications, we have GPU ressoucres available at the institute and access to Jülich. Development is mainly done in Python using common libraries like Pytorch (Lightning) or Tensorflow.
What could a participant of the HIDA Trainee Network learn in your group? How could he or she support you in your group? A guest researcher will join a highly interdisciplinary group at the intersection of computer science and medicine. We are well integrated with in the systems medicine research area and collaborate with clinical partners. Projects can be either focused on the performance analysis and optimization of data processing task in genomics or on ML applications. Here the implementation of a swarm learning use case in a new domain would be option as well as work synthetic cohort generation.
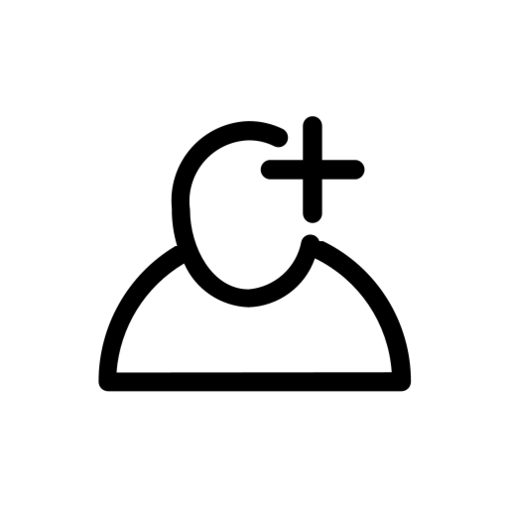
Gastgeber werden
Helmholtz-Gastgeber werden
Wenn Sie daran interessiert sind, eine Nachwuchswissenschaftlerin oder einen Nachwuchswissenschaftler aus einem anderen Helmholtz-Zentrum aufzunehmen und Einblick in Ihre Forschung zu gewähren, wenden Sie sich bitte an teresa.weikert@helmholtz.de
German Cancer Research Center - DKFZ
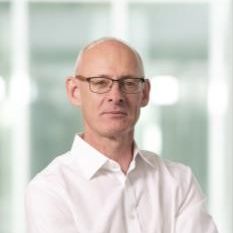
Oliver Jäkel
Oliver Jäkel - DKFZ
German Cancer Research Center (DKFZ) - Division of Medical Physics in Radiation Oncology
Short summary of your group's research: The division of medical physics in radiation oncology at DKFZ comprises multiple research groups, which cover all steps of the radiation treatment chain. We apply machine learning for improving innovative radiotherapy techniques using photons and ion beams. This includes technical developments, improvement of patient-oriented workflows as well as treatment response analyses.
In particular, we focus on the following topics:
1. Image-based patient and motion modeling,
2. Mathematical Methods to optimize physical and biological treatment parameters and dose distributions,
3. Treatment Outcome Analysis & Prediction (Dosiomics & Radiomics),
4. Analysis of molecular and imaging data from preclinical biological studies.
What infrastructure, programs and tools are used in your group?
• Latest generation of radiotherapy treatment machines for image-guided radiotherapy (Ethos, MRLinac, particle therapy)
• Access to data from imaging modalities: MRI, CT, PET
• IT Hardware: Workstations & Servers for AI workflows, OpenStack Computing Cloud
• IT Software: Commercial and Open-Source Treatment Planning and Image Processing Software
• Various series of seminars, lectures and courses
• Close collaboration with data scientists, medical physicists, radiation oncologists, radiologists and biologists
What could a participant of the HIDA Trainee Network learn in your group? How could he or she support you in your group?
Participants of the HIDA Trainee Network will get
• Access to strong interdisciplinary and translational work
• Insights into the latest radiotherapy and imaging developments
• In touch with applications of machine learning to medical (radiotherapy and imaging) data
Candidates could support in data analysis, development of new AI methods and bringing new ideas by translating solutions from a different research field to medical physics.
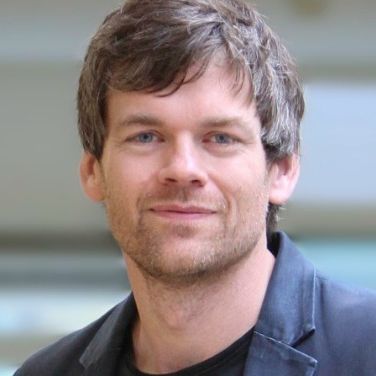
Klaus Maier-Hein
Klaus Maier-Hein - DKFZ
German Cancer Research Center (DKFZ) - Division of Medical Image Computing
Short summary of your group's research: The Division of Medical Image Computing (MIC) pioneers research in machine learning and information processing in the context of image data analytics. We pursue cutting-edge developments at the core of computer science, with applications in but also beyond medicine. We are particularly interested in techniques for semantic segmentation and object detection as well as in unsupervised learning and probabilistic modelling.
What infrastructure, programs and tools are used in your group?
- Python Software Framework / Pytorch / Phabricator
- A GPU cluster at DKFZ tailored to specific needs, ranging from nodes with RTX 2080ti all the way up to Nvidia’s DGX systems
- The DKFZ has an Openstack cluster in which you can instantiate virtual machines for CPU heavy workloads.
What could a participant of the HIDA Trainee Network learn in your group? How could he or she support you in your group? At MIC we pursue cutting-edge developments at the core of computer science, with applications in but also beyond medicine. We believe a sophisticated research software system and infrastructure are key for methodological excellence, for example to facilitate highly scalable data analysis in a federated setting. In cooperation with numerous (clinical) partners, we work on the direct translation of the latest machine learning advances into relevant clinical applications. Depending on the specific interests of the participant of the HIDA training exchange many interesting and challenging aspects could be addressed. Please find a list of ongoing research project in the department on our website in the "research" section.
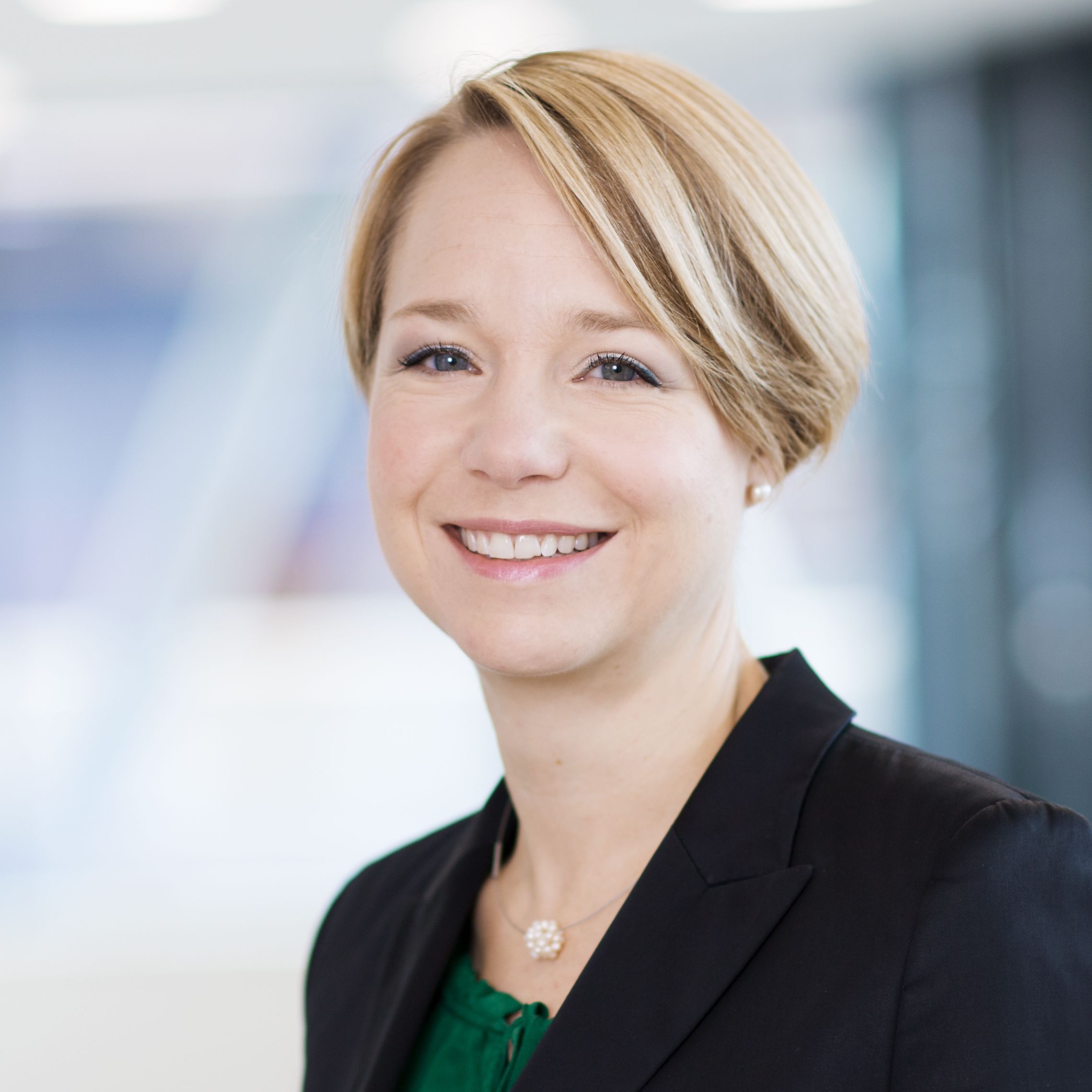
Stella Autenrieth
Stella Autenrieth - DKFZ
German Cancer Research Center (DKFZ) - Dendritic Cells in Infection and Cancer
Short summary of your group's research: Our research group is interested in elucidating the role of different DC subpopulations, their development and basic function in immune activation by bacterial infections, inflammation, and cancer to improve the basic understanding of DC function in disease but also the potential of DCs as targets for therapeutic intervention. Another focus of our research group is the phenotyping of immune cells using spectral flow cytometry. This allows the detailed characterization of all immune cells in the blood and tissues of patients before and during therapy with the goal of identifying cell populations or biomarkers that can predict therapy response.
What infrastructure, programs and tools are used in your group? The main techniques are spectral flow cytometry and scRNA sequencing. With spectral flow cytometry about 40 proteins that are expressed on or in cells can currently be determined simultaneously at the single cell level. We use tools like OMIQ for flow cytometry data and R for scRNA data analysis.
What could a participant of the HIDA Trainee Network learn in your group? How could he or she support you in your group? We offer insights into single cell analysis of immune cells especially how bacterial infections modulate the dendritic cell development. We hope to get support regarding the molecular mechanisms responsible for the infection-induced impaired dendritic cell development by analyzing our scRNA seq & spectral flow cytometry data.
Deutsches Elektronen-Synchrotron - DESY
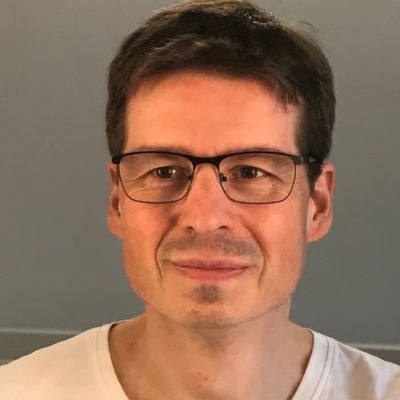
Jochen Küpper
Jochen Küpper - DESY
Deutsches Elektronen-Synchrotron DESY - Controlled Molecule Imaging (Photon Science)
Short summary of your group's research: We develop innovative methods to obtain full control over large molecules and nanoparticles. These methods and the created controlled samples are exploited in fundamental physics and chemistry studies to unravel the underlying mechanisms of chemistry and biology by watching molecules at work. This is coupled with advanced experimental control and data acquisition software, including on-the-fly data reduction, as well as theoretical and computational physics – both utilizing machine learning approaches for improved performance.
What infrastructure, programs and tools are used in your group?
Have a look on our website.
What could a participant of the HIDA Trainee Network learn in your group? How could he or she support you in your group? Machine learning in theoretical physics and quantum chemistry and in real-time data reduction – benefit from current expertise and improve our approaches.
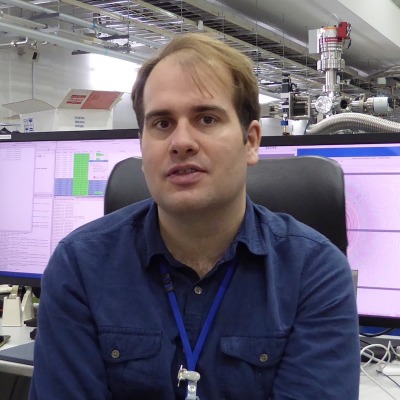
Thomas White
Thomas White - DESY
Deutsches Elektronen-Synchrotron DESY - Scientific Computing (Photon Science)
Short summary of your group's research: We are developing computational tools for processing large crystallographic datasets consisting of many hundreds of thousands of individual frames. Recently, we are moving towards real-time data processing, with the aim of making the data analysis step "disappear" - becoming part of the data acquisition process. Our main product embodying this work is the free and open source "CrystFEL" software package.
What infrastructure, programs and tools are used in your group?
Infrastructure: Gitlab, DESY "Maxwell" cluster
Tools: HDF5, SLURM, GSL, OpenCL, ZeroMQ, MessagePack, CMake, Meson, GTK and more
Languages: C/C++, Python, Lisp and others
What could a participant of the HIDA Trainee Network learn in your group? How could he or she support you in your group? As well as working with large-scale accelerator-based photon facilities (PETRA III and European XFEL, plus other facilities worldwide), you would gain insight into developing a widely-used (>100 worldwide users) domain-specific software package. For instance, how to balance the implementation of cutting-edge scientific methods with other considerations such as stability and reliability. During your stay, you could contribute (for example) by experimenting with a new way of processing data, speeding up existing processing methods, or implementing one of the many requested features in CrystFEL.
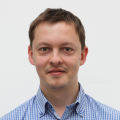
Andrey Yachmenev
Andrey Yachmenev - DESY
Deutsches Elektronen-Synchrotron DESY - Controlled Molecule Imaging group in Center for Free-Electron Laser Science
Short summary of your group's research: Our group is working on the development of computational techniques and high-accuracy simulations to improve our knowledge of the nuclear dynamics and spectra of molecules interacting with external electromagnetic fields. We're also working on ways to simulate ultrafast imaging experiments like photo-electron circular dichroism and laser-induced electron diffraction. We have recently begun to investigate various machine learning tools in applications to our problems, which range from solving differential equations to developing effective representations for molecular potential energy surfaces, as well as analyzing and inverting experimental photoelectron imaging data.
What infrastructure, programs and tools are used in your group? HPC, scientific computing and machine learning tools implemented in Python and Julia.
What could a participant of the HIDA Trainee Network learn in your group? How could he or she support you in your group? You will develop or expand your grasp of many theoretical, computational, and experimental aspects of quantum molecular dynamics, high-resolution spectroscopy, photoelectron imaging, and machine learning applications to these challenges. You may learn more about software development techniques by contributing into development of open source software for molecular dynamics simulations.
We offer highly exploratory projects in development of neural networks for efficient solution of Schrödinger equation for nuclear motion and photo-electron dynamics, mathematical analysis of these approaches, and analysis of experimental photoelectron imaging data. We welcome applicants from a variety of disciplines with some background in machine learning and can offer and adapt a range of possible projects.
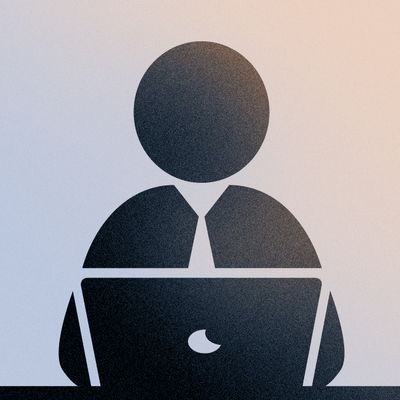
Marek Kowalski
Marek Kowalski - Desy
Deutsches Elektronen-Synchrotron DESY - Astroparticle Physics, Neutrino Astronomy & Cosmology
Short summary of your group's research:
We are performing high energy neutrino astronomy using the observatories IceCube and RNO-G, as well as as the future IceCube-Gen2. Furthermore, we are working on optical surveys such as the Zwicky Transient Facility and the UV satellite ULTRASAT. The joint exploitation of the data enables new routes in multimessenger astrophysics. A key tool to combine the multimessenger data is AMPEL, a software framework developed by my research group to analyses realtime time series.
What infrastructure, programs and tools are used in your group?
AMPEL, IceCube, ZTF
What could a guest researcher learn in your group? How could he or she support you in your group?
Inference of galaxy properties and properties of astronomical transients using machine learning applications on multi band time series and catalog data.
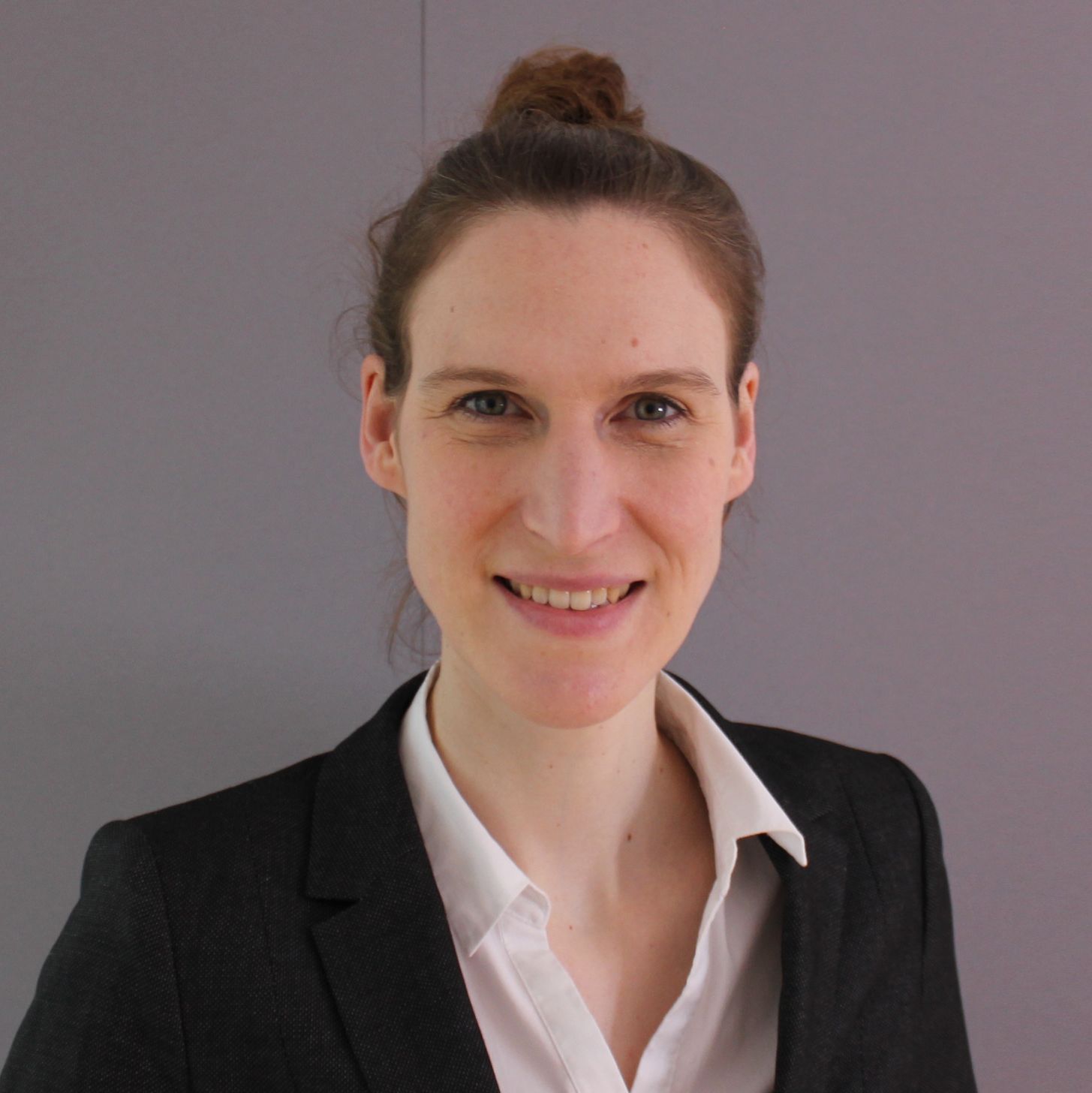
Annika Eichler
Annika Eichler - DESY
Deutsches Elektronen-Synchrotron DESY - Machine beam control (MSK)
Short summary of your group's research:
Intelligent Process Controls (IPC) is a subgroup of the Machine Beam Controls (MSK) group at DESY, pushing forward innovative research for the autonomous operation of particle accelerators at the interface of machine learning, control. For this using reinforcement learning and other cutting-edge optimization techniques. IPC is also engaged in developing advanced feedbacks and enhancing fault diagnosis and anomaly detection through machine learning.
What infrastructure, programs and tools are used in your group?
The participant will have access to high-performance computing infrastructure at DESY. As programming languages, we mainly use Python.
What could a guest researcher learn in your group? How could he or she support you in your group?
As an interdisciplinary research team of experts from control theory, computer science and physics, a participant of the HIDA Trainee Network can gain experience in different directions and support many different applied projects. Here data mining projects as for anomaly detection are possible but also control and optimization problems. For the latter, we strongly encourage the Trainee to participate in shifts and applying the developed methods to the accelerator.
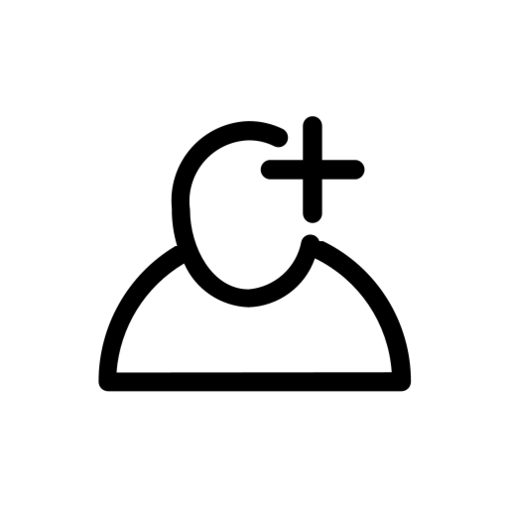
Gastgeber werden
Helmholtz Gastgeber werden
Wenn Sie daran interessiert sind, eine Nachwuchswissenschaftlerin oder einen Nachwuchswissenschaftler aus einem anderen Helmholtz-Zentrum aufzunehmen und Einblick in Ihre Forschung zu gewähren, wenden Sie sich bitte an hida@helmholtz.de
German Aerospace Center - DLR
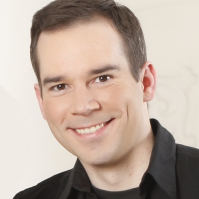
Ronny Hänsch
Ronny Hänsch - DLR
German Aerospace Center (DLR) - SAR Technology department of the Microwave and Radar Institute
Short summary of your group's research: The SAR Technology department addresses everything from building up the sensor (currently the multi-frequency fully polarimetric F-SAR), planning and executing measurement campaigns, SAR processing (i.e. focusing, geocoding, image enhancement), up to the analysis of the final image product. The Machine Learning team mainly focuses on the last part, i.e. using machine learning to derive higher level information (e.g. semantic maps, geo-/bio-physical parameters) from the SAR images. Topics of relevance are single-image superresolution, denoising (despeckling), ensemble learning, deep learning, image synthesis, semantic segmentation, semi-/weakly-/self-supervised learning, XAI, out-of-distribution/anomaly detection, etc.
What infrastructure, programs and tools are used in your group? Programming is mostly done in python or C++; deep learning developments either in PyTorch or Tensorflow. We do have access to GPU servers.
What could a participant of the HIDA Trainee Network learn in your group? How could he or she support you in your group? We offer insights in all aspects of SAR, including hardware, focussing, processing, and analysis, combined with a profound knowledge in machine/deep learning, and applied to interesting and highly relevant applications (e.g. forest monitoring, urban growth, development of glaciers, etc.). We are looking for people with knowledge in machine/deep learning and/or image processing to apply their skills to a very exciting and powerful type of image data, i.e. SAR, or to use machine learning and SAR to solve relevant tasks in emerging applications.
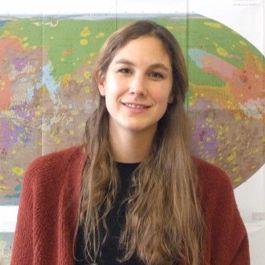
Kristin Rammelkamp
Kristin Rammelkamp - DLR
German Aerospace Center (DLR) - Institute of Optical Sensor Systems, Department of Terahertz and Laser Spectroscopy, Berlin-Adlershof
Short summary of your group's research: In the DLR junior research group “Machine learning for planetary in-situ spectroscopic data”, we investigate data measured in the laboratory but also on Mars by instruments like ChemCam (NASA’s Mars Science Laboratory). We train and evaluate supervised algorithms for the prediction of elemental abundances in rocks and soils, and for the classification of mineralogical classes. Additionally, we use unsupervised methods for pattern recognition in large spectroscopic datasets and aim also for physics informed machine learning based simulations of spectra from geological targets.
What infrastructure, programs and tools are used in your group? For the machine learning part of our research, we work with frameworks like PyTorch, Sklearn etc provided in python. We have laboratory instruments that can be used to measure spectroscopic data, also in simulated martian and vacuum atmospheric conditions. The spectroscopic techniques we are mainly working with are laser-induced breakdown spectroscopy (LIBS) and Raman spectroscopy.
What could a participant of the HIDA Trainee Network learn in your group? How could he or she support you in your group? You can learn several aspects in our group starting with the basics of the spectroscopic methods and the nature of the data, especially with regard to planetary in-situ exploration. Regarding machine learning, you can learn how to optimize models trained with spectroscopic data for different purposes, such as regression and classification. Furthermore, you can benefit from our experience in real planetary mission involvements. We are looking forward to learning from your machine learning experience for other applications and how this could be adapted to spectroscopic data. In particular, we are interested in explainability of machine learning models in order to better understand the predictions and performance of them and in ways to include physical knowledge in the training process.
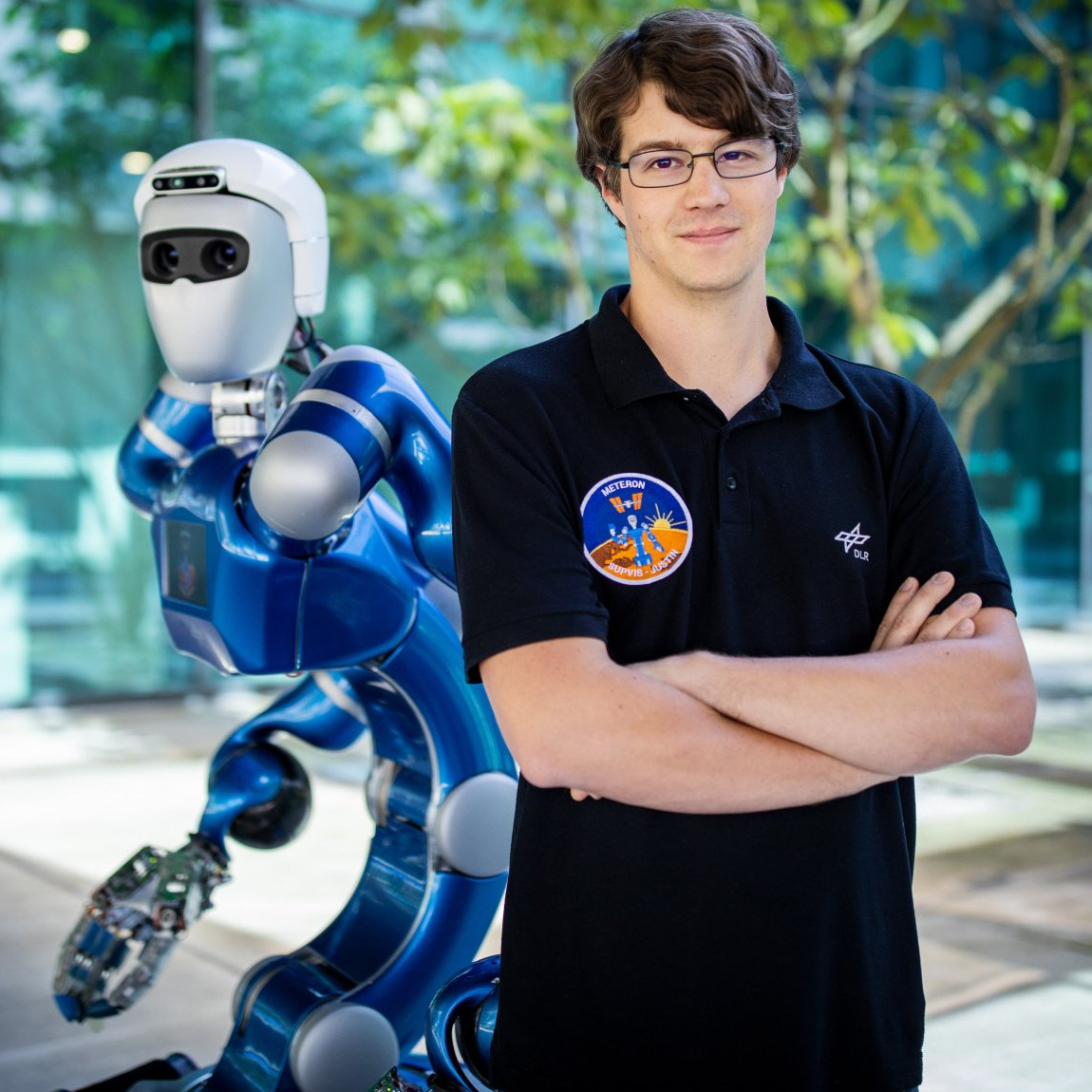
Daniel Leidner
Daniel Leidner - DLR
German Aerospace Center (DLR) - Institute of Robotics and Mechatronics, Department of Autonomy and Teleoperation
Short summary of your group's research: The Institute of Robotics and Mechatronics, Department of Autonomy and Teleoperation explores all aspects of robot autonomy. This includes autonomous operation with AI-based approaches to task and motion planning, as well as teleoperation modalities for remote operation under human supervision with intuitive interfaces. All of this is embedded in fault-tolerant autonomy architectures that enable resilient decision making, inspection, monitoring, and error handling. The design of software components for embedded and distributed computing platforms enables the use of these methods for space applications under hard real-time conditions.
What infrastructure, programs and tools are used in your group? To increase the autonomy of robotic systems we investigate techniques to generate robot programs from logical task descriptions (e.g. PDDL). The robot Rollin Justin (see picture) is one of several demonstration platforms for these tasks. The software stack of the robot is based on different modules developed in Python, C++, and Simulink. We develop model-based techniques for decision making leveraging real-world robot telemetry as well as semantic information inferred through physics-simulations (e.g. Gazebo). To control the robot (e.g. from aboard the ISS) intuitive control modalities are developed such as shared control and supervised autonomy.
What could a participant of the HIDA Trainee Network learn in your group? How could he or she support you in your group? We offer visiting researchers the opportunity to participate in the development of software for some of the most advanced robots in the world. We utilize advanced programming techniques for use under real-time and non-real-time conditions. This includes integrating complex control strategies for compliant robots with AI-based reasoning methods, developing physics-based reasoning for error handling, and developing multi-modal robot interfaces in the context of astronaut-robot collaboration. Visiting researchers will learn to apply an agile, concurrent software engineering process to develop software components for space-qualified robots.
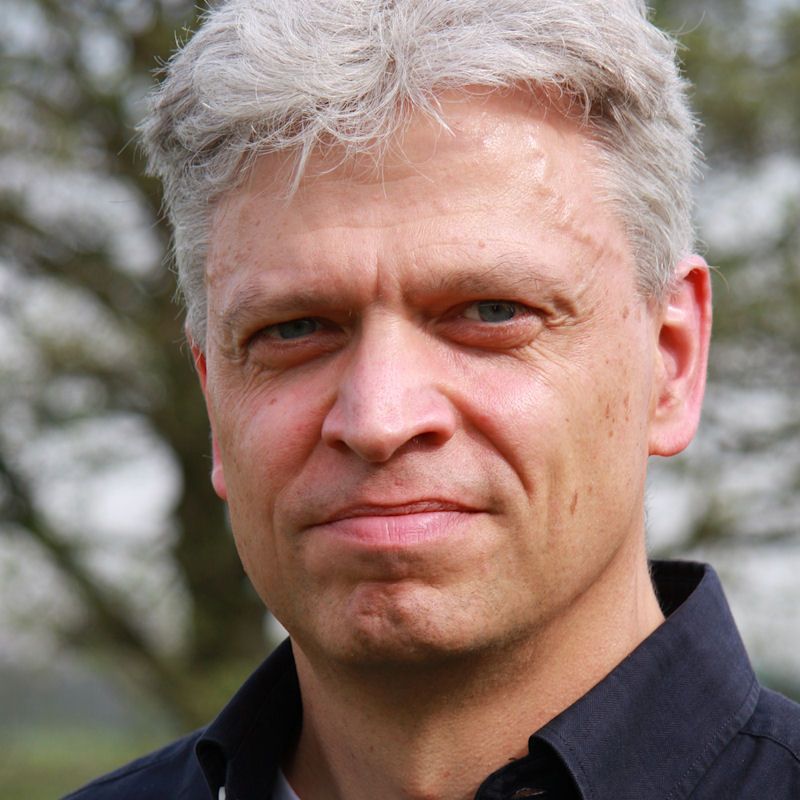
Andreas Gerndt
Andreas Gerndt - DLR
German Aerospace Center (DLR) - Visual Computing and Engineering Department
Short summary of your group's research: The mission of the DLR Institute for Software Technology is research and development in software engineering technologies, and the incorporation of these technologies into DLR software projects. The department of Software for Space Systems and Interactive Visualization investigates and develops methods for robust and reusable software solutions for the design and operation of space missions as well as for interactive visualization applications.
What infrastructure, programs and tools are used in your group? Besides modern computer systems, we are using well-equiped laboratories for flight software integration into onboard sytems, for the investigation of immersive software in virtual environments, and for evaluation of software solutions into mixed and extended reality. This includes large immersive virtual reality powerwall displays, multi-pipe multi-touch display walls, and fully embedded systems development equipement. Additionally, we are operating a software laboratory for highly agile joint software development sessions. All systems have access to institute server for high-performance computing, cluster solution development, and high-end visualization approaches.
What could a participant of the HIDA Trainee Network learn in your group? How could he or she support you in your group? We are looking for excellent scientists for all activities in the research groups of the department: robust and resilient onboard software, formal verification of spacecraft design, digital transformation in the space domain, in-situ data processing and visualization on high-performance computers, topology-based data analysis for large-scale datasets, visual data analytics, virtual reality, mixed reality, extended reality, human factors and user studies, applications in DLR's domain like space, aeronautics, transportation, climate change, and more.
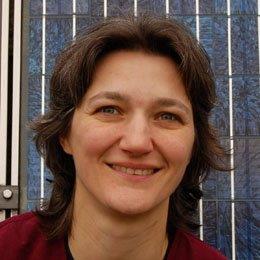
Annette Hammer
Annette Hammer - DLR
German Aerospace Center (DLR) - Institute of Networked Energy Systems, Energy system analysis
Three-sentence summary of your group's research
The Energy Meteorology Group has a long experience in solar forecasting, pv-modelling and satellite retrieval. We operate a sensor and camera network in North West Germany.
What infrastructure, programs and tools are used in your group?
python and pytorch
What could a guest researcher learn in your group? How could he or she support you in your group?
You will use meteorological data, camera images, satellite images and photovoltic power measurements. You will learn, how we model PV power from these input data. You will support us in forecasting PV-power-production for the next minutes and hours form these input data.
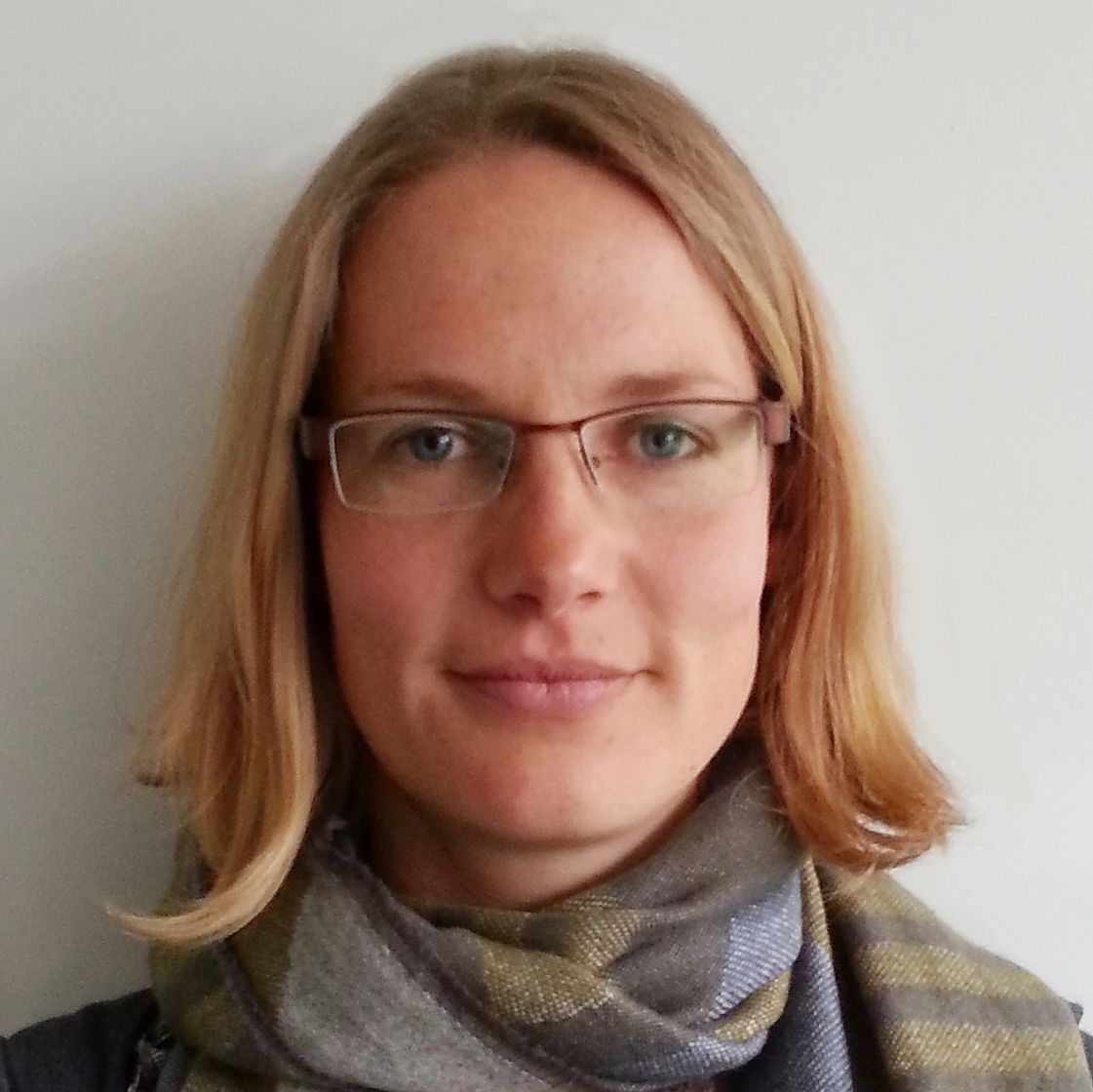
Anne Papenfuß
Anne Papenfuß - DLR
German Aerospace Center (DLR) - Institute of Flight Guidance - Department of Human Factors - Data Science for Human Factors
Three-sentence summary of your group's research: The Human Factors department supports the development of advanced concepts and assistance systems for pilots, air traffic controllers and control center personnel in terms of human-centered automation. It is cross-sectionally manifested in the Institute of Flight Guidance and supports the specialist departments in the early concept phases. An interdisciplinary team of engineers, psychologists and computer scientists plans and manages validations with operators in real-time simulation, field trials and flight tests. In general, it is important to take equal account of improving the individual human-machine cooperation and the cooperation between the on-board and ground-based systems and the people involved in them.
What infrastructure, programs and tools are used in your group? - Virtual environments, High-fidelity simulators of Air Traffic Control working positions, aircraft cockpits, VR-glasses
- Physiological measurement tools like EEG, fNIRS, EKG
- Eye tracking
- Speech recognition
What could a guest researcher learn in your group? How could he or she support you in your group? - A participant could learn about data representing human factors in automated systems, as well as how data are collected in controlled experimental designs and used for informed decisions about system design.
- A participant can furthermore learn about research topics on human-machine interaction and cooperation in the field of aviation.
- Partly automated data processing/data analysis like detection of patterns and anomalies in heterogenous data (e.g. by AI)
- Development of analysis and visualization concepts
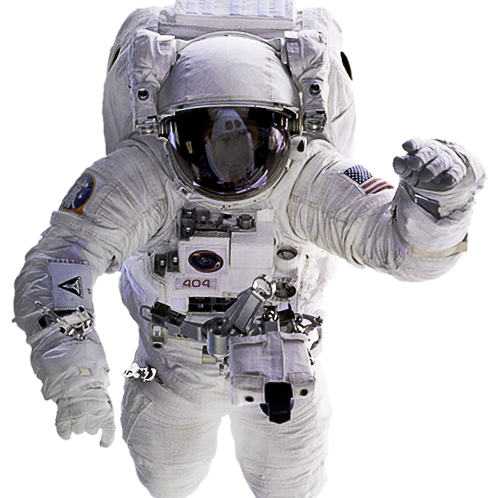
Claudia Stern
Claudia Stern - DLR
German Aerospace Center (DLR) - Clinical Aerospace Medicine (LRM)
Three-sentence summary of your group's research: Long duration human spaceflight missions create medical support challenges for eye changes, which can occur in nearly two-thirds of astronauts. To address these challenges, we are developing artificial intelligence applications to support crew members in monitoring their eyes. These applications have the potential to be used for crew medical support aboard the International Space Station, and beyond.
What infrastructure, programs and tools are used in your group? For the machine learning component of our research, Python, convolutional neural networks, Tensorflow, GPU servers, and computer vision tools are used to conduct our analyses. To collect the raw image and video data used in our analysis, we use ophthalmology imaging tools (e.g., fundoscopy, optical coherence tomography (OCT), etc.) commonly used in clinical practice worldwide. You would require access to a development environment (e.g., VSCode, Pycharm, etc.), understanding of and adherence to data security and ethics standards, and a modern smartphone/tablet.
What could a guest researcher learn in your group? How could he or she support you in your group? In our group, you could learn about the eye changes astronauts experience during long duration spaceflight, especially with regard to the retina. You could advance your machine learning skills to optimize regression, classification, and object detection models trained with our image and video data. In particular, we are interested in and open to new ways to improve the predictions and performance of our networks and models. By joining our group, you could benefit from our experience in aerospace medicine, human spaceflight, and International Space Station research, and we would benefit from your expertise in computer vision for human medical data. We would look forward to the possibility of working together.
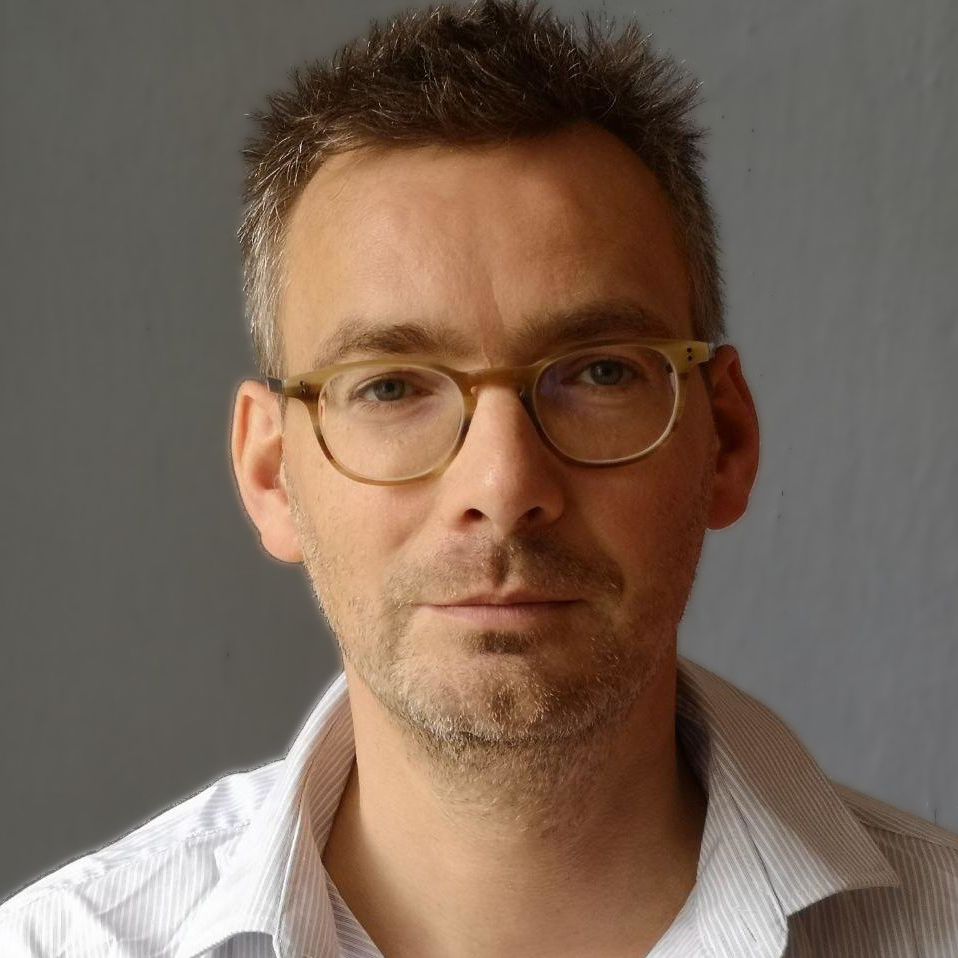
Peter Jung
Peter Jung - DLR
German Aerospace Center (DLR) - Department Real-Time Data Processing, Sensor-AI Group
Three-sentence summary of your group's research: The new SensorAI group in the OS-EDP department will focus on challenging data science and sensing problems related to sensors and optical instruments and imaging devices. This includes the design, development and evaluation of data aggregation methods, calibration and recovery algorithms, and high-dimensional data analysis. Recent theoretical advances in sensing (compressed sensing, low-rank recovery and super-resolution) and AI methods (from deep learning to neural-augmented/unfolding algorithms, PINNs and more recent AI architectures) will be used to address inverse problems in signal processing and physics.
What could a guest researcher learn in your group? How could he or she support you in your group?
What could a guest researcher learn in your group? How could he or she support you in your group?:
Guest researchers are invited to cooperate on topics with the SensorAI group in the EDP department. This includes (but is not limited to):
1) AI for solving inverse problems in signal processing, physics and data science.
2) deep learning, neurally-augmented/unfolding algorithms
3) uncertainty quantification
4) Quantum computing
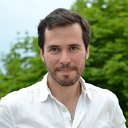
Andrés Camero Unzueta
Andrés Camero Unzueta - DLR
German Aerospace Center (DLR) - EO Data Science
Three-sentence summary of your group's research: The Helmholtz AI consultant team @ DLR provides expertise from Earth observation, robotics and computer vision and an HPC/HPDA support unit.
The team covers a comprehensive package of AI-related services. It is supposed to support both projects within LRV and cooperative projects with other Helmholtz centers. On the other hand, the EO Data Science Department develop AI methods for Earth observation data to tackle societal grand challenges.
What infrastructure, programs and tools are used in your group? Coding is mainly done in Python and C/C++, using the most popular optimization and ML/DL frameworks. We have access to GPU servers.
What could a guest researcher learn in your group? How could he or she support you in your group? We offer a broad range of AI expertise, with a strong focus on Earth observation applications. We are looking for people with knowledge in machine learning, optimization and/or image processing to apply their skills to tackle societal grand challenges using Earth observation data.
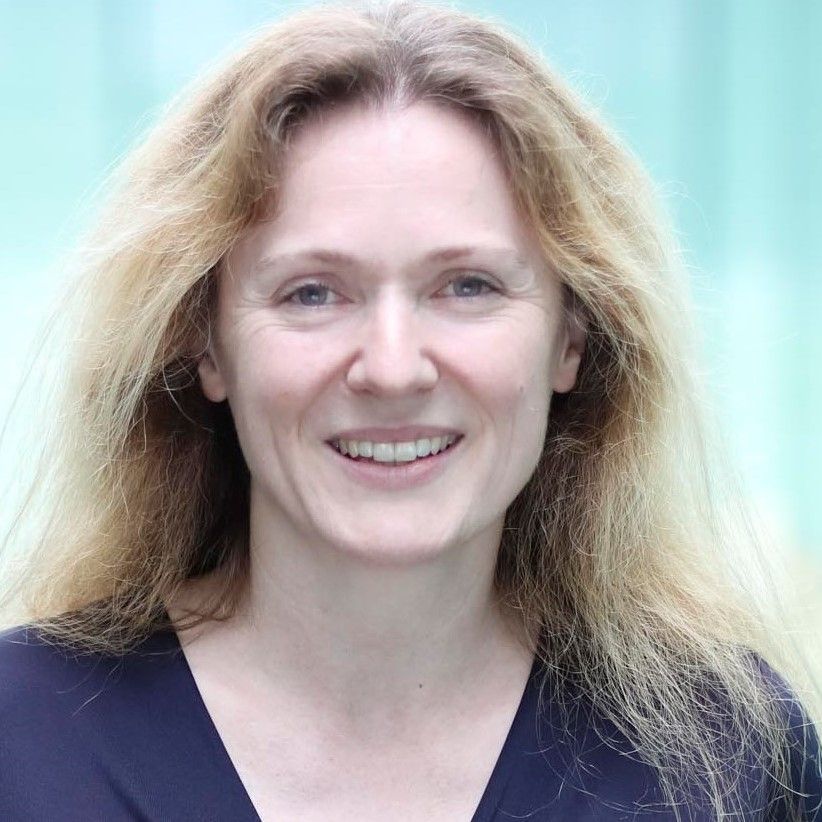
Eva-Maria Elmenhorst
Eva-Maria Elmenhorst - DLR
German Aerospace Center (DLR) - Institute of Aerospace Medicine, Department Sleep and Human Factors Research, Division Performance and Sleep
Three-sentence summary of your group's research: We study the homeostatic and circadian processes that regulate the quality, duration, and timing of sleep, as well as cognitive performance. Data are collected both in the laboratory and in the operational environment, e.g. the cockpit. We study factors like acute and chronic sleep loss, workload, stimulants, circadian misalignment, and inter-individual differences in the vulnerability to sleep loss. We are also interested in cognitive and operational performance in extreme environments, e.g. space, and the influence of a multitude of stressors, such as hypoxia, hypercapnia, simulated microgravity (head down tilt bedrest), or microgravity during parabola flights.
What infrastructure, programs and tools are used in your group? During our studies, we gathered a rich multitude of data under extreme conditions (fatigue, hypoxia, hypercapnia, microgravity): behavioral responses in cognitive tasks and simulations, eye tracking data, physiological measurements (polysomnography, ECG, EEG), actigraphy, fMRI and PET.
What could a guest researcher learn in your group? How could he or she support you in your group? Guest researchers can learn sleep measuring techniques (including polysomnography), circadian analysis techniques (including mathematical modeling of circadian and sleep-wake regulation), eye-tracking techniques, and be part of large laboratory (e.g., bedrest studies) and field studies (e.g., assessing fatigue and cognitive performance in pilots). We welcome expertise in bioengineering, psychology, medicine, biology, and related disciplines, with a special emphasis on skills in advanced statistical analyses and machine learning.
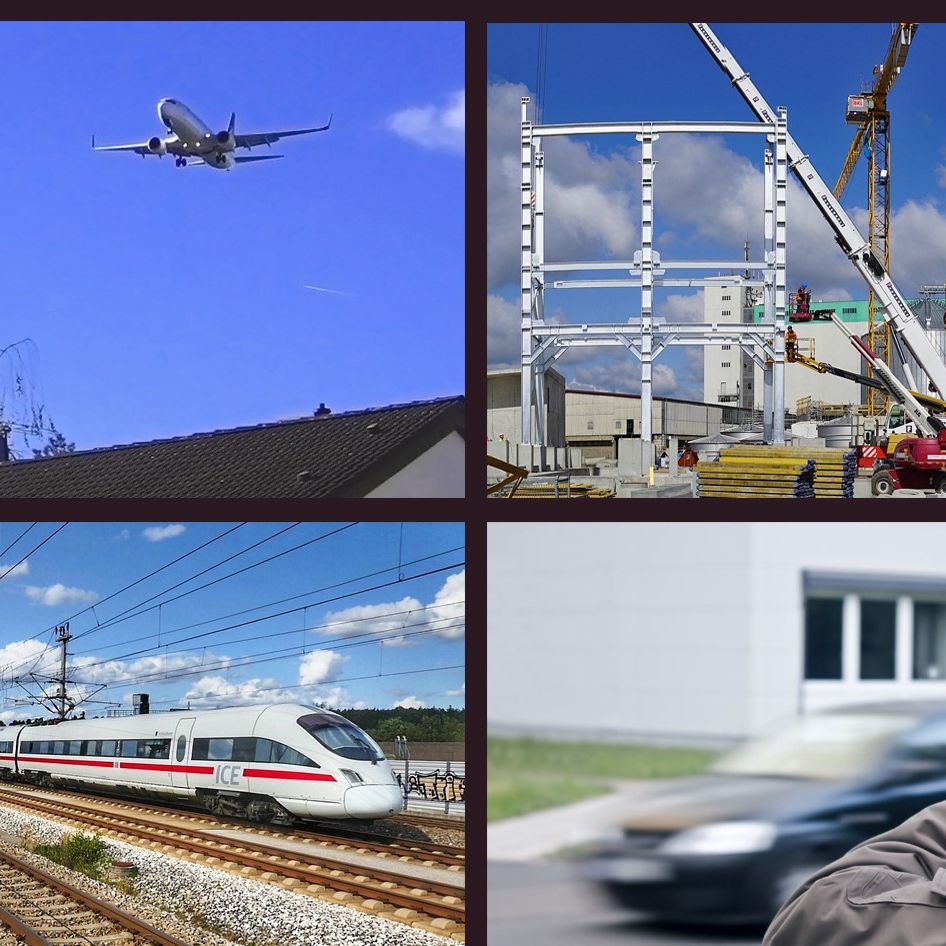
Susanne Bartels
Susanne Bartels - DLR
German Aerospace Center (DLR) - Institute of Aerospace Medicine, Sleep and Human Factors Research - Noise Effects Research
Three-sentence summary of your group's research: We investigate how air, rail and road traffic noise affect sleep, cognitive performance and annoyance. We conduct both laboratory studies as well as field studies in residents affected by transportation noise and derive exposure-response relationships for awakening probabilities and annoyance that are used to develop protection concepts for the affected population. Noise effects are being studied in healthy adults as well as in vulnerable individuals, e.g. children and elderly people.
What infrastructure, programs and tools are used in your group? To collect data on sleep quality and cardiovascular parameter, we apply polysomnography incl. EEG, ECG, pulse oximetry and actigraphy. Noise exposure data are obtained via acoustic measurements (e.g. by means of class-1 sound level meters). Survey data are obtained via postal or online surveys using, for instance, LimeSurvey. For data management and analysis, we use e.g. SOMNOmedics DOMINO, Matlab, R-Studio and SPSS as well as self-programmed and customized tools based on Matlab and LabVIEW.
What could a guest researcher learn in your group? How could he or she support you in your group? By joining our group, you could learn about the manifold effects of environmental noise on human health and their underlying mechanisms. You would benefit from our expertise in data collection in controlled experimental designs in the laboratory and the field as well as in surveys. Furthermore, you could increase your knowledge in sleep measurement techniques, e.g. polysomnography, and apply your skills (incl. machine learning skills) in the analysis of cardiovascular reactions to noise (e.g. regarding heart rate variability, pulse transit time) and/or noise-induced reactions in sleep (e.g. vegetative-motoric reactions, identification of EEG arousals).
Expertise in bioengineering, psychology, medicine, biology, and related disciplines, with a special emphasis on skills in advanced statistical analyses, data visualization and machine learning are very welcome. We are looking forward to an open exchange of ideas and methods for the in-depth investigation of noise impacts on humans.
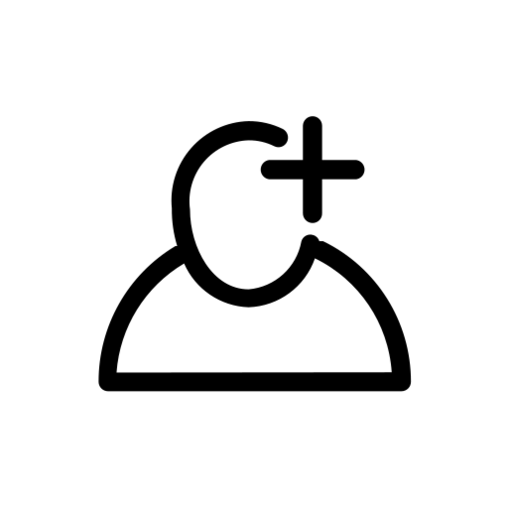
Gastgeber werden
Helmholtz Gastgeber werden
Wenn Sie daran interessiert sind, eine Nachwuchswissenschaftlerin oder einen Nachwuchswissenschaftler aus einem anderen Helmholtz-Zentrum aufzunehmen und Einblick in Ihre Forschung zu gewähren, wenden Sie sich bitte an hida@helmholtz.de
GFZ Helmholtz Centre for Geosciences
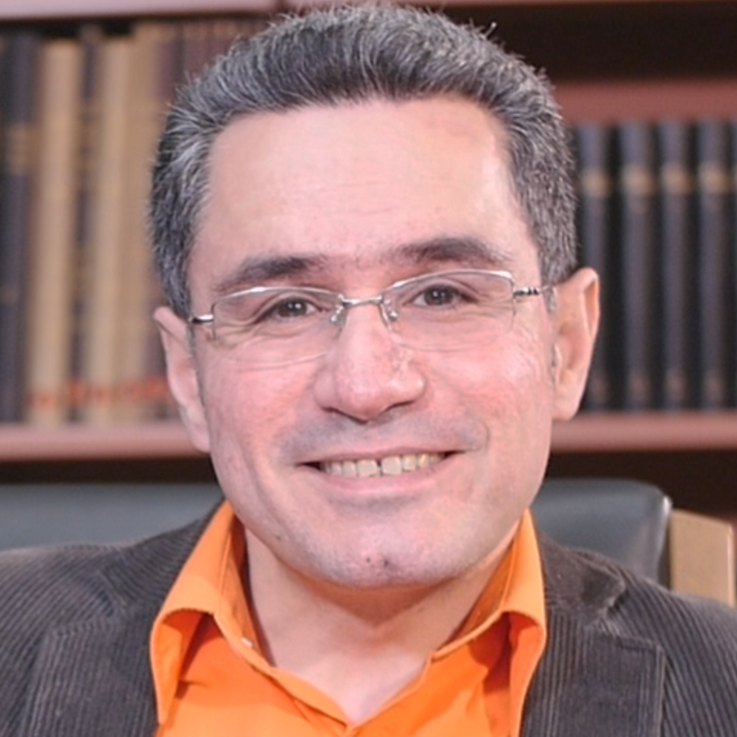
Mahdi Motagh
Mahdi Motagh - GFZ
GFZ Helmholtz Centre for Geosciences - Geodesy
Three-sentence summary of your group's research: My research interests focus on the use of radar remote sensing data to investigate processes related to various types of geological phenomena and engineering applications, such as active tectonics, landslides, floods, groundwater extraction/injection, underground mining, glacier motion and ice mass change, dam stability, and anthropogenic activities in urban areas.
What could a guest researcher learn in your group? How could he or she support you in your group? Integration of SAR data with machine learning approaches to address natural hazards
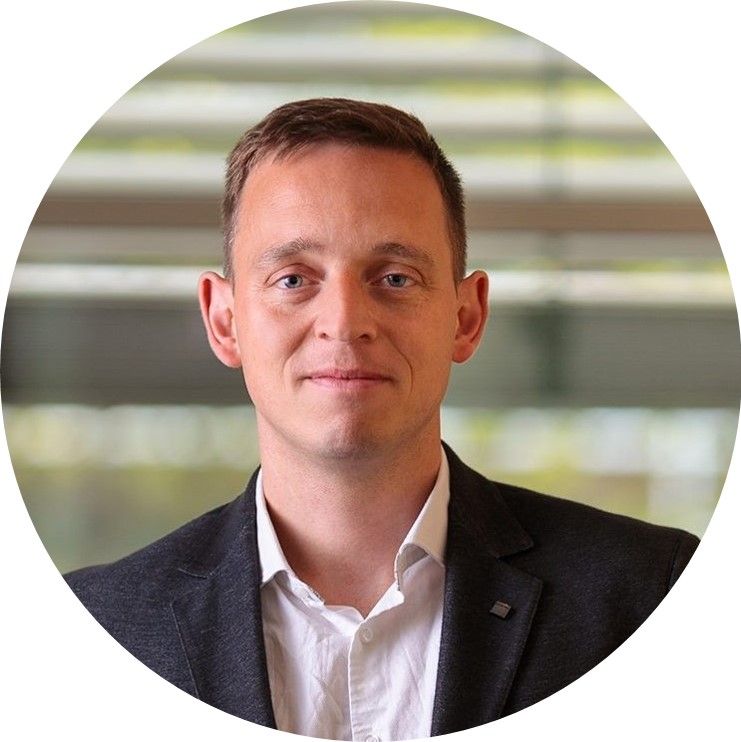
Sven Fuchs
Sven Fuchs - GFZ
GFZ Helmholtz Centre for Geosciences - Hydrothermal Systems
Short summary of your group's research: Our working group explores the Earth’s thermal field and geothermal resources, studies involved processes, quantifies their relevance, and provides knowledge on its behavior over time and across scales. The group is aimed at identifying priority targets for different geoenergy utilizations. With our work, we contribute to the transformation of the conventional energy system and to the reduction of CO2 emission.
With an applied focus, we elaborate exploration strategies to advance the successful development of geoenergy utilization in urban areas, where conventional surface exploration methods are often not applicable. We develop advanced methodologies for cross-scale characterization and for a better scale-dependent parameterization of the subsurface for risk reduction. A key concept is to combine geological expertise with a multi-methodological approach to establish adequate and reliable conceptual subsurface models. Fields of application include the sustainable provision of geothermal energy or the successful application of (thermal) storage systems.
With a more fundamental perspective, we investigate thermal processes in the crust and analyze the thermal field (including heat flow), and provide boundary conditions for multi-process integrated geodynamic models. With the integration of multidisciplinary observation data, we improve the understanding of the present thermal state and involved processes in subsurface thermal geosystems: from local to global views, from rock to lithospheric scales and across time domains.
What could a participant of the HIDA Trainee Network learn in your group? How could he or she support you in your group? We welcome all researchers with interest in geothermal field exploration, numerical methods and related data science. A guest researcher could learn about thermal field modeling approaches and their applications. Possible joint projects could involve processing and mapping of regional to global heat flow data, conducting analysis of observational data for mapping applications.
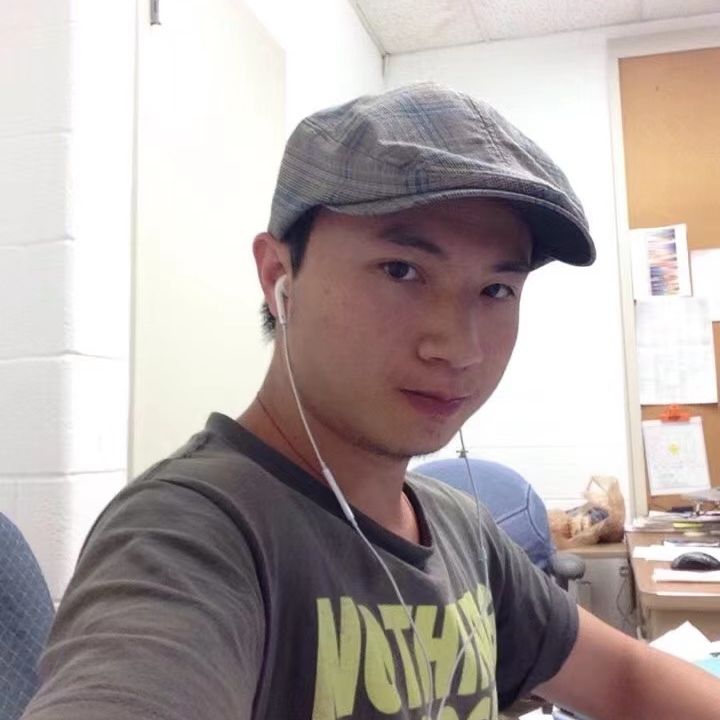
Hui Tang
Hui Tang - GFZ
GFZ Helmholtz Centre for Geosciences - Earth Surface Process Modelling/Hazards and Surface Processes Research Group
Short summary of your group's research: Our research group studies hazards and related surface processes across a wide range of environments, from mountain regions to coastal areas and even deep oceans, over different time scales. Our work has wide topics ranging from earthquake and tsunami, storm and hurricane, landslide and debris flow, to flood and paleo-flood. We use various tools and methods, including field surveys, remote sensing, environmental seismology methods, processes-based modelling, and data science methods, including machine learning to understand the physical processes behind all these natural hazards.
What infrastructure, programs and tools are used in your group? We use the High-Performance Computing infrastructure, including GPU and CPU clusters from our section, GFZ or Helmholtz association, as our main computation resource. For programming languages, we have used different mainstream languages, including Fortran, C, C++, Python, R, Matlab, and Julia, depending on the project. Regarding the data science model, we develop and use open-source platforms such as Scikit-learn, Tensorflow, and PyTorch.
What could a participant of the HIDA Trainee Network learn in your group? How could he or she support you in your group? As an interdisciplinary and diverse research group, we can offer many opportunities for HIDA Trainee Network participants with various interesting projects. There are two main types of projects: method-development-oriented projects and application-oriented projects. For a method-oriented, the participant could learn and develop physics-based machine learning methods for solving partial differential equations or investigating physics. An application-oriented participant could work on earth science or hazard-related research in the project using methods borrowing from computer vision, natural language processing, or signal processing. The participant will involve and support us in developing open-source data science model toolkits for the earth science and hazard science communities. Detailed project descriptions can be found on our group homepage. Feel free to contact us if you have any questions about the projects or bring your own ideas. We specifically welcome applicants from under-presented groups and third-world countries.
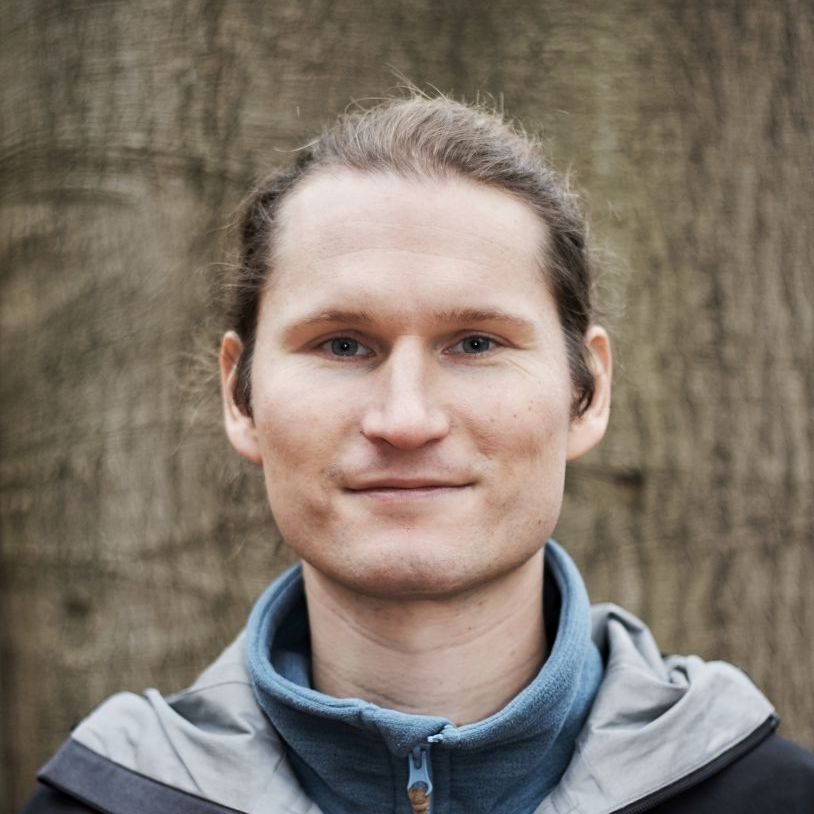
Benjamin Brede
Benjamin Brede - GFZ
GFZ Helmholtz Centre for Geosciences - Section 1.4 Remote Sensing and Geoinformatics
Three-sentence summary of your group's research: We link fine scale observations of vegetation to global satellite observations in order to calibrate and validate EO products. For this we use proximal remote sensing like Terrestrial and UAV laser scanning, radiative transfer models and machine learning.
What infrastructure, programs and tools are used in your group? UAV and terrestrial laser scanning, hyperspectral imaging.
What could a guest researcher learn in your group? How could he or she support you in your group? A researcher can learn to design field experiments that aim to link local to global observations of vegetation. A researcher can support the group with novel ideas on using AI and machine learning, in particular to apply on point cloud data.
Forschungszentrum Jülich - FZJ
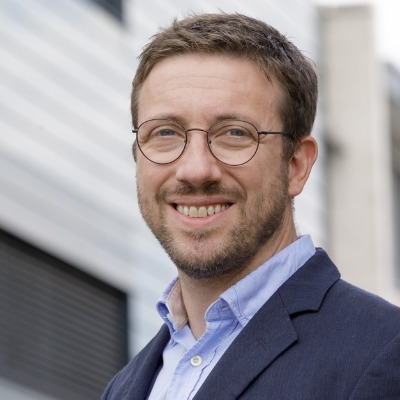
Timo Dickscheid
Timo Dickscheid - FZ Jülich
Jülich Research Centre (FZJ) - Institute of Neuroscience and Medicine Structural and functional organisation of the brain (INM-1) - Big Data Analytics Group (BDA)
Short summary of your group's research: The Big Data Analytics group in Jülich develops software and methodology to build a microscopic resolution 3D model of the human brain, which includes detailed information about distributions of neuronal cells and nerve fibers, and microstructurally defined 3D maps of brain areas. This requires microscopic imaging and analysis of large quantities of histological brain sections at high throughput, leading to image datasets at the Petabyte scale.
At the intersection of neuroinformatics, computer vision and artificial intelligence (AI), our research addresses
- Data and workflow management for high throughput microscopic imaging,
- Machine learning and computer vision algorithms for biomedical image analysis on high performance computers,
- Software development for structured access to large image datasets and interactive 3D exploration of high-resolution brain atlases over the web.
What infrastructure, programs and tools are used in your group? The trainee will be part of the local Helmholtz AI unit in Jülich. Our data management and image analysis workflows run on a distributed environment which includes Jülich’s modular high-performance computing systems. Image acquisition of whole-brain sections at micrometer resolution is performed on a high throughput microscopy facility. Outputs of our research are typically integrated with the human brain atlas hosted in the European research infrastructure EBRAINS, to which we contribute key developments. Most of our software is implemented in Python. Image analysis and machine learning approaches use common Deep Learning frameworks, typically PyTorch or Tensorflow.
What could a participant of the HIDA Trainee Network learn in your group? How could he or she support you in your group?
A trainee in our group can learn about
- biomedical computer vision, especially processing and analysis of high resolution image data using state of the art AI methods
- distributed workflow management and parallel processing of big image data on supercomputers
- neuroinformatics.
A trainee can support our group with practical experience in machine learning approaches for image analysis from the point of view of another application domain. A trainee could also contribute with experience in inverse modelling, simulation, or computer graphics.
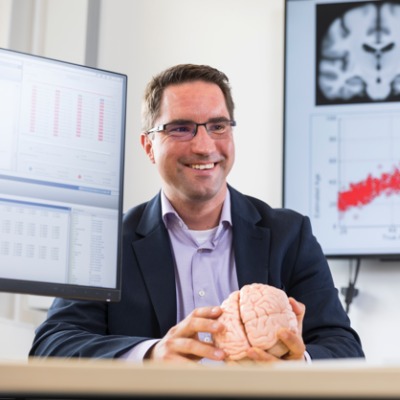
Simon Eickhoff
Simon Eickhoff - FZ Jülich
Jülich Research Centre (FZJ) - Brain and Behaviour (INM-7)
Short summary of your group's research: We develop and apply novel methods to better understand the organization of the human brain and to relate it to behavioral phenotypes in healthy and as biomarkers in clinical populations. To do so, we capitalize on machine learning approaches. In addition to these scientific objectives, we also develop open access methods and tools for data management and reproducible research.
What infrastructure, programs and tools are used in your group? Python, R, MATLAB, HPC, machine learning, neuroimaging
What could a participant of the HIDA Trainee Network learn in your group? How could he or she support you in your group? A HIDA trainee can choose from diverse topics from machine learning, data management to biomarker discovery. For technically oriented trainees we offer opportunities to contribute to our growing set of tools. For application-oriented trainees we offer participation in ongoing projects to uncover brain-behavior relationships.
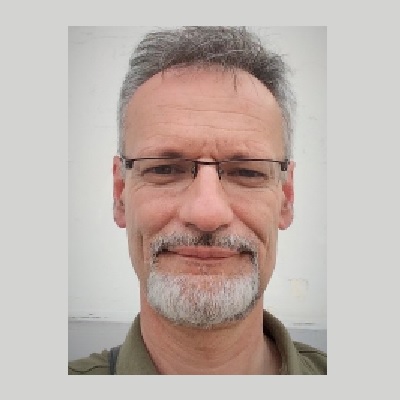
Fabio Fiorani
Fabio Fiorani - FZ Jülich
Jülich Research Centre (FZJ) - Juelich Plant Phenotyping Center/Institute of Bio- and Geosciences IBG2: Plant Sciences
Short summary of your group's research: We focus on the application and development of high-throughput, non-invasive phenotyping methods for screening of plant shoot and root productivity traits. In our group teams of plant biologists, engineers and image processing specialists cooperate to realize screening methodologies for shoot and root growth and architecture combined with physiological measurements of plant performance and plasticity to environmental challenges. Interpretation of results requires the evaluation of multi-dimensional data structures which include dynamic changes of plant phenotypes over time, environmental and imaging sensors data.
What infrastructure, programs and tools are used in your group? We have developed and operate a unique set of automated experimental platforms to analyze plant phenotypes in a minimally-invasive fashion, please click here to learn more.
What could a participant of the HIDA Trainee Network learn in your group? How could he or she support you in your group? Participants to the HIDA Trainee Network would benefit from learning: a) methodologies for high-throughput screening in plants; b) data analyses and visualization of complex data sets; c) experimental designs for plant phenotyping. HIDA Trainee Network participants would support our continued efforts in improving and automating data analyses using existing data stored in several institutional databases.
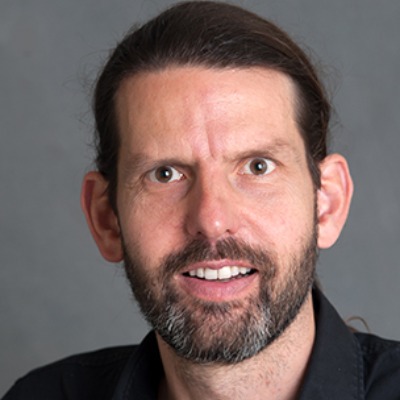
Eric. v. Lieres
Eric von Lieres - FZ Jülich
Jülich Research Centre (FZJ) - Modeling and Simulation @ Institute of Bio- and Geosciences 1
Short summary of your group's research: We provide our local colleagues and the scientific community with state-of-the-art models, algorithms and software. Current challenges in strain selection and optimization as well as process analysis and development are addressed in a strongly data-driven fashion in close collaboration between theory, simulation and experiment. Typical projects involve data analysis, model calibration or training, uncertainty analysis, experimental design. Model predictions are applied for testing hypotheses and focusing experimental work.
What could a participant of the HIDA Trainee Network learn in your group? How could he or she support you in your group? Many projects require advanced numerical methods and high-performance computing, in particular when machine learning/ artificial intelligence, Monte Carlo simulations, global optimization or CFD simulations are applied. We develop and maintain dedicated software packages, most of which are published as open source code https://github.com/modsim.
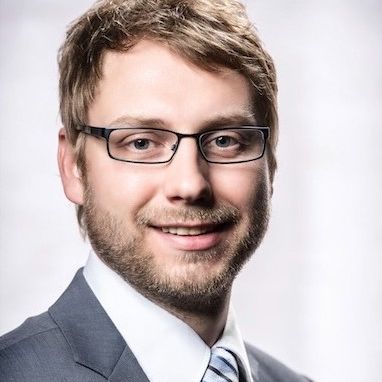
Andreas Lintermann
Andreas Lintermann - FZ Jülich
Jülich Research Centre (FZJ) - Simulation and Data Laboratory "Highly Scalable Fluids & Solids Engineering" (SDL FSE), Jülich Supercomputing Centre (JSC), Institute for Advanced Simulation (IAS)
Short summary of your group's research: The SDL FSE's research focuses, amongst others, on lattice-Boltzmann methods, artificial intelligence, high-performance computing, heterogeneous computing on modular supercomputing architectures, high-scaling meshing methods, efficient multi-physics coupling strategies, and bio-fluidmechanical analyses of respiratory diseases. Furthermore, the SDL FSE aims at supporting users from engineering sciences who have already developed parallel codes but need support for the use of massively parallel systems regarding high scalability, memory optimization, programming of hierarchic computer architectures, and performance optimization on compute nodes.
What infrastructure, programs and tools are used in your group? The group mainly uses the high-performance computing (HPC) systems available at JSC for its various simulation applications. As a simulation code, a massively parallel multi-physics framework, jointly developed with the Institute of Aerodynamics and Chair of Fluid Mechanics, RWTH Aachen University, is employed. The big data outputs generated by the simulation tools are post-processed, e.g., by in-house developed tools, ParaView, machine-learning algorithms, or by Jupyter-based scripts.
What could a participant of the HIDA Trainee Network learn in your group? How could he or she support you in your group? The doctoral researcher will have access to the latest supercomputing hardware installed at JSC and will learn from the experts in the group to develop, adapt, and optimize simulation codes. He or she will support the member of the SDL FSE in running large-scale simulations on the production HPC machines at JSC and post-process the data to gain new insights to physical phenomena in the realm of fluid mechanics. Furthermore, the doctoral researcher will be able to learn how research in a European Center of Excellence in Exascale Computing is performed and has the opportunity to contribute to the corresponding cutting-edge research in bringing AI technologies along various use-cases to exascale.
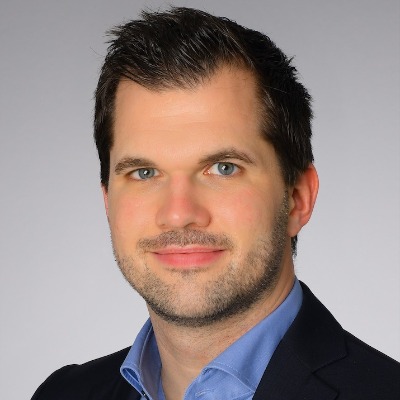
Philipp Lohmann
Philipp Lohmann - FZ Jülich
Jülich Research Centre (FZJ) - Institute of Neuroscience and Medicine, Medical Imaging Physics (INM-4): Brain Tumor Group
Short summary of your group's research: The research of the Brain Tumor Group within the Institute of Neuroscience and Medicine focuses on multimodal imaging in patients with brain tumors, with particular emphasis on amino acid PET in combination with advanced high-field and ultra-high-field MRI. In addition to the development and evaluation of novel PET tracers and PET/MRI methods, the potential of advanced image analysis such as radiomics and deep learning for patients with brain tumors is explored. A major goal of our research is the correlation of imaging findings and radiomics with local neuropathology and histomolecular markers, for which the close collaboration with the surrounding university hospitals in Aachen, Bonn, Cologne, Düsseldorf, and others, is extremely valuable and furthermore offers a high potential for verification and translation of research results into the clinic.
What infrastructure, programs and tools are used in your group? Amino acid PET, advanced MRI, ultra-high-field MRI, hybrid MR/PET, Python, Pyradiomics, PyTorch, LifeX, PMOD, FSL, HDBET, HDGLIO, ITK-SNAP, OsiriX, Matlab, High performance computing, etc.
What could a participant of the HIDA Trainee Network learn in your group? How could he or she support you in your group?
- Amino acid PET in combination with advanced, ultra-high-field MRI in patients with brain tumors
- Advanced image analysis including static and dynamic amino acid PET and MRI
- Feature-based as well as deep learning-based radiomics based on PET and MRI in patients with brain tumors
- Correlation of PET/MRI imaging findings and radiomics with local neuropathology and histomolecular markers
- Establishment of an imaging database of amino acid PET imaging in patients with brain tumors for advanced image analysis
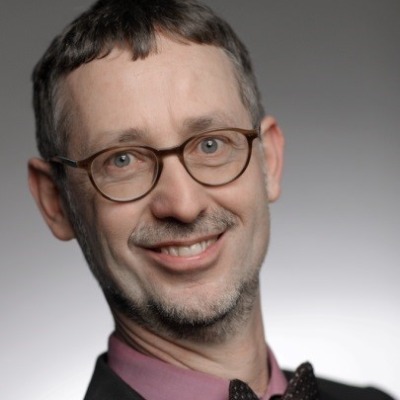
Wolfgang Wiechert
Wolfgang Wiechert - FZ Jülich
Jülich Research Centre (FZJ) - IBG-1: Biotechnology
Short summary of your group's research: Modeling, Simulation and Data Analytics in the fields of systems metabolic engineering and bioprocess development: Cell and process modeling, Omics and bioprocess data processing, process parameter estimation, microbial image analysis, digitalization in lab automation
What infrastructure, programs and tools are used in your group? Hosting CADET and 13CFLUX2 software systems. Using and developing C++, python, Matlab toolboxes. High performance parameter estimation in complex systems, MCMC for parameter estimation and multi model analysis. Deep learning for image analysis. Supercomputing applications in process simulation.
What could a participant of the HIDA Trainee Network learn in your group? How could he or she support you in your group?
Wanted: Expertise in cutting edge Bayesian methods, image analysis and high performance computing
Offering: Challenging realistic problems from the above mentioned fields
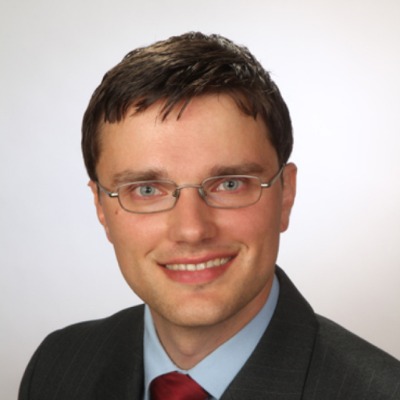
Dieter Weber
Dieter Weber - FZ Jülich
Jülich Research Centre (FZJ) - Ernst-Ruska-Centrum
Short summary of your group's research: We are developing solutions for high-performance data processing, data management and automation in electron microscopy. This includes software like here as well as IT systems and infrastructure. Since modern detectors for electron microscopy can reach a data rate of 50 GB/s, datasets can contain terabytes of data, and electron microscopy is a visual and interactive method, these solutions can be characterized as interactive high performance computing.
What infrastructure, programs and tools are used in your group?
- MapReduce-like processing, "divide and conquer", linear algebra, inverse problem solving
- Python, NumPy, SciPy, Dask, Numba, CuPy, Torch, Jupyter, JupyterHub;
- Linux, Windows;
- Nextcloud, NAS, NFS, CIFS, SSH;
- GitHub, GitLab, Azure Pipelines, PyPI, Zenodo;
- Tox, Pytest, Sphinx;
- product management, project management, software development, test-driven development, Agile;
- PCIe 4.0, SSD RAIDs, 10 GBit Ethernet, AMD EPYC, GPGPU
What could a participant of the HIDA Trainee Network learn in your group? How could he or she support you in your group? You can learn high performance data processing with Python, in particular throughput-oriented processing of large-scale data. That can also involve finding suitable mathematical approaches and implementation strategies to transform an existing "proof of principle" reference implementation into a production-ready high-performance tool. Typically, we achieve speed-ups of 100x to 1000x from a "naive" NumPy or Matlab-based implementation.
Furthermore, you can learn software development methodologies, in particular the "GitHub flow", in combination with quality assurance through automated tests and continuous integration.
Contributions to scientific Open Source software are highly appreciated as a form of support. That can involve software which we actively maintain and develop, work on upstream dependencies, or all other forms of contributions to the Open Source software ecosystem. Such contributions can be in-kind by simply improving existing software and/or releasing new software under an Open Source license, or by joint applications for funding where parts of the resources are dedicated to software development and maintenance.
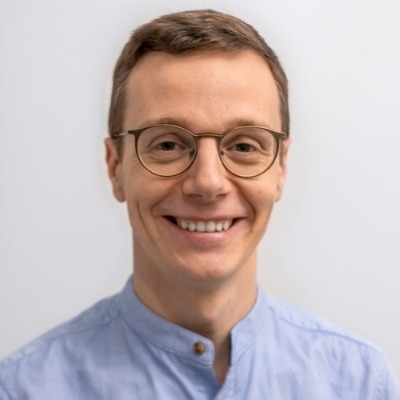
Gabriele Cavallaro
GABRIELE CAVALLARO - FZJ
Jülich Research Centre (FZJ) - Jülich Supercomputing Centre (JSC)
Short summary of your group's research: The joint research group ''High Productivity Data Processing (HPDP)'' at JSC and University of Iceland is highly active in developing parallel and scalable machine (deep) learning algorithms for remote sensing data processing and many other types of applications (i.e., medical research and retail sectors). The main backbone of the research group is the large number of PhD students that are jointly supervised with the University of Iceland. Furthermore, the HPDP works actively with the Cross-Sectional Team Deep Learning (CST DL) and the Helmholtz AI consultant team at JSC.
What infrastructure, programs and tools are used in your group? By being located at JSC, HPDP can rely on HPC technologies with MPI, OpenMP and CUDA (with TensorFlow, Keras, pyTorch, Chainer, Horovod) but also on innovative quantum computing systems.
What could a participant of the HIDA Trainee Network learn in your group? How could he or she support you in your group? Learn how data intensive computing approaches have become indispensable tools to deal with the challenges posed by applications from a diverse range of applications.
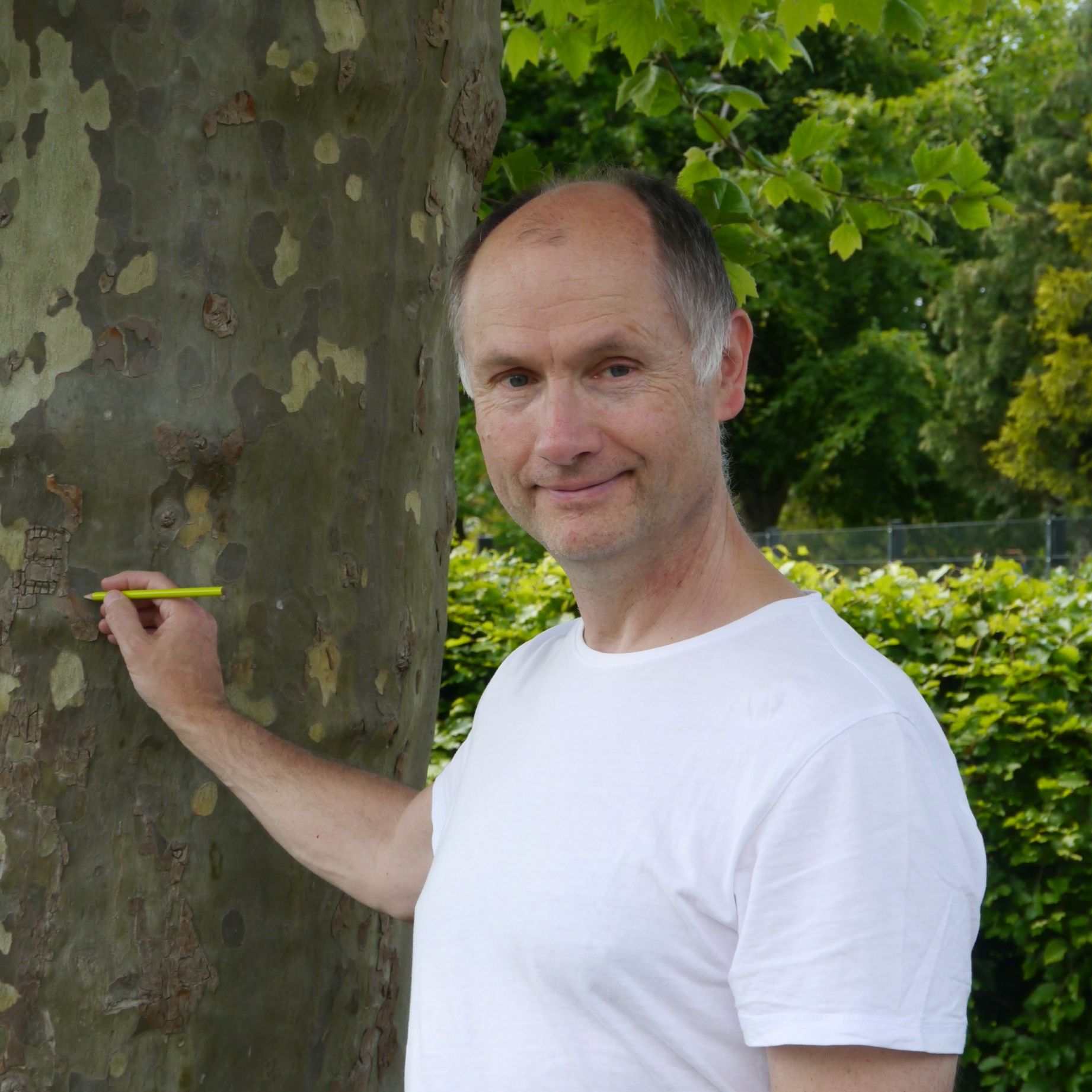
Martin Schultz
Martin Schultz - FZJ
Jülich Research Centre (FZJ) - Earth System Data Exploration research group at the Jülich Supercomputing Centre
Short summary of your group's research: ESDE explores a wide range of machine learning methods for the analysis and forecasting of weather, climate and air quality data. We focus primarily on large, computationally intensive problems and adopt high-end deep learning approaches. Next to machine learning research we are also strongly interested in developing FAIR big data workflows. The 20+ group members have diverse educational backgrounds and build a lively international team of highly motivated researchers and software developers.
What infrastructure, programs and tools are used in your group? We make good use of JSC's high-end computing systems (https://go.fzj.de/juwels) and also operate on smaller GPU clusters. Tensorflow and Pytorch are our main (Python) software libraries. We also use containers and tools like Horovod to port ML codes to the supercomputer.
What could a participant of the HIDA Trainee Network learn in your group? How could he or she support you in your group? If you already have a good background in advanced machine learning techniques (i.e. beyond MLP) you may take it to the next level with a visit to our group and we will be happy to teach you the necessary basics of weather and air pollution science so that you can apply your skills to the problems we work on in our group. If you have a strong background in environmental science but only limited experience with machine learning (but you know how to program in Python) you may learn a lot about more sophisticated deep learning methods and we might be able to integrate your specific experience to better address our environmental research questions.
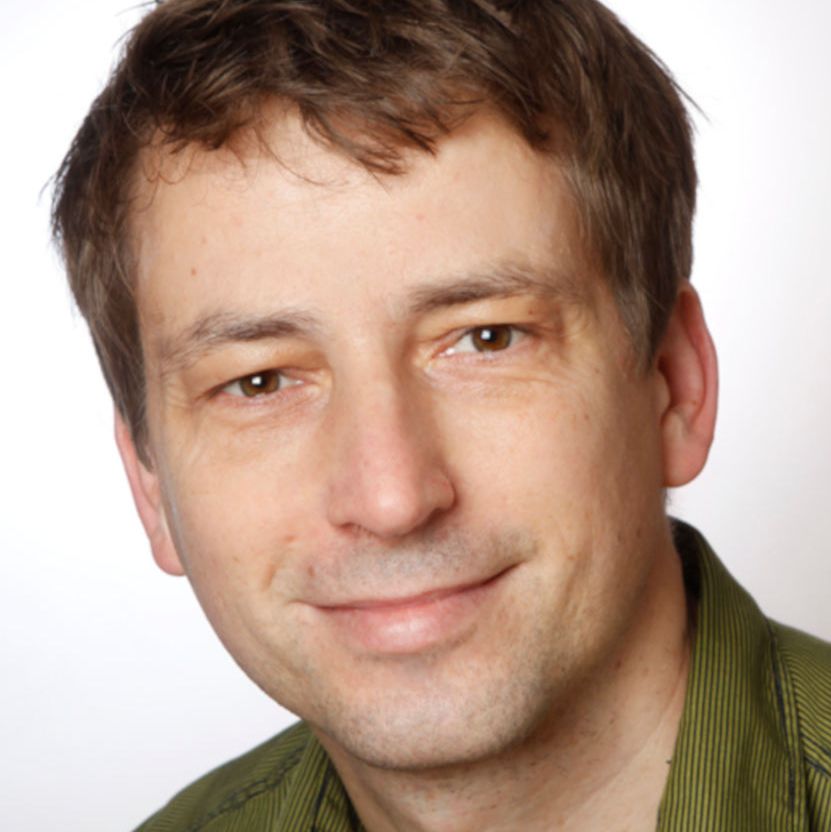
Christoph Lerche
Christoph Lerche - FZJ
Jülich Research Centre (FZJ) - PET Physics Group, Medical Imaging Physics - Institute of Neurosciences and Medicine (INM-4)
Short summary of your group's research: The PET physics group focusses on
- PET methodology development (image reconstruction and data corrections as scatter correction and attenuation correction)
- Multimodal Imaging (Applications and Methodology for combined PET/MR and PET/MR/EEG acquisitions) System development of an UHF MR compatible BrainPET insert
- Quantitative Methods for PET and PET/MR imaging
What infrastructure, programs and tools are used in your group?
- 3T MR-PET insert, consisting of a high resolution BrainPET, (a prototype by Siemens), and a commercial 3T MAGNETOM Trio MRI scanner with several phantoms
- Access to the Radiochemistry department for generation of Radio-tracer and Radionuclides
- Access to High Performance Computing Installations
- Access to PET and MR patient and volunteer data from other studies (neuroreceptor/neurotransmitter studies, neuroonkology)
- Monte Carlo Simulation tools Geant4/Gate, analysis tools: PMOD, SPM
What could a participant of the HIDA Trainee Network learn in your group? How could he or she support you in your group? Our group offers the following expertises
- Thorough understanding of PET systems and Image-formation from gamma photon detection to image reconstruction including all relevant corrections (scatter, attenuation, randoms, dead time)
- Unique access to a dedicated 3T MR BrainPET insert and a 7T human MRT in a research setting with all technical an league requirements to run studies involving human volunteers
- Starting in 2023: access to a in-house developed 7T MR BrainPET insert for humans
- We would highly benefit from guest researcher with expertises in Image post processing (PET and MR), Data science for information extraction from multi parametric and multimodal images, Monte Carlo Simulations
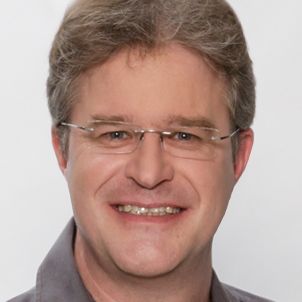
Maik Boltes
Maik Boltes - FZJ
Jülich Research Centre (FZJ) - Pedestrian Dynamics – Empiricism at the Institute for Advanced Simulation: Civil Safety Research (IAS-7)
Short summary of your group's research: Our institute studies the dynamics of pedestrians to understand their behavior and thereupon develop reliable models for enhancing the safety of people inside crowds. Controlled experiments allow the quantitative description of the dynamics and enable the analysis of selected parameters under well-defined constant conditions. Data of these experiments is collected and analyzed by appropriately selected sensors and self-developed software.
What infrastructure, programs and tools are used in your group? During our experiments we are collecting a huge amount of data from (depth) cameras, motion capturing systems, IMU sensors, pressure sensors, heart rate sensors and electrodermal activity sensors sometimes combined with questionnaire data.
Our software is written in C++ or Python like our open-source tool PeTrack, which automatically extracts accurate pedestrian trajectories from videos.
Gathered data of all our experiments can be found in our open data archive.
What could a participant of the HIDA Trainee Network learn in your group? How could he or she support you in your group? We are a multidisciplinary young group of physicists, mathematicians, engineers, sociologists, psychologists, and computer scientists.
Our institute is one of the leading institutions in the field of experimental pedestrian research.
The dynamics and behavior of people in a crowd have many influences. The diversity of performed experiments and the amount of experimental data in our group is large. We learned in the past that a new view, method or discipline can give us another or extended insight to our data so that we would be happy to welcome you to our group.
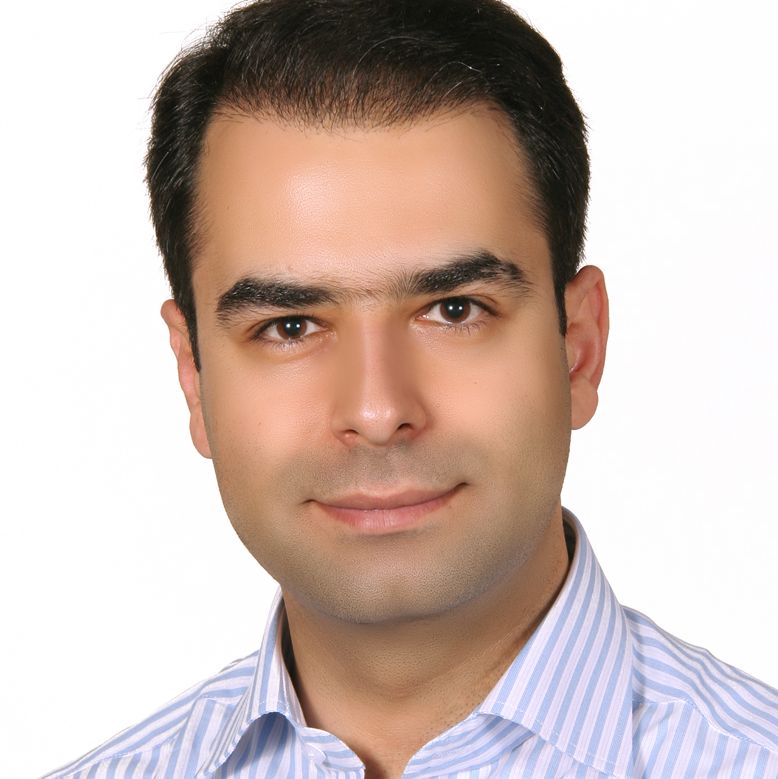
Masoud Tahmasian
Masoud Tahmasian - FZJ
Jülich Research Centre (FZJ) - System Medicine, Brain and Behaviour (INM-7), Institute of Neuroscience and Medicine
Short summary of your group's research: We use a multi-level approach utilizing computational tools and big data to gain an overall insight into neurological and psychiatric disorders to pave the way for personalized medicine. In particular, we focus on the interplay between sleep/sleep disorders and medical and mental conditions using phenotypic and multimodal neuroimaging data (e.g., sMRI, fMRI, PET).
Masoud Tahmasian is part of Prof. Dr. Simon Eickhoff's group on System Medicine.
What infrastructure, programs and tools are used in your group? We use state-of-art methodologies such as machine-learning to big clinical and population-based databases using high-performance computing tools.
What could a participant of the HIDA Trainee Network learn in your group? How could he or she support you in your group? The guest researcher will apply several analyses including machine-learning to identify the role of sleep disturbances and brain structures on predicting neuropsychiatric conditions such as depression using a large-scale general-population sample (e.g., UKBB).
He/She should perform the analyses independently and help us to build a nice analytic framework to find the neurobiological mechanisms behind the link between sleep and neuropsychiatric disorders.
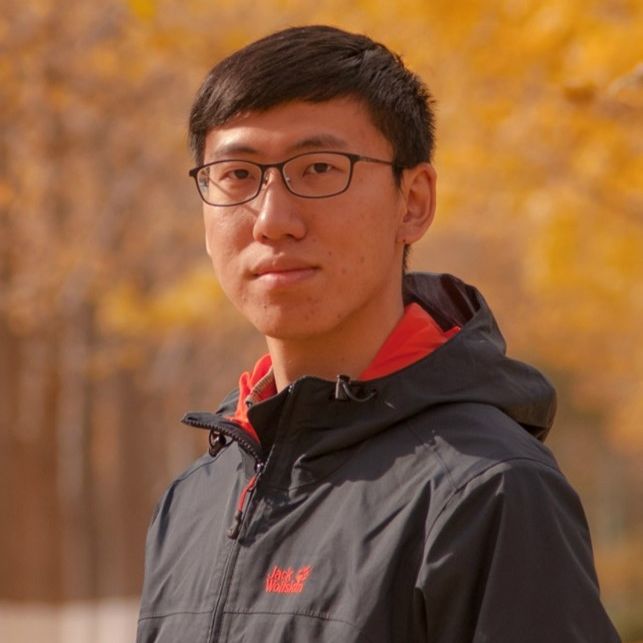
Yuankai Yang
Yuankai Yang - FZJ
Jülich Research Centre (FZJ) - Reactive Transport Modelling Group / Institute of Energy and Climate Research, IEK-6Reactive Transport Modelling Group / Institute of Energy and Climate Research, IEK-6
Short summary of your group's research: Analysing complex coupled thermo-hydraulic-mechanical-chemical-transport processes relevant to environmental systems on different scales.
My research at IEK-6 focuses on the development of complementary computational approaches to analyse and interpret the complex coupled THMC-processes in the near- and far-field of geological repositories for radioactive wastes on different scales, employing high-performance computing (HPC) resources provided by the Jülich Supercomputing Centre. This work aims at an enhanced process and system understanding across scales as well as at the reduction of uncertainties and conservatisms in performance assessments, contributing thus to the scientific basis for an in-depth comparison of different repository concepts and sites as required for the German site selection procedure.
What infrastructure, programs and tools are used in your group? We use the Lattice Boltzmann Method (LBM) for simulations. The High-Performance Computing (HPC) Cluster JURECA-DC is our main computational resource. Currently, we are using CUDA, Matlab, Python, Comsol, and Fortran for developing our numerical framework and data analysis.
What could a participant of the HIDA Trainee Network learn in your group? How could he or she support you in your group? The guest researcher could learn the Lattice Boltzmann method algorithm, multiscale modeling processes, and an understanding of coupled THMC-processes in geological porous media. Use of our techniques and software.
We will support the GPU High-Performance Computing Cluster in JURECA-DC. We will cooperate with the guest to develop their LBM codes. We also welcome guests with a basic background in machine learning, who want to perform joint research with us relevant to environmental systems.
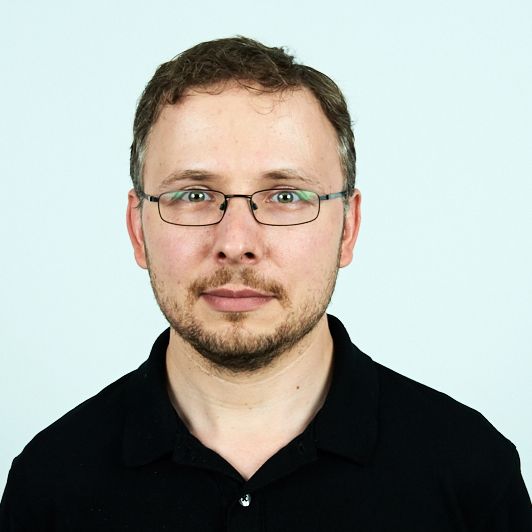
Johannes Laube
Johannes Laube - FZJ
Jülich Research Centre (FZJ)- Stratosphere (ICE-4)
Short summary of your group's research: Our main focus is on flying sensors on balloons to stratospheric altitudes up to 35 km. For instance, we collect air samples and then subsequently analyse these for their content of trace gases important for stratospheric ozone (such as CFCs or halons) or climate (e.g., CO2 or HFCs). We can use the data to better understand the state of the ozone layer or circulation changes at altitudes well above the reach of aircraft.
What infrastructure, programs and tools are used in your group? Observation platforms are aircraft and balloons, measurements are mostly based on cavity-ringdown spectroscopy and mass spectrometry. Current project examples are the ERC Starting Grant EXC3ITE and we are also involved in Modular Observation Solutions for Earth Systems MOSES. We closely collaborate with the atmospheric modelling community, e.g. the Chemical Lagrangian Model of the Stratosphere CLaMS.
What could a participant of the HIDA Trainee Network learn in your group? How could he or she support you in your group? The learning experience would include the challenges of observations in as hostile an environment as the stratosphere, measurement and data retrieval techniques, and inferring key parameters such as average stratospheric transit times from the various trace gas data. Especially the latter part is where a guest researcher might be able to help us to improve our algorithms and procedures.
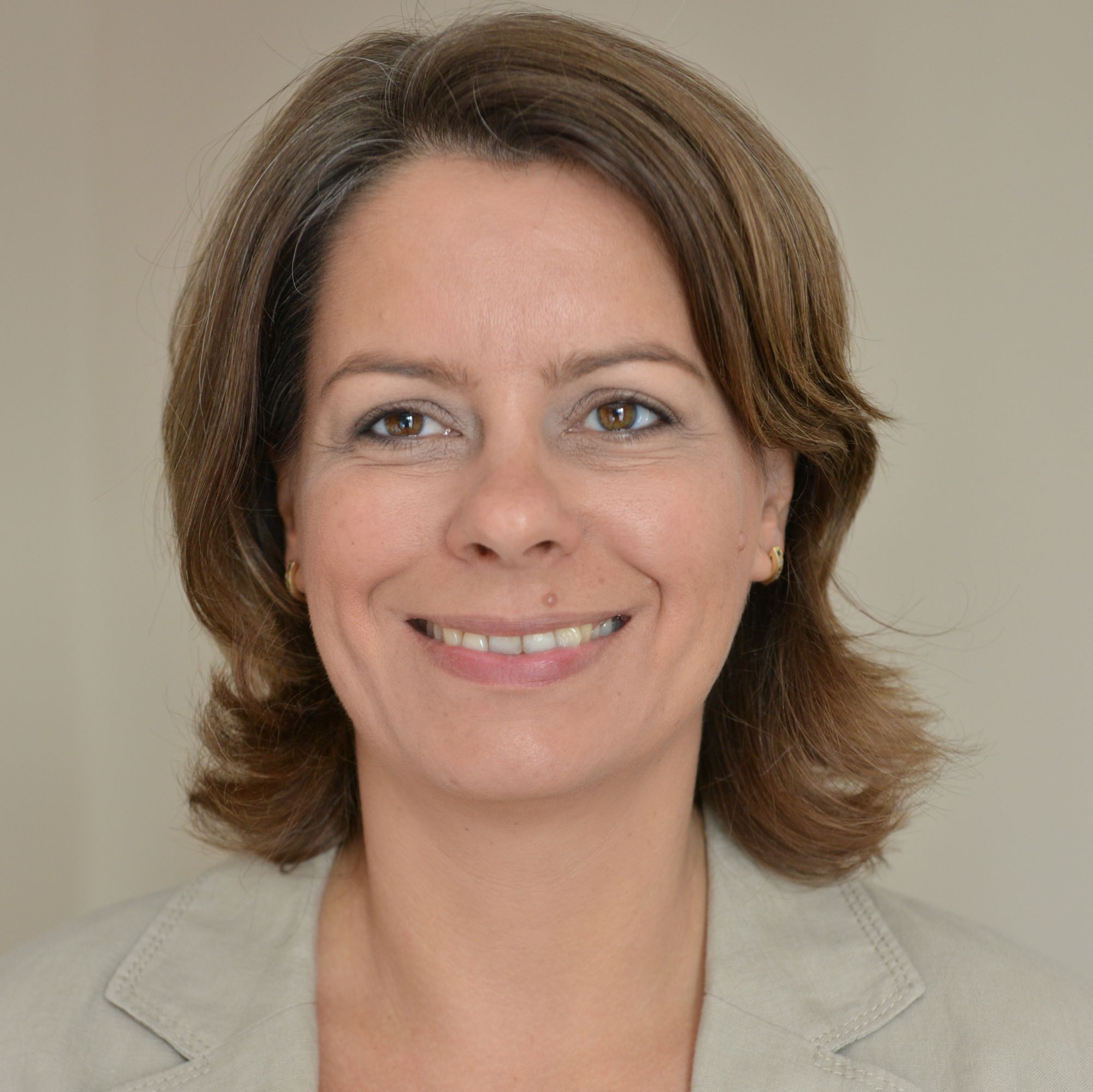
Simone Beer
Simone Beer - FZJ
Jülich Research Centre (FZJ) - Institute of Neuroscience and Medicine (INM-2)
Short summary of your group's research: We use explainable AI on population-based studies to target neurodegenerative diseases, e.g. Alzheimer's disease. Machine learning algorithms like decision trees or tree ensembles can learn patterns in data, and the explanations are therefore promising tools to address interactions of genes, environment and lifestyle with respect to a certain trait or disease. We seek to evaluate and use different methods of explainable AI with respect to scientific discovery tasks.
What infrastructure, programs and tools are used in your group? Python, GitHub, access to various clinical and population-based databases like ADNI (the Alzheimer's Disease Neuroimaging Initiative), PET, hybrid MRI/PET and various laboratory techniques for preclinical and clinical research as well as research in basic neuroscience in a highly interdisciplinary environment.
What could a participant of the HIDA Trainee Network learn in your group? How could he or she support you in your group? A guest researcher will support in evaluating explainable AI for scientific discovery, especially with respect to the interaction of genetics, environment and lifestyle in neurodegenerative diseases.
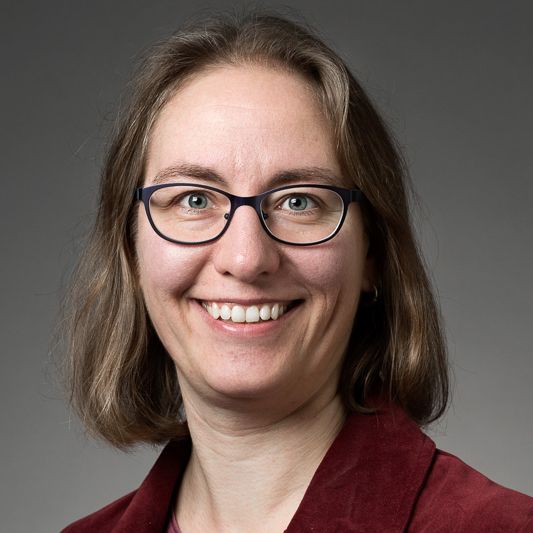
Ira Assent
Ira Assent - FZJ
Jülich Research Centre (FZJ) - Data Analytics and Machine Learning (IAS-8, Institute for Advanced Simulation)
Short summary of your group's research: We aim at advancing theory and application of data sciences with a focus on image processing and computer vision for imaging as a scientific measurement tool. Our interests range from use-inspired, method-driven theory to application-driven research.
What infrastructure, programs and tools are used in your group? We work with state-of-the-art methods and algorithms in machine learning and data mining; most of our implementations are in python, and we make use of gpu-parallelization for efficiency and scalability; we devise methods that support scientists in other fields in analyzing and handling their data
What could a participant of the HIDA Trainee Network learn in your group? How could he or she support you in your group? You can learn more about how to train machine learning models, develop (variants of) such models, devise efficient algorithms, or use them to work with your data or with the data provided by our collaborators.
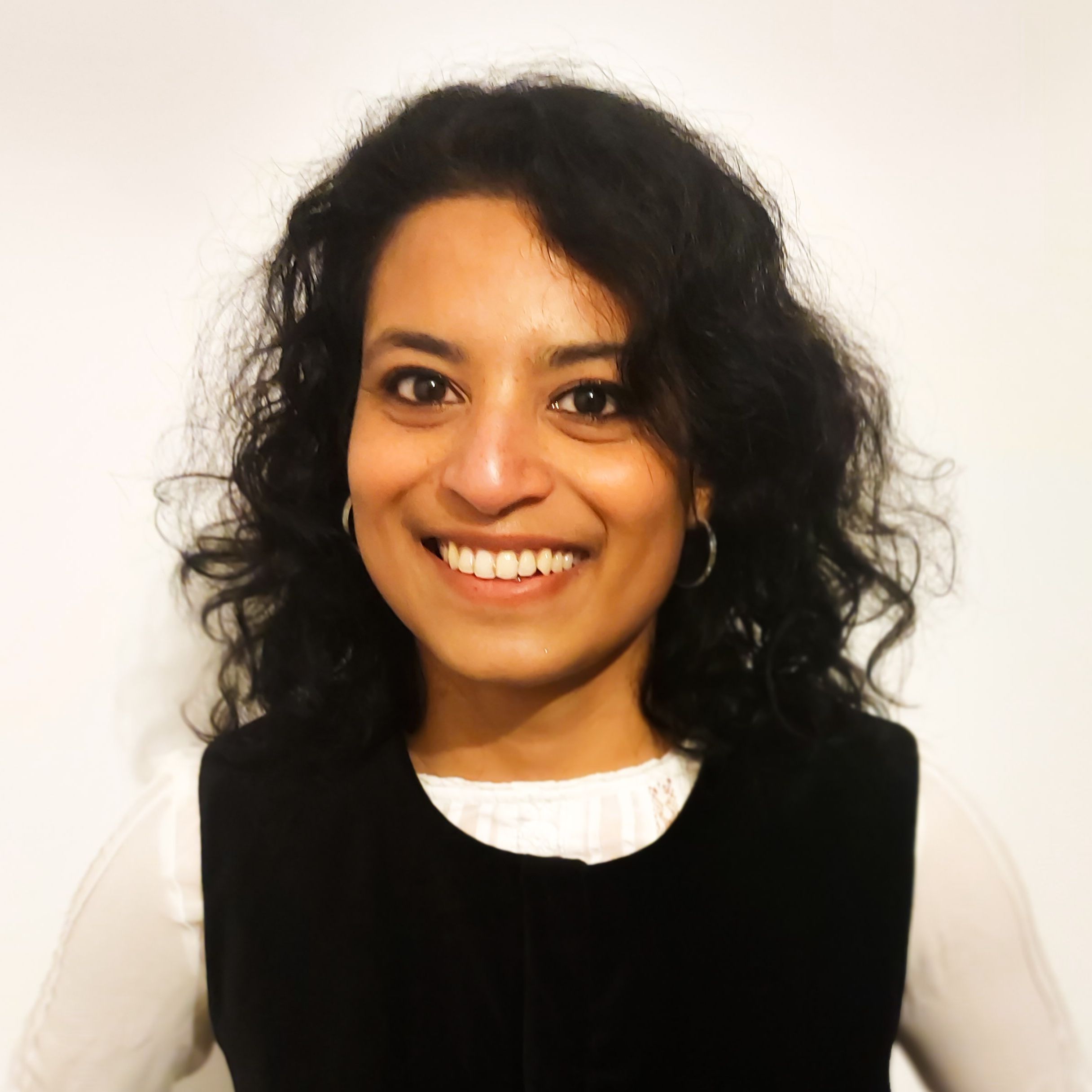
Jenna Poonoosamy
Jenna Poonoosamy - FZ Jülich
Jülich Research Centre (FZJ) - The Institute of Energy and Climate Research - Nuclear Waste Management (IEK-6)
The institute performs cutting-edge research in the fields of nuclear waste management and safety, considering the process of “Energiewende”, the transition of the German energy system. This comprises fundamental as well as applied research and development for the safe management of nuclear wastes, covering issues from the atomic scale to the macroscopic scale of actual waste forms and waste packages or the engineered barrier system of deep geological repositories for nuclear wastes.
Short summary of your group's research: We apply innovative experimental and computational approaches across the scale to analyze and interpret complex coupled thermal-hydraulical-mechanical-chemical (THMC) processes in porous media related to energy applications. Recently, I have acquired an ERC project to investigate the gas water mineral interactions in confinement https://cordis.europa.eu/project/id/101040341. In this project, AI and Neural network tools will be used to (i) automate the analysis of microfluidics experiments and process experimental results (ii) derivation of constitutive equations to rationalize complex processes and (iii) accelerate geochemical processes.
What infrastructure, programs and tools are used in your group? Reactive transport tool software (Pflotran, Openegeosys-Gems, comsol multiphysics). Geochemical simulation. Image processing based on machine learning. In addition, we also have a microfluidic laboratory equipped with confocal Raman microscopy, IR and fluorescence microscopy.
What could a participant of the HIDA Trainee Network learn in your group? How could he or she support you in your group? Multiphase flow using Comsol multiphysics and geochemical modelling using phreeqc or GEMS. We have conducted novel experiments in the field of geochemistry; a lab on a chip approach to investigate radionuclide incorporation in mineral phases in a confined nano volume of (1-10nL) solution. The guest researcher would work on simulating droplet generation using comsol multiphysics and conduct geochemical modelling.
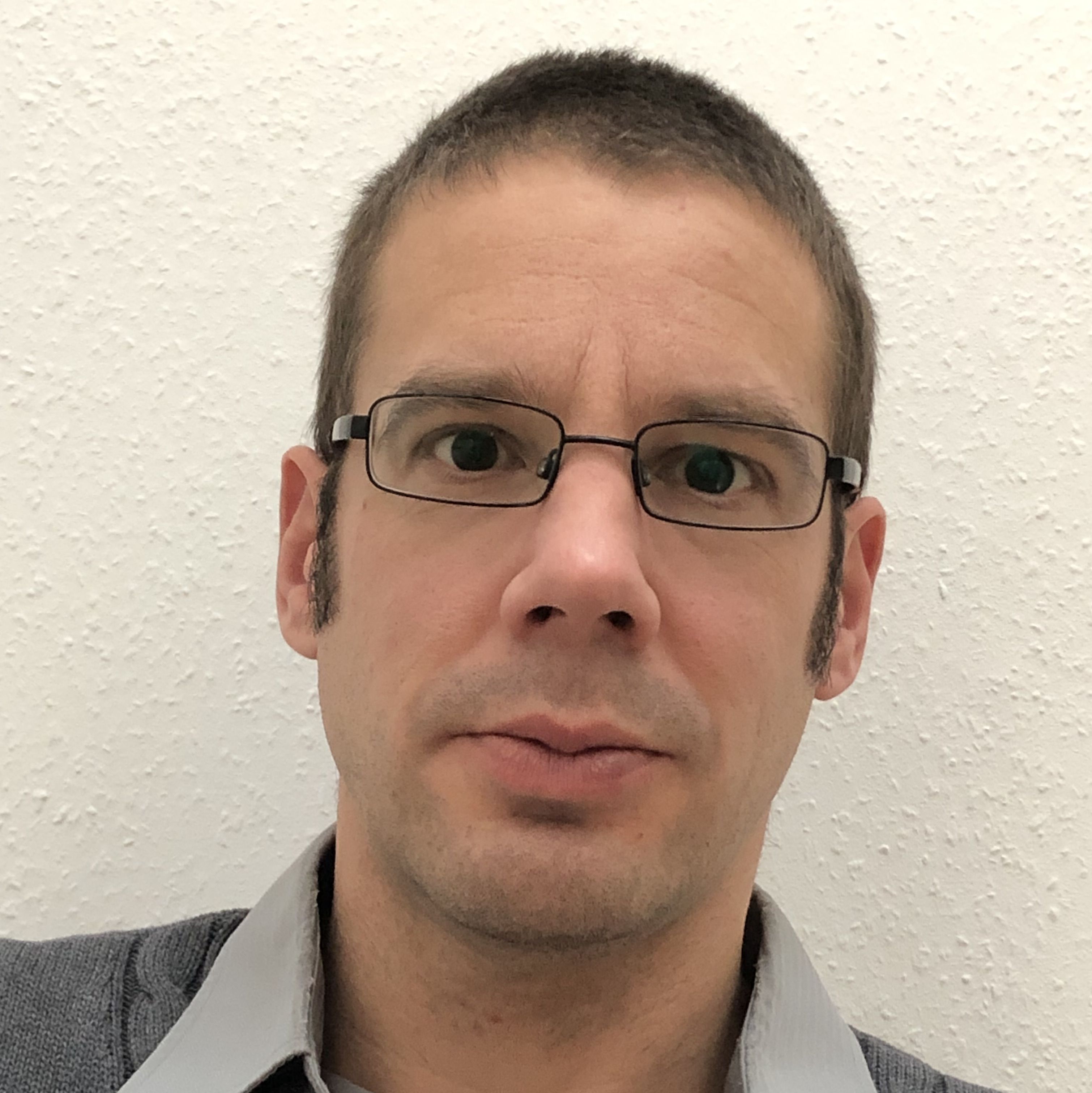
Andreas Kleefeld
FZJ - Andreas Kleefeld
Jülich Research Centre (FZJ) - Algorithms, Tools and Methods Lab (ATML) "Numerical and Statistical Methods", Mathematics and Education Department, Jülich Supercomputing Centre
Three-sentence summary of your group's research: The ATML "Numerical and Statistical Methods" develops, implements, and tests new procedures/methods in the area of stochastic ordinary and partial differential equations that arise, for example, in image processing, acoustic, electromagnetic, and elastic scattering problems, as well as in reaction-diffusion-advection equations. In this context, the group also deals with algorithms for the solution of ill-posed inverse problems and the computation of eigenvalue problems taking real-world data into account. Furthermore, the group offers software support for supercomputers.
What infrastructure, programs and tools are used in your group? We develop software and tools in Fortran, Matlab, and Python depending on the requirements of the project.
For instance, solvers for acoustic, electromagnetic, and elastic scattering for time-harmonic waves in unbounded domains have been implemented and further used for related inverse problems. The high performance infrastructure of the Jülich Supercomputing Centre is at our disposal.
What could a guest researcher learn in your group? How could he or she support you in your group? A researcher in our group will learn how to efficiently solve partial differential equations in unbounded domains with boundary integral equations numerically. A recent development is mainly focusing on the efficient numerical calculation of interior transmission eigenvalues which can be used to visualize the interior of a given three dimensional object to uncover location, size, and shape of an inclusion for either given real-world or synthetic data which is the aim in non-destructive testing. A researcher can support our group with the development of this algorithm for HPC. Moreover, the mathematical theory still needs to be established. Finally note that a detailed description of our projects can be found at our homepage.
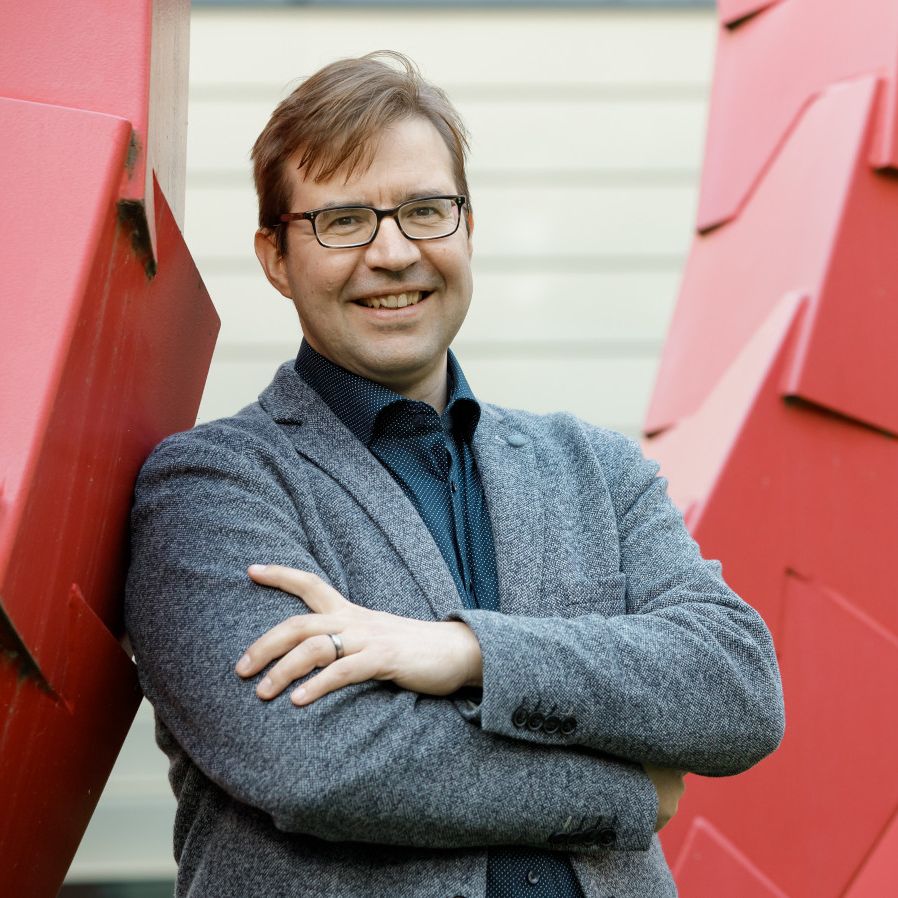
Stefan Sandfeld
FZJ - Stefan Sandfeld
Jülich Research Centre (FZJ) - Materials Data Science and Informatics
Three-sentence summary of your group's research: Research work at the IAS-9 is located at the intersection of data science and machine learning, research data management and semantic technology, and simulation in the field of computational materials science. In the data science department we investigate how predictive and generative machine learning models are able to replace or accelerate computationally expensive aspects of simulations; and as a second main topic we perform data mining of and method development for microscopy images and data. In the field of materials simulations we are particularly interested in predicting the structure-property relation in the context of solid-mechanical systems. In the field of RDM we are involved in software development and design ontologies and metadata schemas together with domain scientists.
What infrastructure, programs and tools are used in your group? Probably the main "tool" used in the IAS-9 is programming. 90% of the time, we use Python for writing code, but once in a while there is also some C/C++ or Java. Half of our activities are taking place on HPC hardware.
What could a guest researcher learn in your group? How could he or she support you in your group? A guest researcher can learn how (data) science is done in our team and how we sometimes also collaboratively work together on small projects -- sometimes with people from the field of simulation, research data management, and data science.
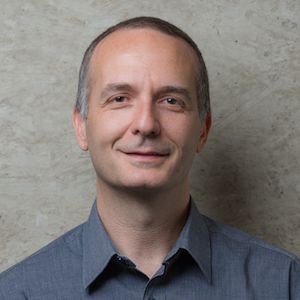
Eduardo di Napoli
Eduardo di Napoli - FZJ
Jülich Research Centre (FZJ) - Simulation and Data Lab Quantum Materials, Jülich Supercomputing Centre, Institute for advanced Simulations
Short summary of your group's research
The Simulation and Data Laboratory Quantum Materials (SDLQM) serves as a dynamic hub for pioneering research at the intersection of simulation science and quantum materials. With a unique emphasis on leveraging High-Performance Computing and cutting-edge scientific Machine Learning, SDLQM offers a rich repository of expertise in quantum-based simulations within the realm of Materials Science. Our lab operates as a catalyst for innovation, actively engaging in dedicated projects and hosting research initiatives that delve into the essential domains of method refinement, algorithmic enhancement, and performance optimization. At the forefront of simulation science, SDLQM thrives on fostering cross-disciplinary collaborations spanning the global landscape. We take pride in our robust engagement within both the European and Japanese research arenas. Within the EU, our lab spearheads pioneering research and development initiatives, propelling the domain of Materials for Energy to new horizons. We are resolutely focused on facilitating exascale simulations of quantum transport, unraveling complexities that hold the key to transformative advancements. Our endeavors transcend borders, as we cultivate robust ties with esteemed partners in Japan, notably nurturing a dynamic synergy with the illustrious RIKEN supercomputing center in Kobe.
What infrastructure, programs and tools are used in your group?
Guided by an unwavering commitment to excellence, SDLQM not only facilitates extensive-scale simulations on state-of-the-art HPC platforms but also pioneers the training of intricate learning models driven by insights garnered from in-silico simulations. SDLQM operates at the heart of the Juelich supercomputing center, a pioneering institute that presently houses one of the largest supercomputers across the European Union. This convergence of minds and machines is set to elevate to unprecedented heights, with Europe's inaugural exascale supercomputer slated for 2024. Immerse yourself in a realm of unbounded possibilities, as the lab cultivates expertise encompassing high-performance computing, parallel programming, numerical linear algebra, quantum transport simulation, functional Renormalization Group methods and Tensor Networks. As a nexus at the frontier of knowledge, we seamlessly unite the realm of materials science simulation with the realm of massively parallel machines, thereby nurturing a community driven by cutting-edge innovation and collaborative excellence.
What could a guest researcher learn in your group? How could he or she support you in your group?
The doctoral researcher will be granted privileged access to JSC's cutting-edge supercomputing hardware, ushering them into the realm of computational supremacy. Working in close tandem with the group's accomplished experts, they will embark on a transformative journey of code development, adaptation, and optimization. Moreover, the doctoral researcher will gain firsthand exposure to the dynamic intersection of numerical linear algebra and materials science simulation. This immersive journey will encompass code integration, delving into the intricacies of parallel programming best practices, and honing indispensable software management skills. Join us in shaping the future of quantum-centric materials research, where innovative simulations and data-driven discoveries converge.
Helmholtz Center HEREON
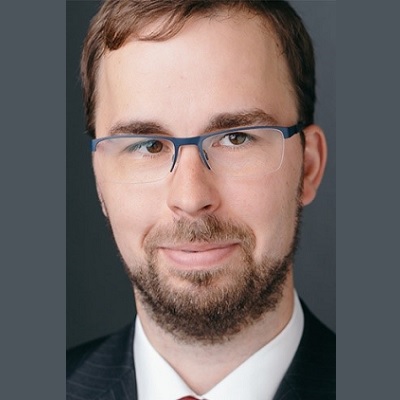
Christian Feiler
Christian Feiler - Hereon
Helmholtz-Zentrum Hereon - Department of Interface Modelling at the Institute of Surface Science
Short summary of your group's research: The research activities at the Institute of Surface Science revolve around the development of ultra‐light‐weight materials and surface protection technologies for the application areas air and ground transportation, infrastructures, regenerative medicine, as well as energy storage and functional materials. Gaining control over surface reactivity characteristics and a reliable prediction of service-life aspects of the employed materials are prerequisites to unlock their full potential in the respective application areas. In this context, we utilise information derived from multiscale simulations, experimental investigations in labs and field data from industrial end-users to gain a comprehensive insight into the material behaviour and employ a variety of supervised and unsupervised machine learning techniques for the discovery of effective additives prior to experimental testing as well as to predict how materials and structures behave during their service-life after exposure to the environment with focus on a sustainable circular economy.
What infrastructure, programs and tools are used in your group? Access to Hereon’s HPC Cluster, HPC workstations in the group for less demanding jobs, Several simulation software packages (DFT, MD, FEM) and tools to generate molecular descriptors (alvaDesc, rdkit), Python-based machine learning workflows.
What could a participant of the HIDA Trainee Network learn in your group? How could he or she support you in your group?
Our offer:
- Insights into material science with focus on materials degradation and corresponding corrosion mitigation strategies.
- Techniques to generate molecular descriptors for quantitative structure-activity/property relationship models and approaches to identify features that are relevant to the chosen target property as well as subsequent model development.
- Opportunity to validate the robustness of your machine learning model(s) directly with our experimentalists who provide the training data.
Your support:
- You could either apply your machine learning knowledge to our existing training data or shape a new project together with our interdisciplinary team with focus on corrosion mitigation and/or predictive maintenance.
- You could employ your experience in materials modelling to generate additional input features for our predictive models.
- You could help us to develop tools that can be utilised by end-users without any domain expertise
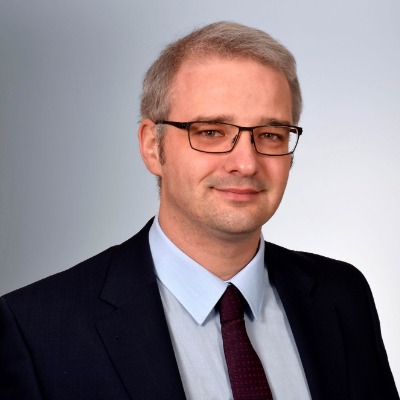
Paul Jerabek
Paul Jerabek - Hereon
Helmholtz-Zentrum Hereon - Group for Modeling of Hydrogen Storage Materials at the Institute of Hydrogen Technology
Short summary of your group's research: We perform state-of-the-art multi-scale simulations of hydrogen storage materials, e.g. interstitial metal hydrides or complex hydride systems. For that, we develop digital workflows bridging the atomistic scale with the mesocopic regime by coupling accurate ab-initio calculations with thermodynamic modeling and phase-field simulations. Our set out goal is to be able to understand and accurately predict materials properties as independently from experimental input as possible.
What infrastructure, programs and tools are used in your group? We have access to Hereon's own HPC cluster (more than 160 nodes with 48 CPUs/node) that we utilize for running various molecular and periodic quantum chemical program packages (VASP, QuantumEspresso, CASTEP, ORCA, Gaussian etc.)
Furthermore, we use open-source software to perform thermodynamic modeling (OpenCALPHAD) and phase-field simulations (FiPy, MOOSE).
What could a participant of the HIDA Trainee Network learn in your group? How could he or she support you in your group?
What you can learn in our group:
- You will be able to gain or deepen your understanding in quantum chemical methods like DFT or AIMD for structural optimization, calculation of reaction energies and pathways and prediction of spectroscopic properties
- You will learn how to perform thermodynamic modeling to predict phase diagrams for multi-component systems via an ab-initio assissted CALPHAD methodology
- You can be trained in utilizing phase-field simulations for prediction of microstructure evolution and kinetic properties of materials on the mesoscale
How you can support us:
- You can complement our team by working with our established methodologies on your own project on the topic of hydrogen storage materials
- You can help with automatizing and streamlining some of our scale-bridging, digital workflows
- You can kickstart our planned activities for utilizing machine-learning techniques for some of our computational methods
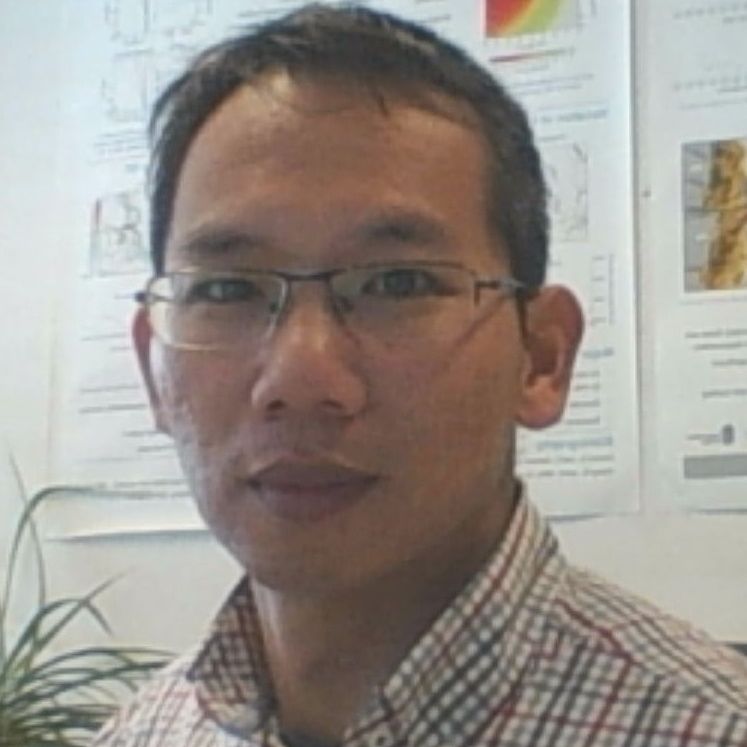
Wenyan Zhang
Wenyan Zhang - Hereon
Helmholtz-Zentrum Hereon - Department of Sediment Transport and Morphodynamics
Short summary of your group's research: Continental margins are constantly changing their shape through erosion and sediment deposition. In terms of climate protection and sustainable coastal development, the “Sediment Transport and Morphodynamics” department studies in detail the interactions between morphodynamics, ecosystem functions and biogeochemistry. We apply process-based models combined with artificial intelligence to investigate particle transport, coastline change, morphodynamics and their role in ecosystem functioning.
What infrastructure, programs and tools are used in your group? We develop and apply numerical models (physics-biology-biogeochemistry coupled) with parameterizations optimized by data-driven approaches. The models include SCHISM, ROMS and Delft3D.
What could a participant of the HIDA Trainee Network learn in your group? How could he or she support you in your group? We provide guidance to learn hybrid modeling combining process-based models and data-driven optimization. We hope the guest research could bring the expertise in artificial intelligence with application to remote sensing data (especially satellite images) and causal linkage identification from multivariate datasets.
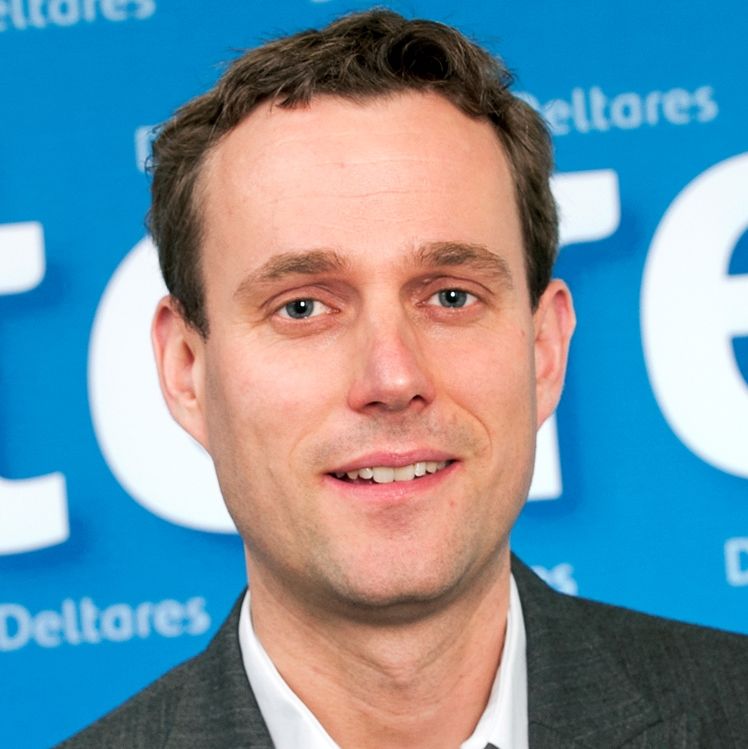
Laurens Bouwer
Laurens Bouwer - HZG
Helmholtz Zentrum Hereon - Geesthacht Centre for Material and Coastal Research (HZG) - Climate Service Center Germany
Three-sentence summary of your group's research: The interdisciplinary team at GERICS develops scientifically based prototype products and services to support decision-makers in politics, business and public administration in adapting to climate change. We are located in the historic "Chilehaus" in the centre of Hamburg. Specifically, my group develops applications in the field of water resources (water supply, flooding), as well as socio-economic impacts, such as damages and health impacts.
What infrastructure, programs and tools are used in your group? We operate our own regional climate model REMO, and participate in the WCRP EURO-CORDEX network. In addition, we develop state of the art tools for pre- and post-processing of climate and other environmental data.
What could a guest researcher learn in your group? How could he or she support you in your group? We use data science methods and machine learning for improving simulations as well as visualistion of our results. Example applications are the application of ML for data post-processing of weather and climate predictions (Link), impact models for flood damage assessment (Link), and health impact modelling (Link). We are also open to accept novel ideas and proposals of other applications of data science methods, that fit the goals of GERICS and our product portfolio.
Helmholtz-Zentrum Dresden-Rossendorf - HZDR

Michael Bussmann
Michael Bussmann - HZDR
Helmholtz-Zentrum Dresden-Rossendorf (HZDR) - Center for Advanced Systems Understanding (CASUS)
Short summary of your group's research: The Center for Advanced Systems Understanding is a new center working in the field of data-driven systems science. We strive to understand complex systems in an interdisciplinary way using newest digital technologies. From modelling the formation of complete organisms from a single cell to studying the interplay between ecosystems and biodiversity, from understanding what exoplanets look like to working on the mobility of the future, CASUS provides a diverse and welcoming research culture.
What infrastructure, programs and tools are used in your group? We provide access to a large HPC systems for both high performance compute simulations and large-scale AI. We have expertise in high performance computing, scalable AI, human-machine interaction, and strive for the development of professional research software and solutions. We have access to some of the largest compute resources on the planet.
What could a participant of the HIDA Trainee Network learn in your group? How could he or she support you in your group? Trainees have a large variety of options, from learning High Performance Computing (including GPU and FPGA computing) to newest developments in AI (physics-informed NN, Invertible NNs, Normalizing Flows, ...), from applied mathematics and data science foundations to using their skills for a variety of applications in physics, ecology, digital health, autonomous systems, cyber security, earth systems science and more.
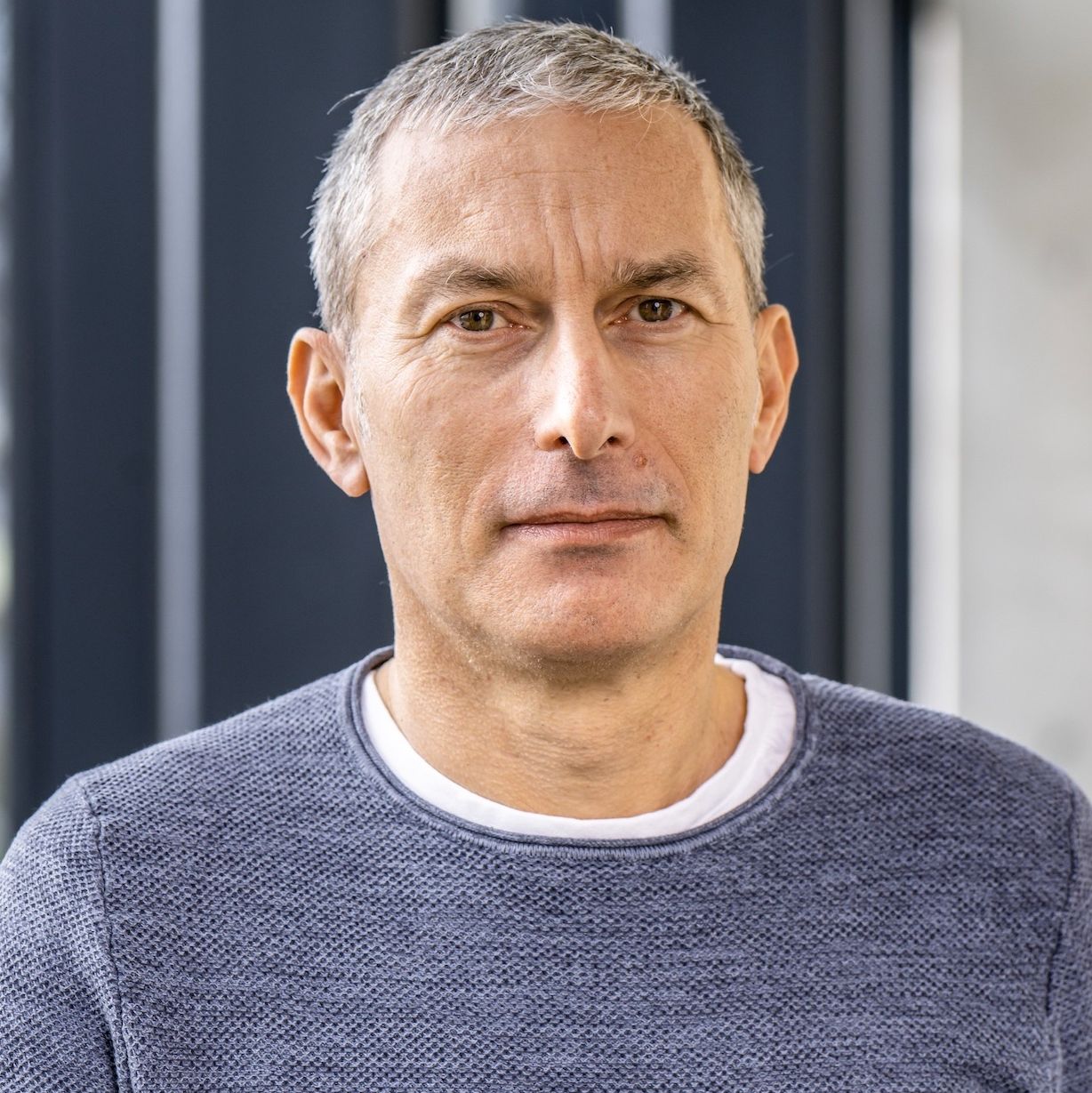
Richard Gloaguen
Richard Gloaguen - HZDR
Helmholtz-Zentrum Dresden-Rossendorf (HZDR) - Exploration and characterization
Short summary of your group's research: We are developing imaging technologies for the characterization of complex material streams and the Earth's surface. Our approach is based on sensor integration and processing using machine learning. Our platforms include drones, robots and conveyor systems.
What infrastructure, programs and tools are used in your group? We have our own imaging sensors, a GPU HPC and develop our own python tools.
What could a participant of the HIDA Trainee Network learn in your group? How could he or she support you in your group? A guest researcher would learn to work with cutting edge technology and process data with the latest developments in computer vision and machine learning.
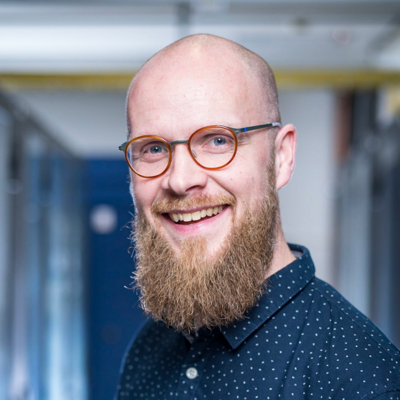
Peter Steinbach
Peter Steinbach - HZDR
Helmholtz-Zentrum Dresden-Rossendorf (HZDR) - Artificial Intelligence
Three-sentence summary of your group's research: We are Helmholtz.AI consultants and work on a wide range of projects. Our core strengths presently are digital twins using machine learning (simulation based inference, surrogate modelling), pattern recognition and object detection in images and other modalities, anomaly detection, uncertainty quantification and prediction robustness. As a plus, we have a strong background in teaching.
What infrastructure, programs and tools are used in your group? We use HPC infrastructure locally and available across Germany. We so far, have concentrated on the use of libraries like pytorch, scikit-learn, scikit-image to which we occasionally contribute.
What could a guest researcher learn in your group? How could he or she support you in your group? In our team, a scientist could learn about how to make a machine learning model more trustworthy (with respect to stability, fairness, uncertainty, robustness and explainability). We can offer guidance on each of the topics we specialize in: digital twins, surrogate modelling, object recognition, anomaly detection, prediction robustness. We can also help to set up a reprucible and scalable machine learning workflow. If you like to learn more, please let me know.
We always welcome support in converting our learnings into open-source tools that the community at large can reuse subsequently.
CISPA - Helmholtz Center for Information Security
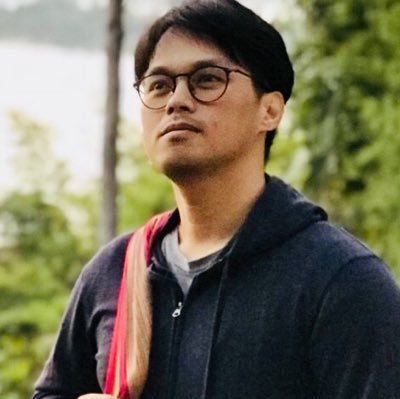
Krikamol Muandet
Krikamol Muandet - CISPA
Helmholtz Center for Information Security (CISPA) - Machine Learning
Personal website
Three-sentence summary of your group's research:
The group’s research aims at understanding the principles that enable autonomous agents to learn from past experience and interact successfully with complex environments, and to use this understanding to design new machine learning algorithms.
What could a guest researcher learn in your group? How could he or she support you in your group?
Conducting and collaborating on world-class research projects that lie at the intersection of machine learning and economics.
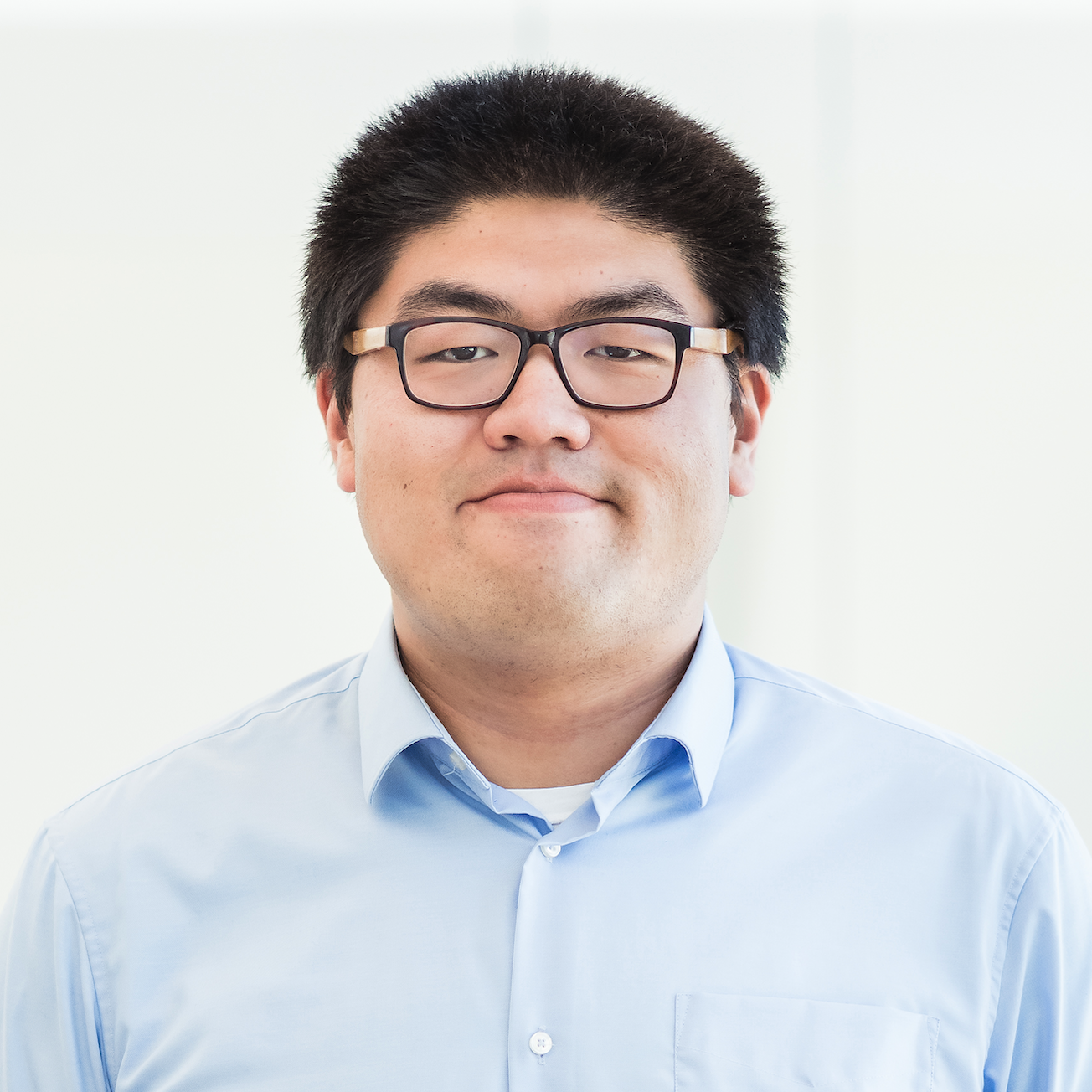
Yang Zhang
Yang Zhang - CISPA
Helmholtz Center for Information Security (CISPA) -
Three-sentence summary of your group's research: Yang Zhang is a faculty member at CISPA Helmholtz Center for Information Security, Germany. His research concentrates on trustworthy machine learning. Moreover, he works on measuring and understanding misinformation and unsafe content like hateful memes on the Internet. Over the years, he has published multiple papers at top venues in computer science, including CCS, NDSS, Oakland, and USENIX Security. His work has received the NDSS 2019 distinguished paper award and the CCS 2022 best paper award runner-up.
What could a guest researcher learn in your group? How could he or she support you in your group? The intern will either lead or assist in a research project conducted in the group. The intern will mainly work on the empirical side of the research, like collecting data, running machine learning models, etc.
What could a guest researcher learn in your group? How could he or she support you in your group? The guest researcher could learn experiences from our research domains and learn each other.
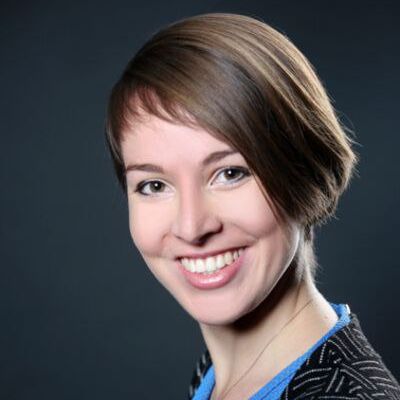
Franziska Boenisch
Franziska Boenisch - CISPA
CISPA - Helmholtz Center for Information Security - Machine Learning Research: Group on Secure, Private, Robust, INterpretable, and Trustworthy Machine Learning
Three-sentence summary of your group's research: Our group, the SprintML lab focuses their research on Secure, Private, Robust, INterpretable, and Trustworthy Machine Learning. We make sure that data processing through machine learning models respects the privacy of their training data, and that after training, the model makes robust, secure, and interpretable predictions.
What infrastructure, programs and tools are used in your group? We rely on high performance computing infrastructure and GPU clusters from the Helmholtz association. Our software is mainly developed in Python and deployed to the GPU clusters in form of GitHub containers.
What could a guest researcher learn in your group? How could he or she support you in your group? A participant under the Visiting Researcher Grant will learn how to automatically process data and manage information through the lens of trustworthy machine learning. The participant will be given the opportunity to discover the risks to machine learning privacy, robustness, and security that arise from the data itself or the training procedure. They will explore mitigation methods to the most crucial risks, and get the chance to implement those themselves, or expand them to new contexts. Finally, the participant can extend their knowledge on publishing academic results in the area of trustworthy machine learning, and will, in the best case, contribute to a paper submission to a top tier machine learning conference. We welcome all participants with a background in machine learning, and an interest in developing data processing that goes beyond considering only utility of the resulting application.
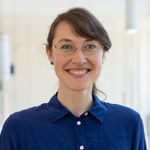
Rebekka Burkholz
Rebekka Burkholz - CISPA
CISPA - Helmholtz Center for Information Security - Relational Machine Learning
Three-sentence summary of your group's research: Our main goal is to reduce the computational costs associated with deep learning based on algorithmic innovations and theoretical insights with practical implications. We particularly enjoy tackling problems that involve graph based data. Most of the applications that motivate us are in the biomedical domain or economics ranging from modeling gene regulatory dynamics, gaining insights into cancer drivers, understanding sex differences, or analyzing systemic risk in international food trade.
What infrastructure, programs and tools are used in your group? As a lot of our projects involve deep learning and neural network sparsification, we make use of GPU clusters.
What could a guest researcher learn in your group? How could he or she support you in your group? We would be happy to collaborate on interesting applications as well as method development in the machine learning domain.
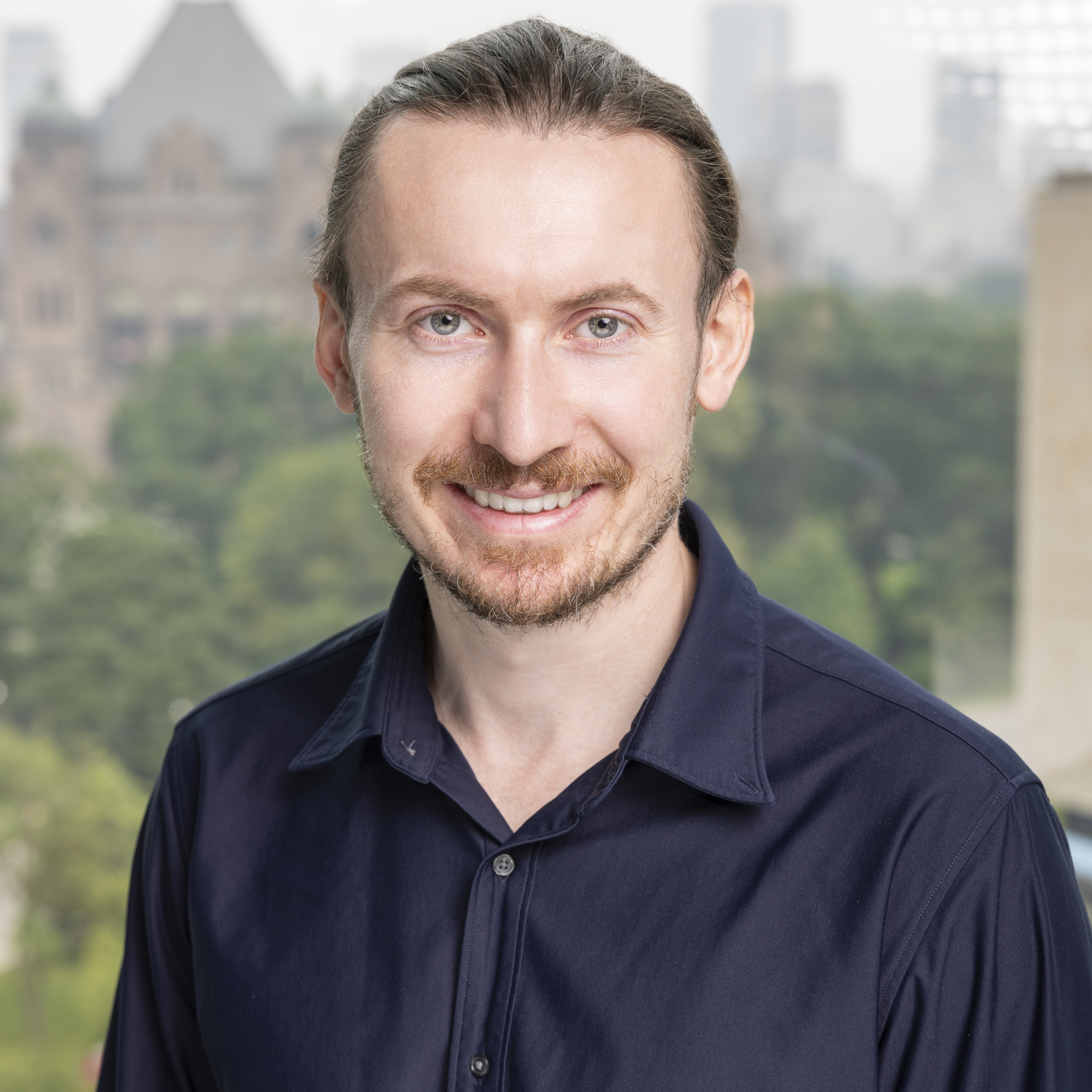
Adam Dziedzic
Adam Dziedzic - CISPA
CISPA - Helmholtz Center for Information Security - SprintML
Three-sentence summary of your group's research: Our research is focused on secure and trustworthy Machine Learning as a Service (MLaaS). We design robust and reliable machine learning methods for training and inference of ML models while preserving data privacy and model confidentiality. We are focused on the state-of-the-art ML methods and models, such as, self-supervised learning, large language and vision models (LLMs), foundational models.
What infrastructure, programs and tools are used in your group? We use large scale GPU clusters and program in the latest ML frameworks.
What could a guest researcher learn in your group? How could he or she support you in your group? As a guest researcher you can learn how to use tools from data privacy and model confidentiality to enable trustworthy machine learning. We also support our guests with theoretical analysis as well as running large scale experiments to publish at the top ML conferences.
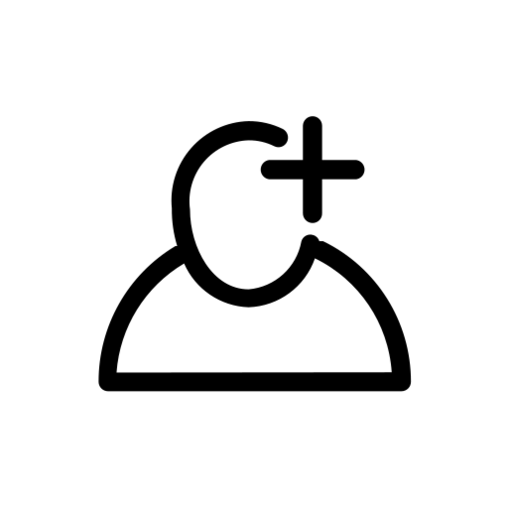
Gastgeber werden
Franziska Boenisch - CISPA
Wenn Sie daran interessiert sind, eine Nachwuchswissenschaftlerin oder einen Nachwuchswissenschaftler aus einem anderen Helmholtz-Zentrum aufzunehmen und Einblick in Ihre Forschung zu gewähren, wenden Sie sich bitte an teresa.weikert@helmholtz.de.
Helmholtz Munich
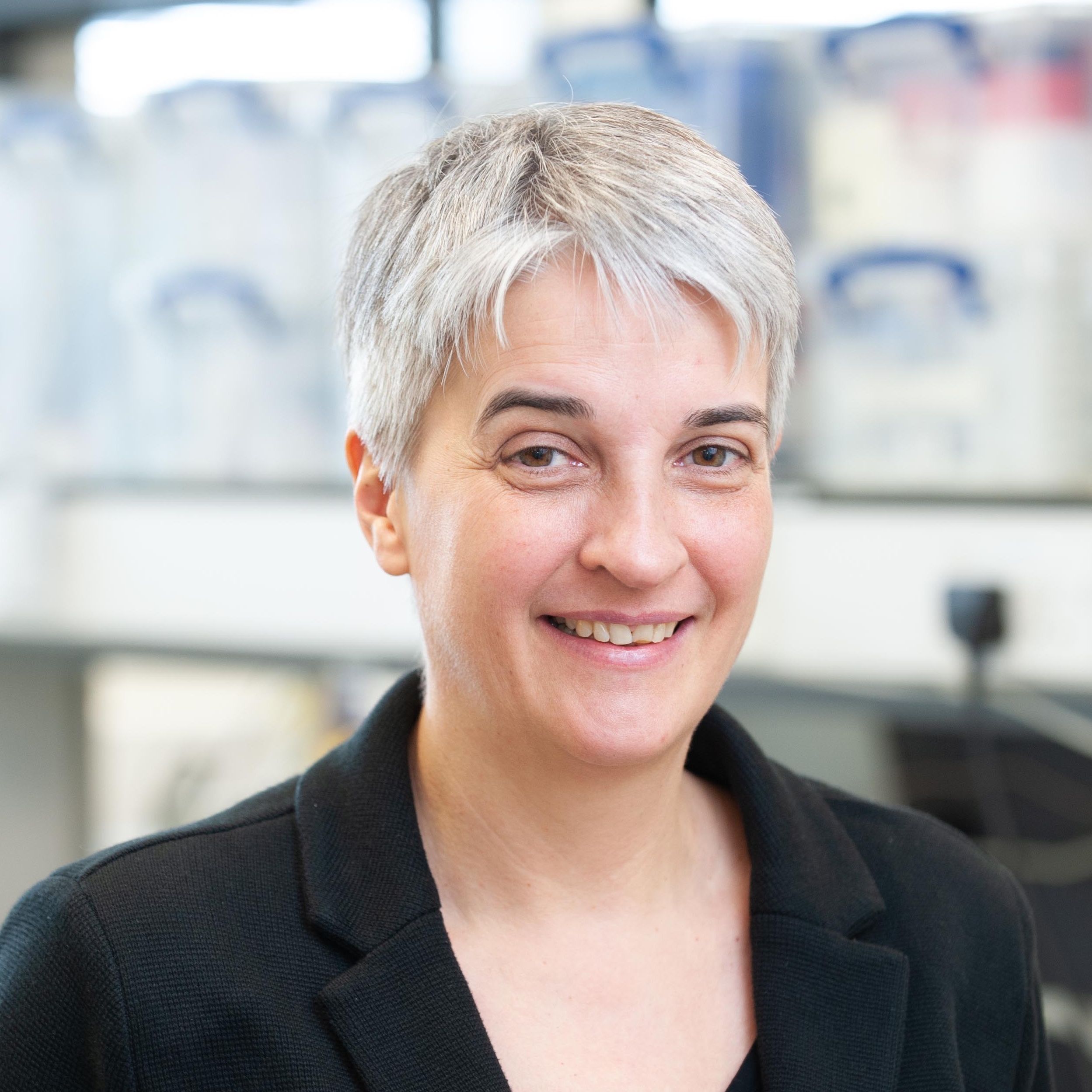
Julia Schnabel
Julia Schnabel - HMGU
Helmholtz Munich (HMGU) - Institute of Machine Learning in Biomedical Imaging - Institute Director and Professor of Computational Imaging and AI in Medicine at TU Munich
Short summary of your group's research: The Institute of Machine Learning in Biomedical Imaging (IML) focuses on research to leverage machine learning for the grand challenges in biomedical imaging in areas of unmet clinical need. Its goal is to fundamentally transform the use of imaging for diagnostics and prognostics. Novel and affordable solutions should empower clinics to make more accurate, fast and reliable decisions for early detection, treatment planning and improved patient outcome.
What infrastructure, programs and tools are used in your group? Novel and affordabel solutions should empower clinics to make more accurate, fast and reliablie decisions for early detection, treatment planning and improved patient outcome.
What could a participant of the HIDA Trainee Network learn in your group? How could he or she support you in your group? Deep learning and machine learning methods for intelligent imaging solutions, from imaging sensor to clinical applications
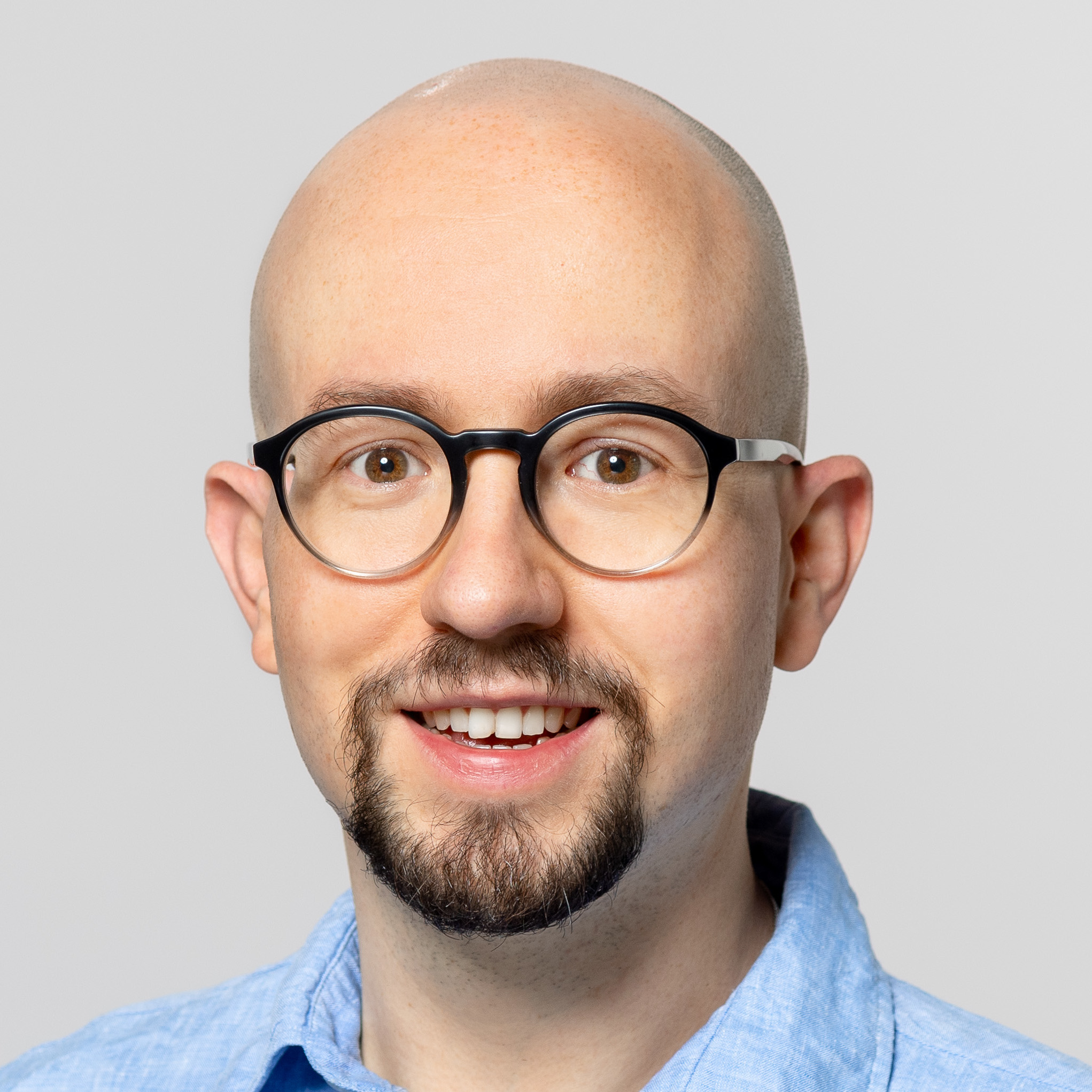
Bastian Rieck
Bastian Rieck - HMGU
Helmholtz Munich (HMGU) - AIDOS Lab, Insitute of AI for Health
Short summary of your group's research: Our primary research interests are situated at the intersection of geometrical deep learning, topological machine learning, and representation learning. We want to make use of geometrical and topological information—also known as manifold learning—to imbue neural networks with more information in their respective tasks, leading to better and more robust outcomes. Following the dictum ‘theory without practice is empty,’ we also develop methods to address current challenges in biomedicine or healthcare applications.
What infrastructure, programs and tools are used in your group? HPC CPU/GPU cluster using Slurm, machine learning frameworks (mostly pytorch) and data analysis tools (scikit-learn, pandas, numpy) based on Python. On the theory side, we are world leaders in topological machine learning, as nascent branch of machine learning.
What could a participant of the HIDA Trainee Network learn in your group? How could he or she support you in your group? Getting to know cutting-edge AI research in geometrical deep learning or topological machine learning, including—but certainly not limited to—graph representation learning. At HMGU, we are sitting on a treasure trove of complex high-dimensional data sets, bearing the promise to substantially advance our understanding of disease-driving mechanisms, for instance. Visitors will benefit from being exposed to a unique combination of theory and practice, with the prospect of working on challenging, impactful applications. We need motivated doctoral researchers who can support our endeavour of developing the next generation of machine learning models to boldly tackle the challenges of today and tomorrow in healthcare!
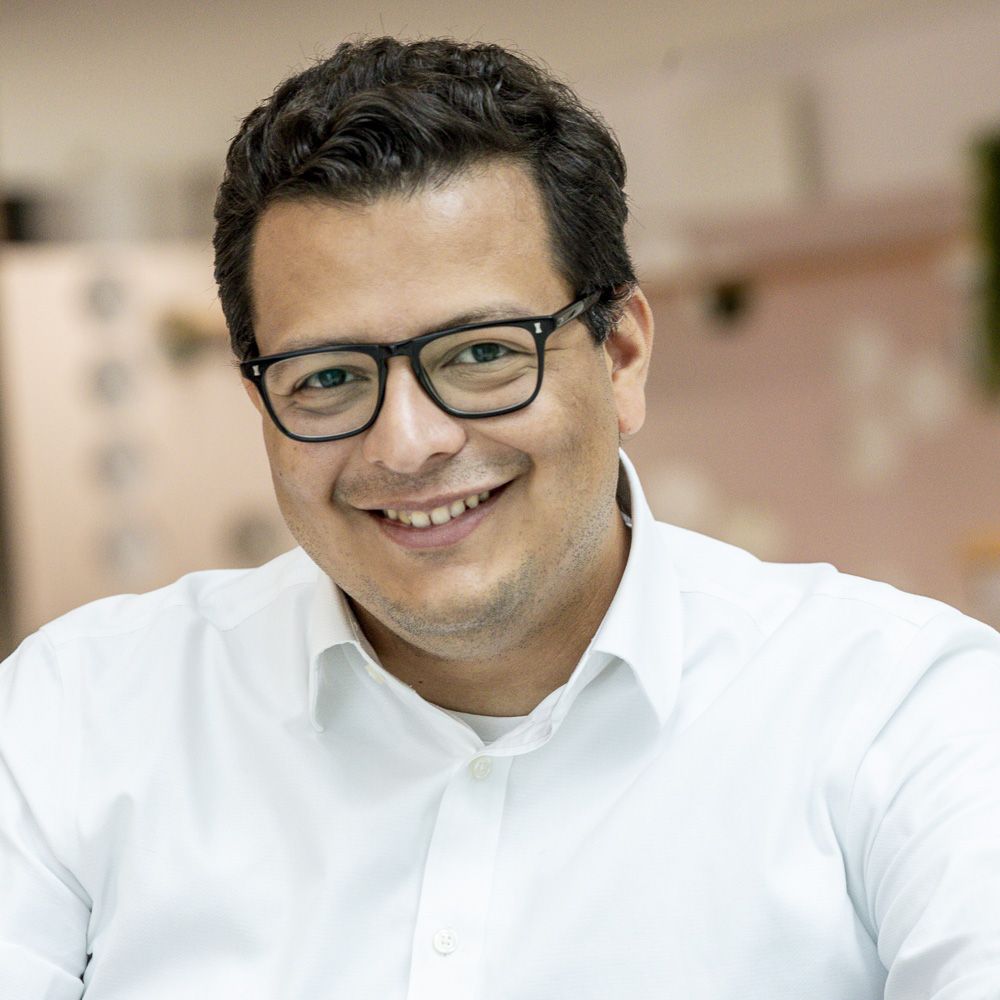
Carlos Talavera-López
Carlos Talavera-López - HMGU
Helmholtz Munich - Computational Immunobiology Lab - Institute of Computational Biology - Computational Health Department
Short summary of your group's research: Our lab specialises in translational single cell biology with focus on infectious diseases. We develop integrative AI approaches to characterise cellular behaviour in health and disease with the aim of identifying diagnostic biomarkers that can be easily deployed in the clinic.
What could a participant of the HIDA Trainee Network learn in your group? How could he or she support you in your group? We are looking for a curious, dedicated scientist interested in learning to apply AI/ML methods to single cell multiome data, and to help us find ways to better communicate biological insights using novel data visualisations. Together, we will better understand the cellular social networks of inflammatory processes, and how these interactions could be potentially be used as diagnostic biomarkers.
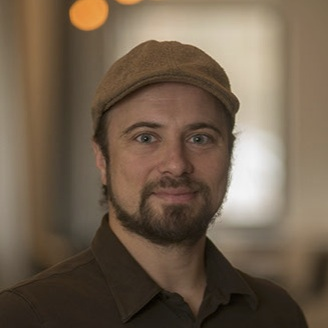
Christian L. Müller
Christian L. Müller - HMGU
Helmholtz Munich (HMGU) – German Research Center for Environmental Health (HMGU) - Biomedical Statistics and Data Science
Three-sentence summary of your group's research: We do research in high-dimensional statistics, (non-)convex optimization, network inference, causal inference, and compositional data analysis with a special interest in microbiome research and microbial ecosystems. We care about sound statistical methodology and good software that is useful for answering broad statistical questions in computational biology and microbial ecology.
What infrastructure, programs and tools are used in your group? We use R, Python, (and MATLAB) for software development, and use GitHub for software deployment and versioning. Group communication is decentralized via Slack and Mattermost. We have (shared) office spaces at LMU Munich (City center) and Helmholtz Munich (in the North of Munich).
What could a guest researcher learn in your group? How could he or she support you in your group? High-dimensional statistics concepts, data analysis workflows for microbiome and microbial data, journal club covering state-of-the-art concepts ranging from experimental high-throughput biology to deep learning.
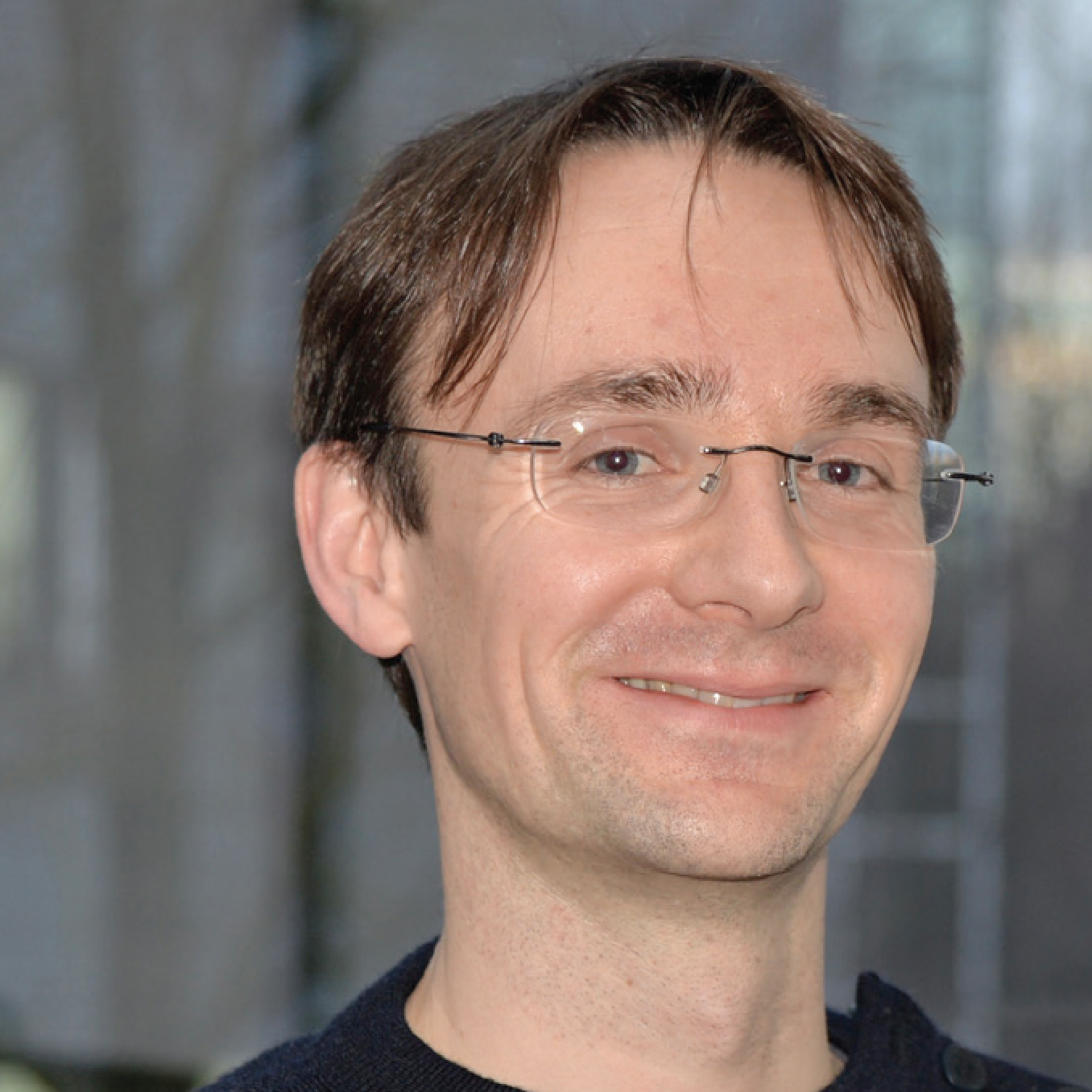
Julien Gagneur
Julien Gagneur - HMGU
Helmholtz Munich (HMGU) – German Research Center for Environmental Health (HMGU) - Gagneur lab / Computational Health Center
Three-sentence summary of your group's research: Our goal is an improved understanding of the genetic basis of gene regulation and its implication in diseases. To this end, we employ AI and statistical modeling of 'omic data and work in close collaboration with experimentalists. We are both based at the TUM and HMGU.
What infrastructure, programs and tools are used in your group? We work with python, ML libraries (pytorch, etc.) and R/Bioconductor.
We have developed multiple software for regulatory genomics and genetic diagnostics including :
- API and repos for trained ML models in genomics Kipoi
- sequence-based model of splicing MMSplice (GitHub)
- Base pair resolution modeling of genome-wide assays BPnet (Article regarding the topic)
- Omics based diagnosis of rare disorders: OUTRIDER, FRASER, DROP: (Protocol regarding the topic)

Niki Kilbertus
Niki Kilbertus - HMGU
Helmholtz Zentrum München – German Research Center for Environmental Health (HMGU) - Reliable Machine Learning Group Leader @ Helmholtz Munich , Informatics Professor @ TUM
Personal website
Three-sentence summary of your group's research: Our main research interests include causality, interpretable dynamical systems modeling, as well as machine learning systems that interact with humans, where we focus on reliable, fair and socially beneficial systems.
What infrastructure, programs and tools are used in your group? We make use of high performance CPU and GPU compute clusters (via Slurm) and regularly use ML frameworks (mostly jax and pytorch) together with the standard Python data analysis and visualization stack (scikit-learn, pandas, numpy, matplotlib, etc). On the theory side, we are interested in (partial) identifiability of causal effects in complex data modalities as well as in the identifiability and estimation of dynamical systems (ODEs, PDEs) from observational data.
What could a guest researcher learn in your group? How could he or she support you in your group? You can learn about the latest and greatest in causal learning and dynamic systems modeling as well as how to train both small and (very) large ML models on one of the largest GPU clusters in Europe. You can apply cutting-edge models to novel bio-medical data to push the boundaries of machine learning powered scientific discovery, drug development, and understanding of health and disease. You can experience working right at the intersection of theory and application with lots of freedom to explore either direction to whatever extent suits you.
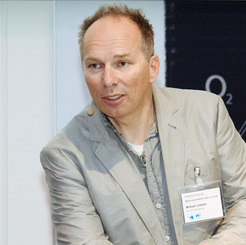
Michael Schloter
Michael Schloter - HMGU
Helmholtz Munich (HMGU) – German Research Center for Environmental Health (HMGU) - Inst. for Comparative Microbiome analysis
Three-sentence summary of your group's research: The human microbiome is a key component for our health. It is strongly influenced by environmental microbiota, which interact with the microbiome of barrier organs like skin or respiratory system. As a consequence, the reduced microbial diversity in the environment, resulting from climate- and global change, strongly impacts human – environment interactions, resulting in an increase in environmental diseases and infections. According to the planetary health concept the prevention of such diseases requires strategies which increase biodiversity in the environment.
We identify key microbiota from the environment, which trigger our health, develop strategies to promote the abundance of those microbiota in urban and indoor environments and analyze consequences for our health.
What infrastructure, programs and tools are used in your group?: We do next generation sequencing of microbiomes using metagneomics approaches from various sources and perform high throughput bioinformatics including KI based tools to reconstruct genomes of microborganisms.
What could a guest researcher learn in your group? How could he or she support you in your group?: In silco genome assembly of microbiota from metagenomics data and functional predications.
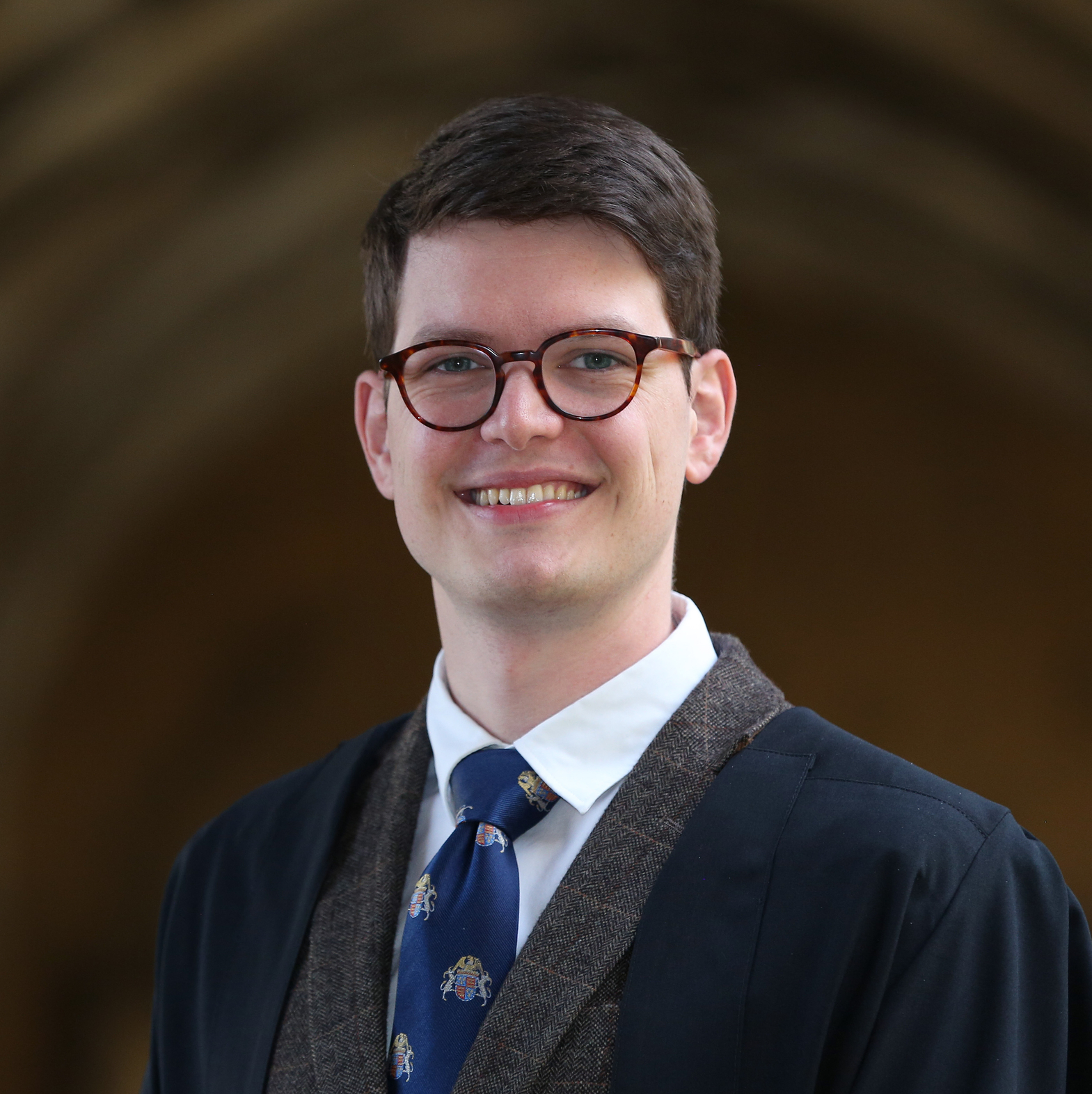
Vincent Fortuin
Vincent Fortuin - HMGU
Helmholtz Munich (HMGU) –
ELLIS lab
Helmholtz AI
Three-sentence summary of your group's research: Our group focuses on the interface between Bayesian inference and deep learning with the goals of improving robustness, data-efficiency, and uncertainty estimation in these modern machine learning approaches. While deep learning often leads to impressive performance in many applications, it can be over-confident in its predictions and require large datasets to train. Especially in scientific applications, where training data is scarce and detailed prior knowledge is available, insights from Bayesian statistics can be used to drastically improve these models. Important research questions include how to effectively specify priors in deep Bayesian models, how to harness unlabeled data to learn re-usable representations, how to transfer knowledge between tasks using meta-learning, and how to guarantee generalization performance using PAC-Bayesian bounds.
What infrastructure, programs and tools are used in your group? We make use of high-performance CPU and GPU compute clusters (via Slurm) and regularly use deep learning frameworks (mostly PyTorch and JAX) together with the standard Python data analysis and visualization stack (scikit-learn, pandas, numpy, matplotlib, etc). On the theory side, we are interested Bayesian model selection, approximate Bayesian inference, and PAC-Bayesian generalization bounds.
What could a guest researcher learn in your group? How could he or she support you in your group? You can learn about the latest and greatest in Bayesian machine learning as well as how to train both small and (very) large deep learning models on one of the largest GPU clusters in Europe. You can apply cutting-edge ML models to a range of scientific data to push the boundaries of ML-powered scientific discovery, drug development, and understanding of health and disease. You can experience working directly at the intersection of theory and application with lots of freedom to explore either direction to suit your interests.
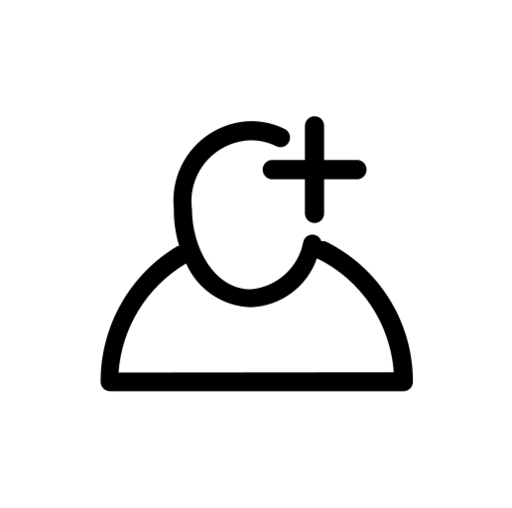
Gastgeber werden
Helmholtz-Gastgeber werden
Wenn Sie daran interessiert sind, eine Nachwuchswissenschaftlerin oder einen Nachwuchswissenschaftler aus einem anderen Helmholtz-Zentrum aufzunehmen und Einblick in Ihre Forschung zu gewähren, wenden Sie sich bitte an teresa.weikert@helmholtz.de.