Machine learning to improve cities
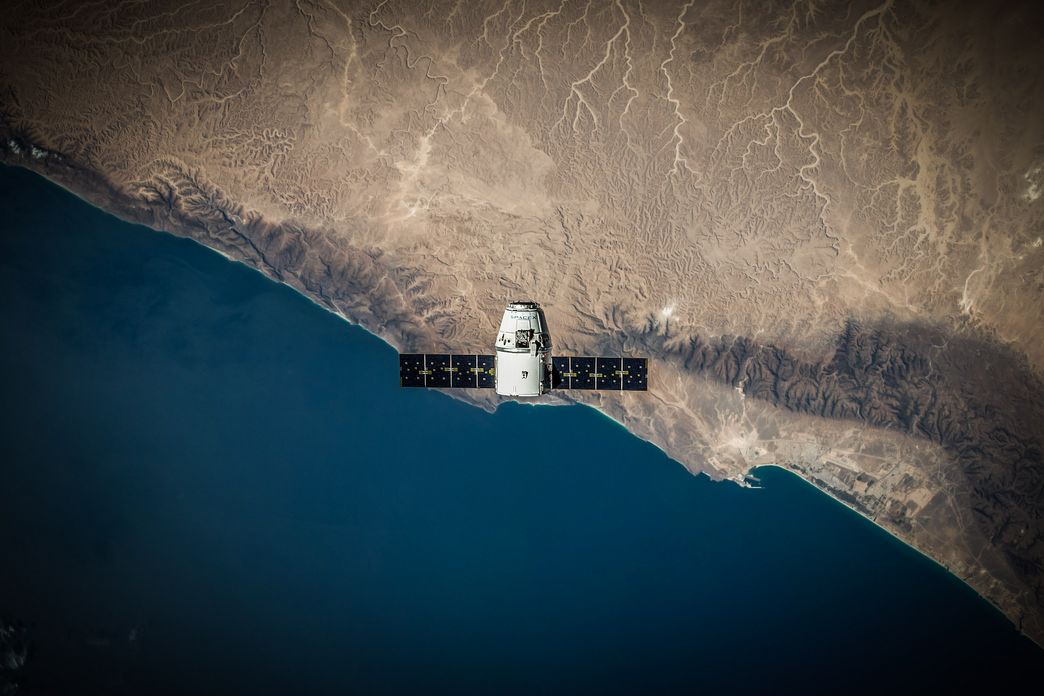
Armed with social media data and satellite images, Dr. Xiaoxiang Zhu and her team at the German Aerospace Center are creating global maps of urban areas that can be used to manage urbanization in the era of climate change.
“When I was a little child, I saw a picture of the Earth taken from space and I found the image so thrilling,” says Dr. Xiaoxiang Zhu, Head of the Earth Observation Data Science Department at the German Aerospace Center, also known as the DLR. “This sparked my interest in space and since then I’ve been fascinated by it.”
In addition to her role at the DLR, Zhu is a professor for Signal Processing in Earth Observation at the Technical University of Munich (TUM) since 2015. Born in 1984 in Hunan, China, she decided to further pursue her interest in space and completed a Bachelor’s degree in aerospace engineering in China. In 2008, Zhu moved to Munich to get a Masters and Doctorate in engineering at TUM. For someone who was interested in both space and mathematics from a young age, it’s a wonderful coincidence that she now gets to work with data and images from space every day.
Alongside her team at the DLR in Munich, Zhu conducts research on using signal processing and machine learning methods to extract geo-information from petabytes of satellite data that are freely available. One petabyte is equivalent to a thousand trillion (or 1,000,000,000,000,000) bytes of digital information. “In the past couple of years, the Earth observation field has changed dramatically. Today, up to a hundred petabytes of satellite data from different sensors are freely available,” she explains.
Using social media data and satellite imagery to tackle urbanization and climate change
In particular, Zhu and her team focus on how large amounts of geo-information can be extracted from satellite data and used to map urban areas around the world. Ultimately, this would then be used to create a global model of cities to overcome challenges related to the rapid rise of global urbanization. In 2050, two-third of the world’s population is projected to live in cities. While urban areas are expected to become opportunities for economic growth, they will also face a number of challenges, especially in relation to climate change. For instance, by 2050, summers in Berlin could see temperatures increase as high as those in the Australian capital city of Canberra.
To respond to these problems and ensure the sustainability of cities, we need more comprehensive data on a global scale so that urban climatologists, who examine the relationships between urban areas and the atmosphere, can prepare for the future. That’s where theSo2Sat project, led by Zhu, comes into play. With the aim of closing gaps of knowledge and to better understand how urban areas develop over time, the So2Sat project is about developing machine learning algorithms that can effectively combine large amounts of image and text files from social networks with petabytes of remote sensing data from various German and European Earth observation satellites. Hence the project name, So(cial media data)2Sat(ellite data).
Currently, several satellites from the European Space Agency (the so-called Sentinel satellites) measure the Earth’s surface. However, the measurements are not in a form that is useful to urban climatologists. Fusing huge amounts of social media data with Earth observation satellite data would enable the creation of high-res 3D and 4D maps of cities across the globe that could provide more accurate information about different kinds of buildings/urban infrastructures as well as population densities. “We will make this data accessible to everybody and we hope it will lead to a better understanding of the global urbanization process,” said Zhuin a 2019 TEDx Talk. “We want to provide this data to stakeholders, like the UN, to help them make strategic decisions.”
“I want to encourage young people who are interested in machine learning to focus on the big challenges that human beings are facing now, like climate change and urbanization.”
Dr. Xiaoxiang Zhu
Providing open access to data to enable a larger global impact
For a long time, there wasn’t a universal or accessible approach to describe the physical nature of cities and to compare them across geographical regions for urban climatologists. To address this issue, scientists developed the Local Climate Zone classification scheme. It divides landscapes into 17 zones that are differentiated by the physical properties of land, like surface structure (e.g. height, density and arrangement of buildings and vegetation) and surface cover (e.g. percentage of greenery, surfaces that allow water to seep through versus impervious surfaces like concrete). “This classification can help people identify where the heat islands are which is very important in investigating the effects of climate change on urban areas,” Zhu tells us.
Zhu, who has received many scientific awards such as the Leopoldina Early Career Award and PRACE Ada Lovelace Award in 2018, believes the next generation of data scientists can make a big difference if they apply their skills in the realm of Earth observation. “I want to encourage young people who are interested in machine learning to focus on the big challenges that human beings are facing now, like climate change and urbanization,” she says.
For the HIDA Datathon for Grand Challenges on Climate Change, Zhu and her team at DLR are asking data scientists to develop machine learning models that can automatically usepublicly available satellite data from the Sentinel missions to create consistent Local Climate Zone maps. The dataset, dubbed So2Sat LCZ42, consists of three analysis-ready parts: training, validation and test data. While there are algorithms that calculate these maps using this dataset, they can still be improved, especially by further developing the deep learning networks that are applied to analyzing visual imagery called the Convolutional Neural Networks (CNN).
By developing machine learning models that can create more reliable Local Climate Zone maps, data scientists can help urban climatologists learn more about steps to take in order to combat extreme temperatures as a result of climate change. This information can then be relayed to city planners who might decide to plant trees from the Mediterranean that withstand heat, or plant greenery that soaks up water and provides shade to areas with a lot of concrete and asphalt. Ultimately, changes made on the local scale will affect the overall climate on a larger scale.
Author: Charmaine Li