Algorithms to prevent mudslides
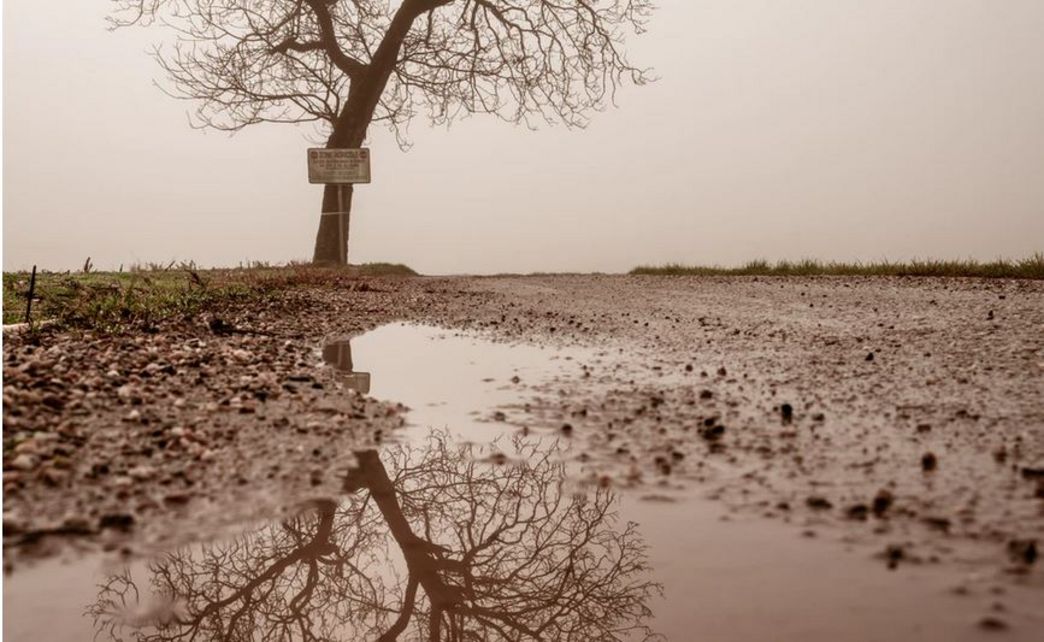
In order to be better prepared for extreme weather events in the future, scientists at the Helmholtz Centre for Environmental Research are trying to map the laws of nature using algorithms.
"I've always been curious about the outside world. To its nature, which is so very diverse in different corners of the world. Since my youth, I have loved being outside. Kayaking, mountain biking, hiking. The nature of the earth has so much to offer, it is a madness that we endanger it. I wanted to do something about that in my profession. I decided to study geography because this subject is about the environment and nature from very different perspectives."
How we get a better understanding of water
"Do you know hydrology? That was my main focus in my master's degree at the University of Freiburg. The water sciences deal with questions like: When does flooding occur? How can changes in groundwater be predicted? How will the water balance on earth change due to climate change? What does this mean for agriculture, vegetation and land use? Hydrologists' research provides key data to better understand the consequences of climate change.
Up to now, hydrology has mainly worked with physical models. We feed them with observational data and our knowledge of the relationships. For example: How much water seeps away and evaporates depending on rainfall, solar radiation, soil conditions, plant growth? The problem: The models have gaps because in many places we have not yet understood exactly how the different parameters are related. For example, how much CO2 from the soil is absorbed by the atmosphere? We know that this exchange depends on microbacterial processes in the soil, on parameters such as vegetation or temperature. But we know little about how exactly this happens. We can now fill these gaps with algorithmic Big Data models."
Why data and algorithms can help us in the fight against climate change
"In my master degree, I understood: Statistics and simulation can be the key to solving many climate problems. If we succeed in mapping laws of nature in algorithms, we can use them to build models that allow us to predict the future. Two developments are making this possible now: Big Data and the enormous advances in artificial intelligence.
Today we can collect and store more data than ever before. New sensors are collecting new data on hydrology everywhere in the environment, from groundwater levels to vegetation, soil moisture and land use. High-performance computers store this information in huge databases.
On the other hand, with the help of artificial intelligence and machine learning, we can finally make effective use of these huge amounts of data. The new big thing is algorithmic big data models. The trick: If we train algorithms with millions of data, they learn patterns. You could also say they learn relationships. These patterns enable us to predict how things will develop - even if the data is incomplete.
For example, data from reservoirs are often missing when we want to use a physical model to predict how the water level of a river will develop. This is because some operators do not provide this data. An algorithm-based model can fill this gap by calculating probabilities from existing data. How much water flows into the reservoir, how much comes out, how much has it rained?
The more data and knowledge from different disciplines we feed into such models, the better are the results. In the "Digital Earth" programme, researchers from several Helmholtz Centres and Research Fields are currently working at full speed on this. For example, to be able to forecast flood risks in the climate crisis more accurately and to better understand the erratic long-term effects on the land and coastal system. This requires a great deal of data management. And that's the crux of the matter right now."
What I want to do to save the world
"I want us to use data much more efficiently than before. To do this, we need to standardize formats and methods and define common interfaces. This is the only way to bring together data from completely different fields and make it usable for research.
We have to find ways of evaluating all the measurement data automatically. And to make the results foolproof. Because there are hidden errors in a lot of data. For example, when an earthworm crawls past a soil sensor, the signal changes. If leaves stick to a precipitation collector, it delivers incorrect data. We can't find the errors in millions of pieces of data everywhere by ourselves. We need artificial intelligence, a machine-learning model.“
Author: Anja Dilk