The thought leaders for environmental solutions: The UFZ is one of the world's leading institutes in environmental research and uses state-of-the-art technology to develop sustainable concepts to protect our natural resources.
With HIDA's mobility programs, data science talents can work on solutions to global environmental problems at the Helmholtz Centre for Environmental Research (UFZ). The transfer of knowledge into practice also plays a central role, for example through innovative monitoring tools such as the Drought and Forest Condition Monitor and the Renewable Energy Monitor.
Apply now!
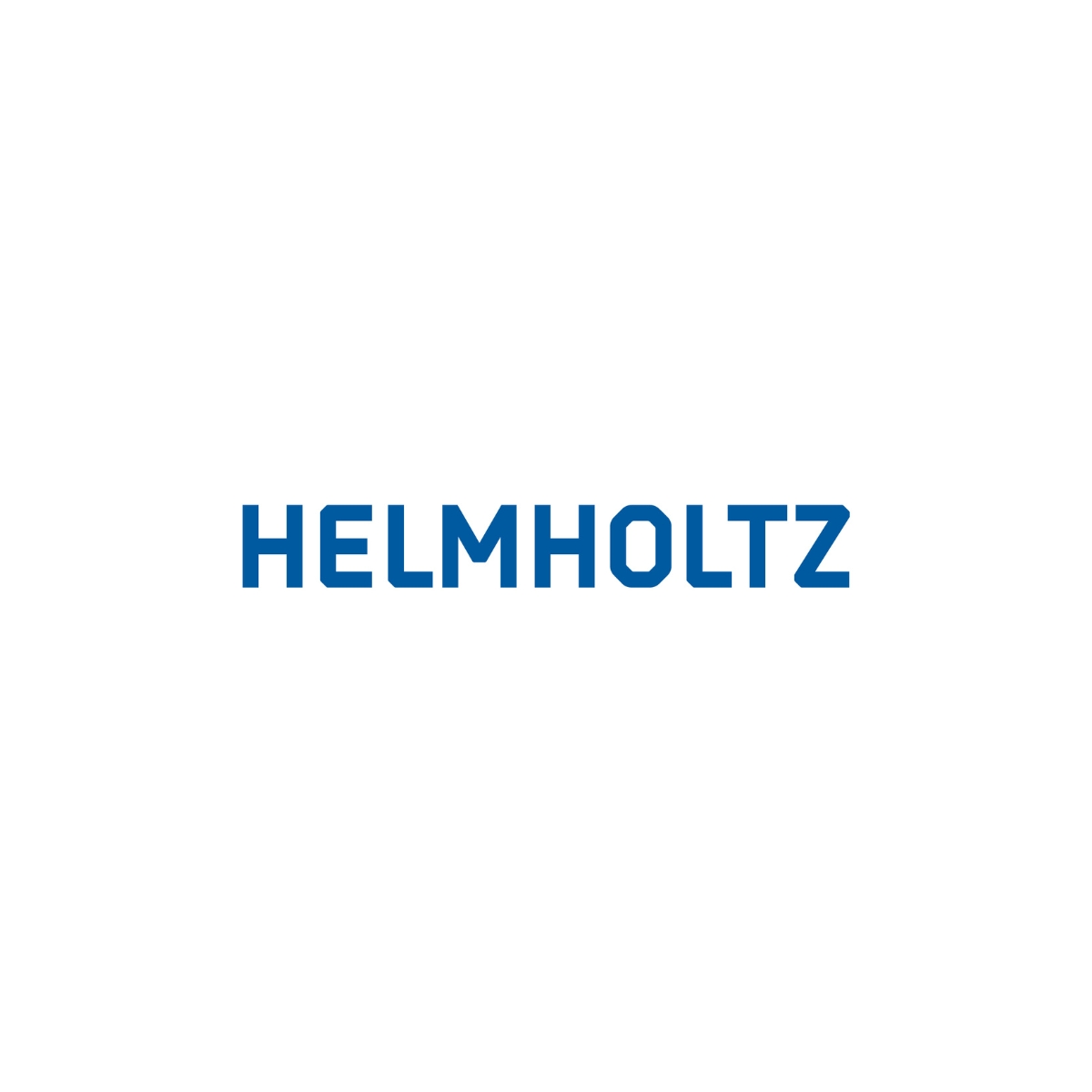
The programs
Get to know the UFZ with HIDA
The Helmholtz Center for Environmental Research is part of the Helmholtz Association.
Data science talents can conduct research at the Center through the following programs.
The UFZ is a leading research institute in the field of environmental research with over 1,100 employees. It investigates the effects of human activities on the environment, particularly in relation to climate change, land use, population growth and energy and food requirements. The researchers work in interdisciplinary teams to develop solutions to global environmental problems.
Main research areas:
- Biodiversity and ecosystem performance
- Quality and quantity of water resources
- Climate protection and climate adaptation research
- Land use conflicts
- Energy transition
- Bioeconomy
- Effects of chemicals on humans and the environment
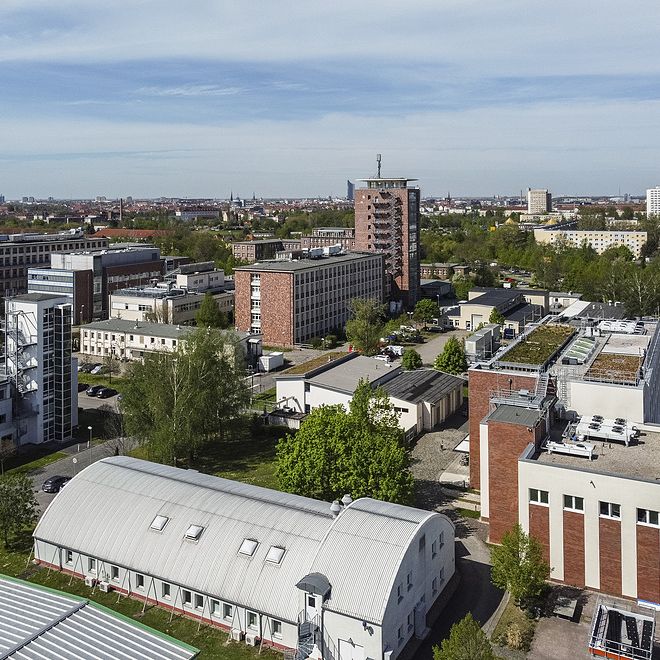
The sites
Sites
UFZ sites
- Leipzig (main location)
- Halle
- Magdeburg
UFZ expertise in the field of Data Science and AI
The UFZ uses data science and artificial intelligence to better understand environmental processes and develop solutions for protecting natural resources. With the help of big data analyses and machine learning, environmental changes, such as the effects of climate change or changes in biodiversity, are precisely modeled. The UFZ uses AI to analyze environmental data and generate sustainable recommendations for action. Particularly in the area of environmental monitoring systems and resource use, AI is used as a key technology to make processes more efficient and sustainable.
- AI-supported environmental modeling
- Analysis of climate data and simulation of climate impacts
- Automated evaluation of environmental monitoring data
- Optimization of land use and resource conservation with machine learning
- Development of sustainable energy concepts using AI
The UFZ is one of the world's leading institutes for environmental research and uses AI and data science to develop solutions for a sustainable future and secure the foundations of life on Earth.
Notes on the application
Notes on the application
Helmholtz hosts
Get to know some potential hosts at different Helmholtz Centers and learn more about their respective data science research by clicking on the cards.
Please note: Please contact your potential supervisor in advance by e-mail to propose and discuss a research project. Only submit your application after this clarification.
If you have any questions, please send an e-mail to: hida@helmholtz.de
Would you like to become a Helmholtz host yourself and are looking for support for your research project? Then please also contact the above e-mail address.
Apply now!
The hosts at the UFZ
Get to know some potential hosts at the Helmholtz Center for Environmental Research and learn more about their respective data science research.
Before contacting the potential hosts, please read the application instructions.
Please note: The listed hosts represent only a selection of possible supervisors.
You are also welcome to independently contact other potential hosts at the center and coordinate your participation in the HIDA Mobility Program directly with them.
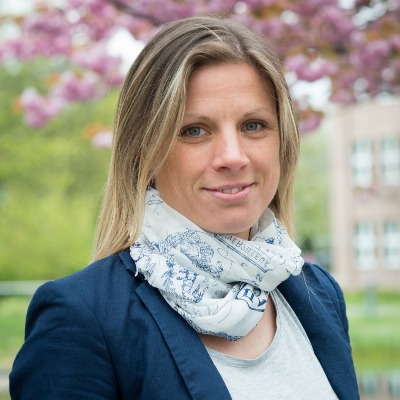
Jana Schor
Microbial Data Science
Contacts
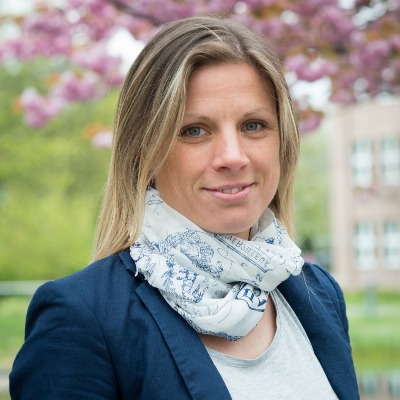
Short summary of your group's research: The new research group Data Science under the supervision of Dr. Jana Schor focuses on Data Science methods, like Machine Learning and Data Integration, to boost the extraction of knowledge from human and environmental health Big Data sets. We apply and develop respective computational methods on public and in-house data sets, mainly in the field of environmental and health research, to generate novel hypotheses, and make predictions on a large scale. To increase the credibility of our AI approaches we introduce explainability and methods for the quantification of uncertainty into our applications. We further attach great importance on the principles of reproducible research.
What infrastructure, programs and tools are used in your group? High performance computing infrastructure at the UFZ and storage as well as data integration capabilities of the European Open Science Cloud; Software is developed in R, and Python, and is provided in Singularity containers and/or in public gitlab repositories at the HZDR; for training our deep learning models or other computationally expensive calculations we use the GPUs that are integrated into our servers and the HPC system.
What could a participant of the HIDA Trainee Network learn in your group? How could he or she support you in your group? A participant of the HIDA Trainee Network will learn to organize his/her data analysis or software development projects according to clear data management plans and with SOPs that allow shared and joint code development, respective documentation, and the reproducibility of research results. We offer to share our expertise in the application of machine learning approaches and respective data pre-processing strategies. Further, we are experienced in story telling with data and offer to teach respective principles to the participants. We welcome participants with a background in machine learning who are interested in the applications of their methods to our research questions, e.g. in the field of predictive toxicology. We also invite researchers with a chemistry, (eco-)toxicological or biological background who might inspire us with interesting research questions and who are willing to learn helpful data science strategies.
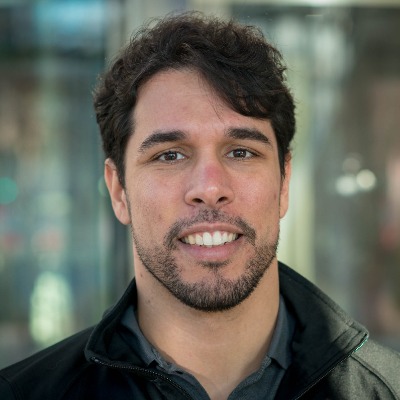
Ulisses Nunes da Rocha
Microbial Data Science
Contacts
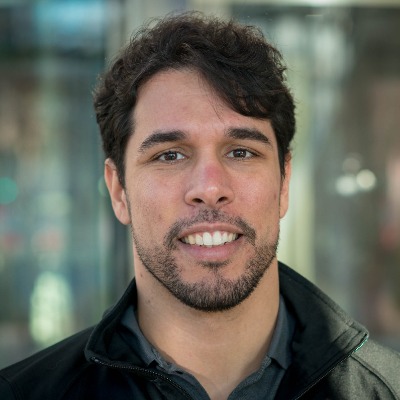
Short summary of your group's research: Our group strives to assess environmental health of terrestrials and man made environments by predicting how resilient/stable microbial communities are to disturbances. A special emphasis is put on the development of concepts and theories to scale microbial interactions to the real diversity found in nature. The key research topics of the Microbial Data Science group are based on genetic potential of microbial communities, multi-omics integration and predictive biology. Currently these topics cover:
- Use of (in silico) mock microbial communities to test microbial ecology theories;
- From microbial 'Big Data' to novel ecological concepts and theories;
- Predictive analytics in microbiology, microbial ecology and environmental microbiology.
What infrastructure, programs and tools are used in your group? We use the High-Performance Computing (HPC) Cluster EVE as our main computational resource. We develop and use tools to analyze, resolve and interpret multi-omics data, specially metagenomes. Eg.: tools for the recovery of multi-domain genomes (prokaryotes, viruses and eukaryotes) from metagenomic data. As programming languages, we mostly use Python, R, Bash and Perl to handle and process microbial big data.
What could a participant of the HIDA Trainee Network learn in your group? How could he or she support you in your group? A participant from the HIDA Trainee Network will learn how to download, process and interpret metagenomic data using both publicly available datasets as well as in-house generated data. Further, the participant will work with hands on experimental design and predictive analytics using omics data. We welcome participants from a broad range of fields (e.g. microbiology, ecology, computer/data science) that are eager to learn and/or expand their knowledge in computational biology/data science.
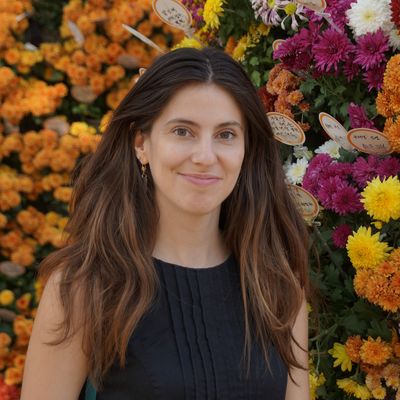
Mariana Madruga de Brito
Environmental Risks & Vulnerability
Contacts
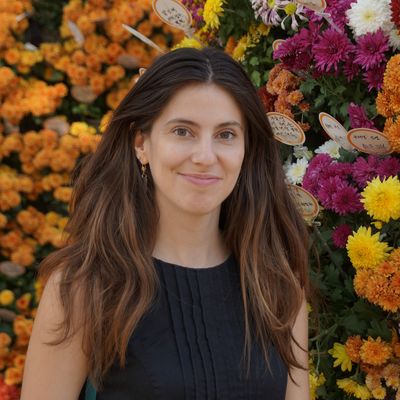
Short summary of your group's research: The research stay will be conducted at the working group Environmental Risks and Extreme events led by Prof. Christian Kuhlicke (https://www.ufz.de/index.php?en = 40374). Dr. Mariana Madruga de Brito will supervise the research. The group has expertise in computational social sciences and digital humanities. We use text as data for natural hazards adaptation, resilience and vulnerability research. We are particularly interested in understanding how these processes affect society and who are prominent stakeholders. Topics of interest include natural language processing (NLP), topic modelling, and unsupervised and supervised learning algorithms. Our text corpora include newspaper articles, tweets, and large sets of peer-reviewed articles.
What infrastructure, programs and tools are used in your group? The participant will have access to high-performance computing infrastructure at the UFZ. As programming languages, we mainly use R and Python.
What could a participant of the HIDA Trainee Network learn in your group? How could he or she support you in your group? As an interdisciplinary research group, we offer many possibilities for a participant of the HIDA Trainee Network and many different applied projects to support. Weekly meetings will be conducted to help monitor progress. The participant can either apply their NLP knowledge to our existing data or shape a new project together with a focus on natural hazards and climate change adaptation research. The final goal is to have a prominent joint publication.
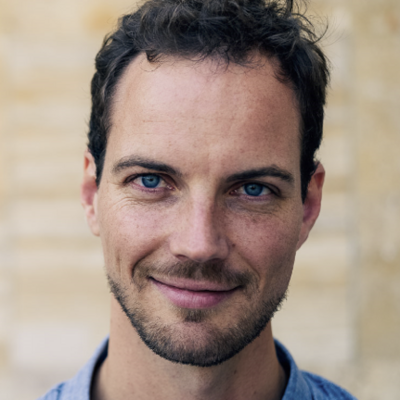
Jakob Zscheischler
Computational Hydrosystems
Contacts
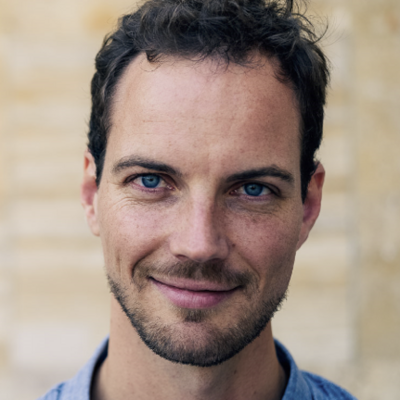
Three-sentence summary of your group's research: We work on better understanding and modelling compound weather and climate events and their impacts. We use statistical and machine learning approaches to analysis climate and impact data to better understand compounding climate drivers of impacts, We further analysie climate model projections to estimate future climate risk.
What infrastructure, programs and tools are used in your group? We analyse large amounts of data, learn new statistical and machine learning approaches to estimate climate risks.
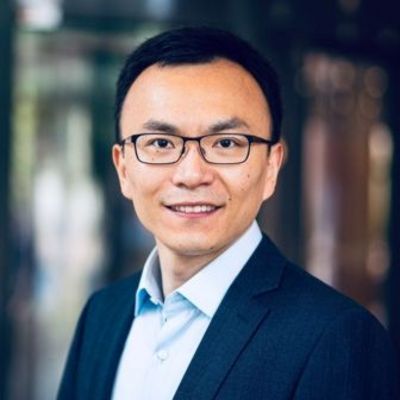
Jian Peng
Hydrology and Remote Sensing
Contacts
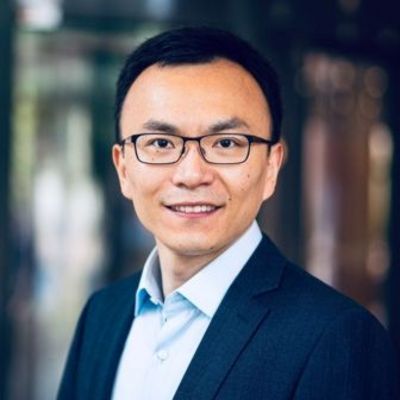
Short summary of your group's research: The Department of Remote Sensing conducts innovative research to advance the understanding of the Earth system via various remote-sensing techniques. It has extensive research experience in quantifying land surface dynamics from multi-source Earth observations across scales. Another focus lies on the investigation of land-atmosphere interaction and climate extremes using novel remote sensing products.
What infrastructure, programs and tools are used in your group? Our team has established data lake to facilitate access to and analyse of datasets from satellite, airborne remote sensing and earth system model outputs. Modelling frameworks including radiative transfer model and land surface model have also been developed in last years. Computational infrastructures including in-house HPC and external cloud computing system can also be used by guest researchers.
What could a participant of the HIDA Trainee Network learn in your group? How could he or she support you in your group? The guest researcher can benefit from our expertise on:
1.Quantification of land surface parameters using multi-source remote sensing observations.
2. Integrate remote sensing data and land surface modelling framework to better quantify water cycle and vegetation dynamics
3. Explore land-atmosphere feedbacks and hydro-climatic extremes through novel use of remote sensing datasets (e.g., detection of extreme events, impacts of extremes on water cycle and ecosystems).
The guest researcher are encouraged to contribute to the above mentioned research topics using machine learning approaches.
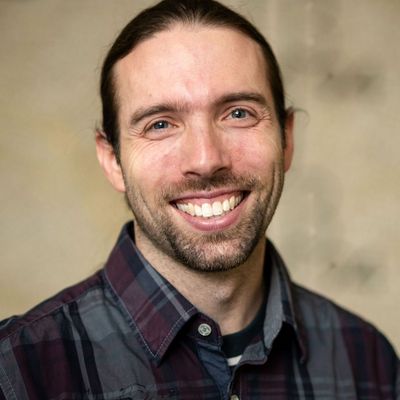
Oliver Lechtenfeld
Environmental Analytical Chemistry
Contacts
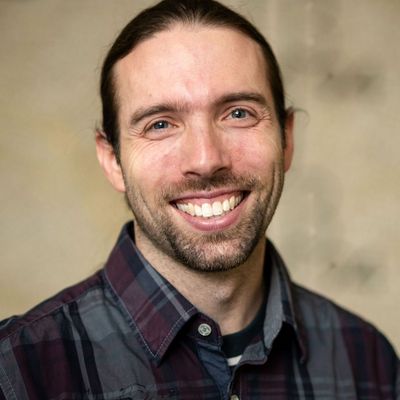
Short Summary of your Groups research: The research group BioGeoOmics integrates state-of-the art instrumental and methodological approaches to study dynamic interactions of molecules in the environment. These molecular interactions include natural processes (e.g. metabolism, organic matter diagenesis) as well as anthropogenic interferences (interaction of biological systems with chemicals). In addition to analytical method development we also develop chemoinformatics and machine learning aided data evaluation techniques.
What infrastructure, programs and tools are used in your group?At the core of the the analytical toolkit of the BioGeoOmics-group, we use ultra-high resolution mass spectrometry (FT-ICR MS) and mass spectrometric imaging (MSI). We devolp methods for non-target metabolomics and biogeochemistry using stable isotopes to study chemical and biological transformations of organic molecules, their spatial localization in tissues, and to identify metabolites and unknown chemicals and transformation products in complex mixtures.
Our group develops (automated and interactive) data processing pipelines using R, the ETL platform KNIME, and PostgreSQL DBMS. We apply multivariate statistical tools and machine learning models to extract knowledge from the zentillion data points generated from ultra-high resolution mass spectrometry and large evvironmental data sets.
What could a participant of the HIDA Trainee Network learn in your group? How could he or she support you in your group? Since our group has a focus on non-targted mass spectrometry, our guest researchers will be able to learn how to utilize and interpret non-targeted data, how to use statistical tools to evaluate the data quality, and how to derive confidence limits inorder to make sound statements about the data. Besides instrumental training on state-of-the art FT-ICR MS systems, we can provide helpful insights about data management and quality control.
Future guest researchers are welcome to provide input on fast and efficient visualization strategies for large amount of data points, on (technical legal) aspects) of software publication, and on continuous integration/deployment.
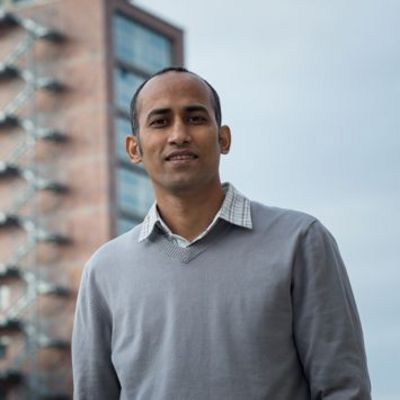
Rohini Kumar
Computational Hydrosystems
Contacts
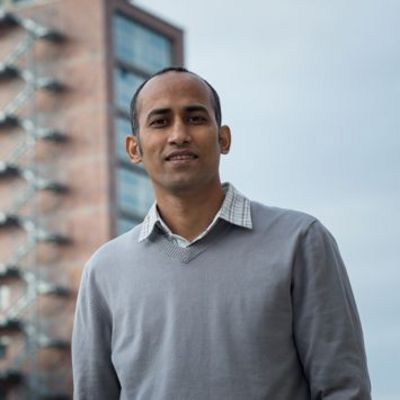
Three-sentence summary of your group's research: Our group works on improving our understanding of hydroclimatic drivers, anthropogenic pressures, and responses to the fate of water and nutrient cycles in the terrestrial system. We use data analytics and mechanistic modeling approaches to analyze and disentangle the varying role of climate, landscapes, and socioeconomic drivers shaping the hydrological response of the system. Our analysis encompasses a range of temporal scales and spatial domains varying from hourly to annual timescale in small headwater catchments to big continental-scale river basins, and our investigations cover challenging freshwater resource problems with an emphasis on unraveling hydroclimatic extremes (e.g., floods, droughts, heatwaves) under historical, contemporary and future climate conditions.
What infrastructure, programs and tools are used in your group? We use both the local PC and High-Performance Computing (HPC) systems tailored to specific needs. We use our in-house developed multi-scale hydrologic modeling system (www.ufz.de/mhm) and water quality models for large-scale simulations.
What could a guest researcher learn in your group? How could he or she support you in your group? Given the wide diversity of our work, we offer learning processes on the building blocks of mechanistic models, working with large-sample and large-scale databases. We welcome and invite researchers from different disciplines (e.g. computational hydrology, water quality, agronomy, and soil sciences) to work with us on synthesizing and documenting processes, patterns, and trends of water and nutrient flow under historical and future climate and socioeconomic conditions.
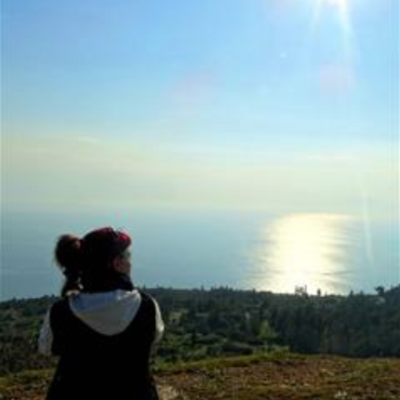
Elina Takola
Computational Landscape Ecology
Contacts
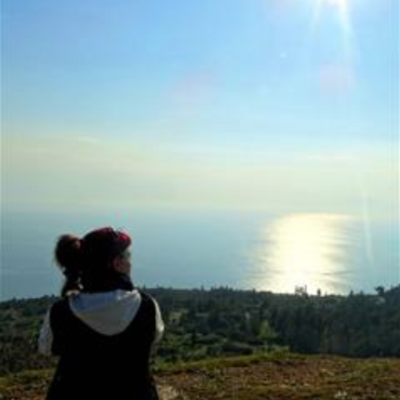
Three-sentence summary of your group's research: My research interests are in the field of landscape ecology, with a focus on animal ecology, biodiversity monitoring, data syntheses and meta-analyses.
I am a supporter of open science and FAIR principles. I mostly R and sometimes Python to analyze published studies and big datasets.
What could a guest researcher learn in your group? How could he or she support you in your group? Guests can participate in data synthesis projects and meta-analyses. We summarize large amounts of literature in a comprehensive way, in order to provide a complete picture of evidence to scientists, stakeholders and decision-makers.
We at the UFZ